Camera Pose Matters: Improving Depth Prediction by Mitigating Pose Distribution Bias
2021 IEEE/CVF CONFERENCE ON COMPUTER VISION AND PATTERN RECOGNITION, CVPR 2021(2021)
摘要
Monocular depth predictors are typically trained on large-scale training sets which are naturally biased w.r.t the distribution of camera poses. As a result, trained predictors fail to make reliable depth predictions for testing examples captured under uncommon camera poses. To address this issue, we propose two novel techniques that exploit the camera pose during training and prediction. First, we introduce a simple perspective-aware data augmentation that synthesizes new training examples with more diverse views by perturbing the existing ones in a geometrically consistent manner. Second, we propose a conditional model that exploits the per-image camera pose as prior knowledge by encoding it as a part of the input. We show that jointly applying the two methods improves depth prediction on images captured under uncommon and even never-before-seen camera poses. We show that our methods improve performance when applied to a range of different predictor architectures. Lastly, we show that explicitly encoding the camera pose distribution improves the generalization performance of a synthetically trained depth predictor when evaluated on real images.
更多查看译文
关键词
geometrically consistent manner,per-image camera,predictor architectures,synthetically trained depth predictor,camera pose matters,improving depth prediction,mitigating pose distribution bias,monocular depth predictors,large-scale training sets,trained predictors,reliable depth predictions,testing examples,uncommon camera,simple perspective-aware data augmentation,synthesizes new training examples,diverse views
AI 理解论文
溯源树
样例
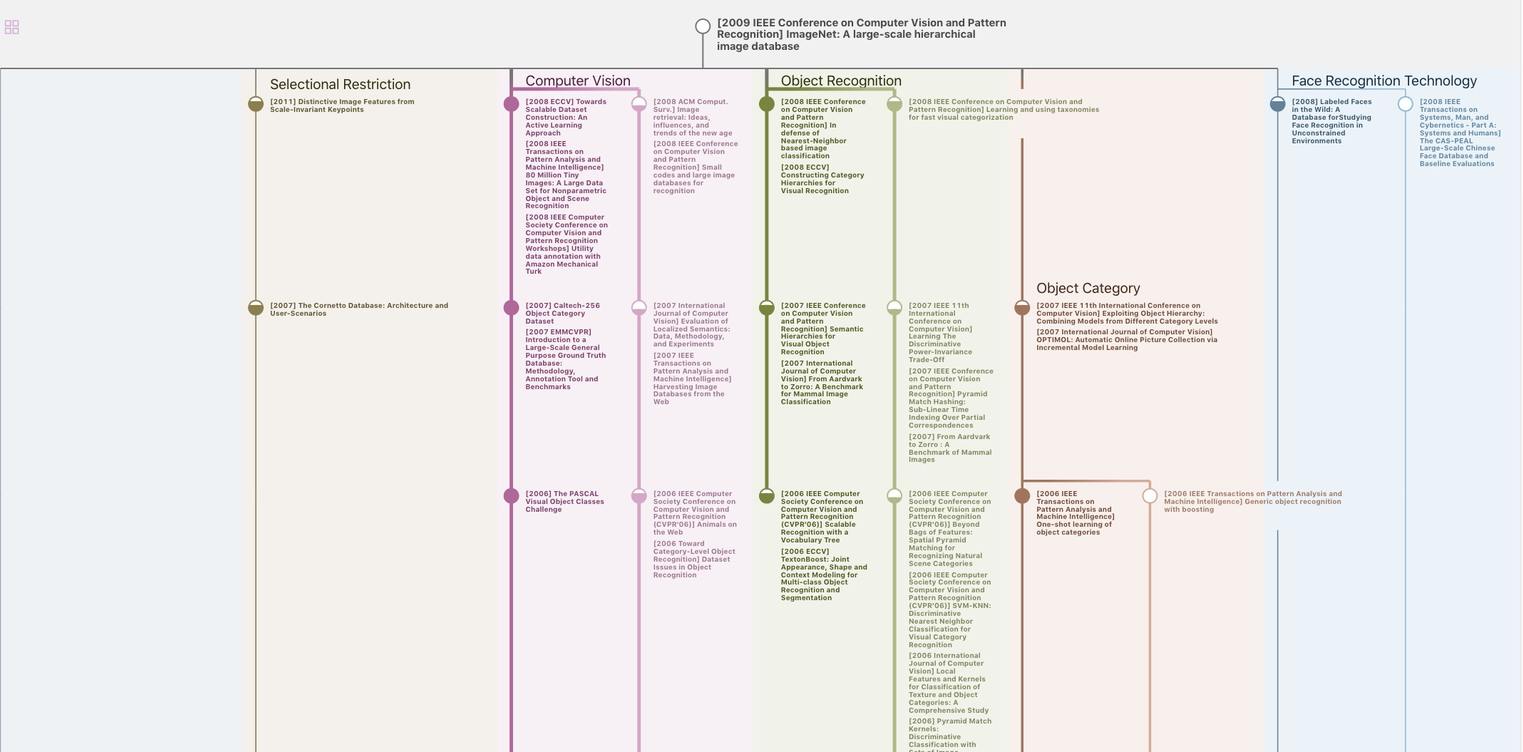
生成溯源树,研究论文发展脉络
Chat Paper
正在生成论文摘要