End-to-End Automatic Morphological Classification of Intracranial Pressure Pulse Waveforms Using Deep Learning
IEEE journal of biomedical and health informatics(2021)CCF CSCI 2区SCI 1区
Wroclaw Univ Sci & Technol | Wroclaw University of Science and Technol-ogy partment of Biomedical Engineering | Wroclaw Medical University Department of Anaesthesiology and In-tensive Care | Wroclaw Med Univ
- Pretraining has recently greatly promoted the development of natural language processing (NLP)
- We show that M6 outperforms the baselines in multimodal downstream tasks, and the large M6 with 10 parameters can reach a better performance
- We propose a method called M6 that is able to process information of multiple modalities and perform both single-modal and cross-modal understanding and generation
- The model is scaled to large model with 10 billion parameters with sophisticated deployment, and the 10 -parameter M6-large is the largest pretrained model in Chinese
- Experimental results show that our proposed M6 outperforms the baseline in a number of downstream tasks concerning both single modality and multiple modalities We will continue the pretraining of extremely large models by increasing data to explore the limit of its performance
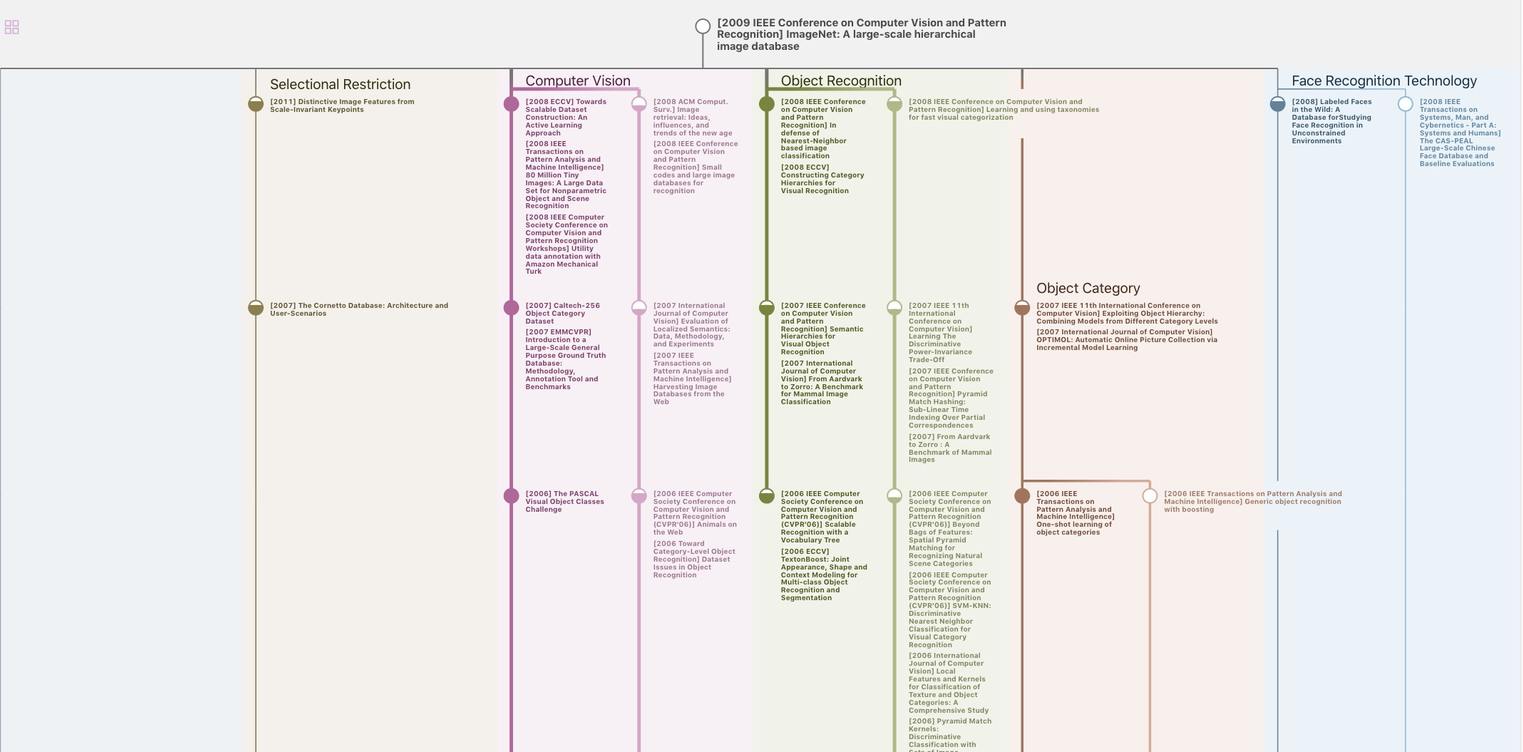
被引用9
Analysis of the Shape of Intracranial Pressure Pulse Waveform in Traumatic Brain Injury Patients
被引用5
被引用0
被引用9
Peak Appearance Time in Pulse Waveforms of Intracranial Pressure and Cerebral Blood Flow Velocity
被引用3
被引用1
A Deep Learning-Based Automated Framework for Subpeak Designation on Intracranial Pressure Signals
被引用0
Rheoencephalography: A Non-Invasive Method for Neuromonitoring
被引用0
被引用0
Artificial Intelligence and Machine Learning Applications in Critically Ill Brain Injured Patients
被引用1
被引用0