Towards Safe Control Of Continuum Manipulator Using Shielded Multiagent Reinforcement Learning
IEEE ROBOTICS AND AUTOMATION LETTERS(2021)
摘要
Continuum robotic manipulators are increasingly adopted in minimal invasive surgery. However, their nonlinear behavior is challenging to model accurately, especially when subject to external interaction, potentially leading to poor control performance. In this letter, we investigate the feasibility of adopting a model-free multiagent reinforcement learning (RL), namely multiagent deep Q network (MADQN), to control a 2-degree of freedom (DoF) cable-driven continuum surgical manipulator. The control of the robot is formulated as a one DoF, one agent problem in the MADQN framework to improve the learning efficiency. Combined with a shielding scheme that enables dynamic variation of the action set boundary, MADQN leads to efficient and importantly safer control of the robot. Shielded MADQN enabled the robot to perform point and trajectory tracking with submillimeter root mean square errors under external loads, soft obstacles, and rigid collision, which are common interaction scenarios encountered by surgical manipulators. The controller was further proven to be effective in a miniature continuum robot with high structural nonlinearitiy, achieving trajectory tracking with submillimeter accuracy under external payload.
更多查看译文
关键词
Reinforcement learning, modeling, control, and learning for soft robots, model learning for control, robust/adaptive control, medical robots and systems
AI 理解论文
溯源树
样例
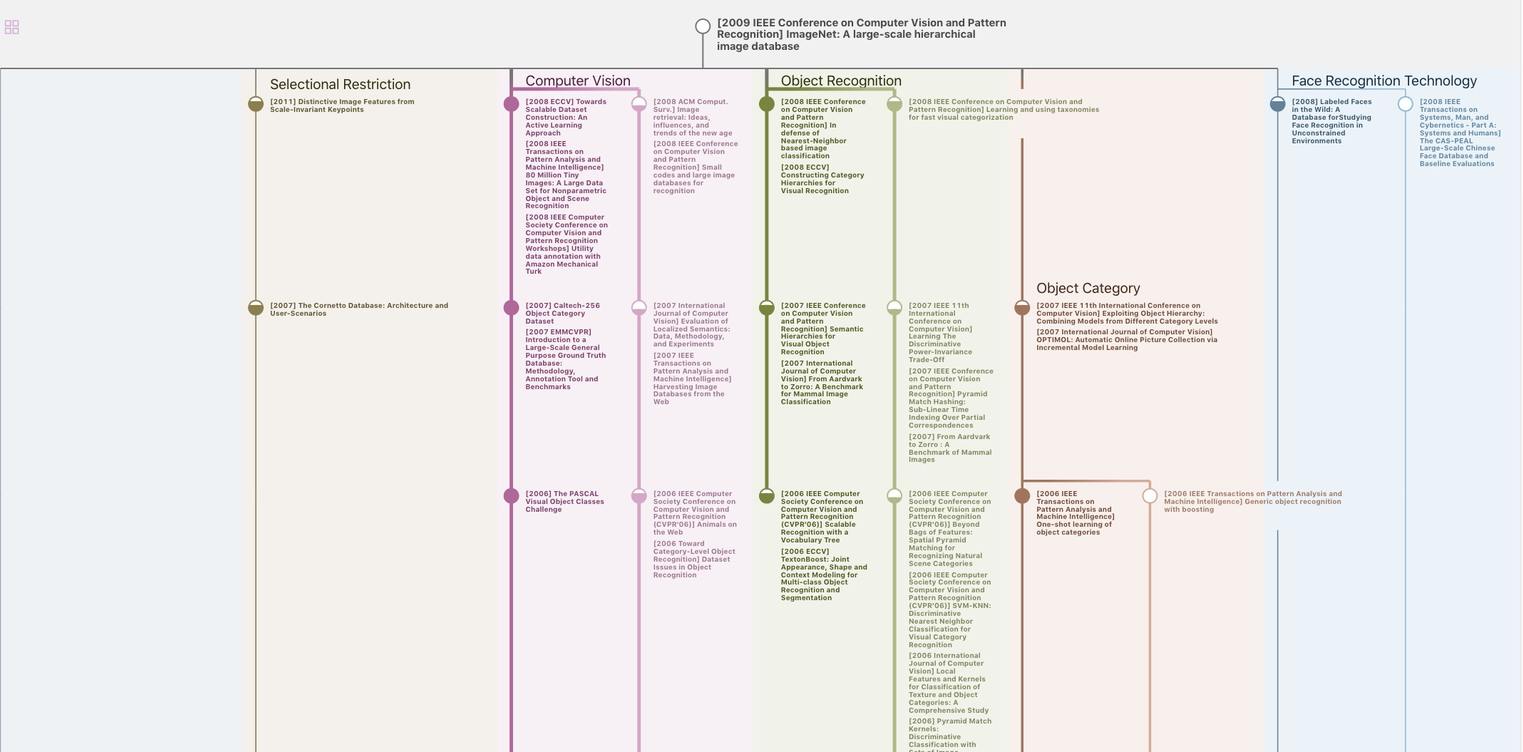
生成溯源树,研究论文发展脉络
Chat Paper
正在生成论文摘要