Brain connectivity markers in advanced Parkinson’s disease for predicting mild cognitive impairment
EUROPEAN RADIOLOGY(2021)
摘要
Objectives Mild cognitive impairment (MCI) is a well-defined non-motor manifestation and a harbinger of dementia in Parkinson’s disease. This study is to investigate brain connectivity markers of MCI using diffusion tensor imaging and resting-state functional MRI, and help MCI diagnosis in PD patients. Methods We evaluated 131 advanced PD patients (disease duration > 5 years; 59 patients with MCI) and 48 healthy control subjects who underwent a diffusion-weighted and resting-state functional MRI scanning. The patients were randomly assigned to training ( n = 100) and testing ( n = 31) groups. According to the Brainnetome Atlas, ROI-based structural and functional connectivity analysis was employed to extract connectivity features. To identify features with significant discriminative power for patient classification, all features were put into an all-relevant feature selection procedure within cross-validation loops. Results Nine features were identified to be significantly relevant to patient classification. They showed significant differences between PD patients with and without MCI and positively correlated with the MoCA score. Five of them did not differ between general MCI subjects and healthy controls from the ADNI database, which suggested that they could uniquely play a part in the MCI diagnosis of PD. On basis of these relevant features, the random forest model constructed from the training group achieved an accuracy of 83.9% in the testing group, to discriminate patients with and without MCI. Conclusions The results of our study provide preliminary evidence that structural and functional connectivity abnormalities may contribute to cognitive impairment and allow to predict the outcome of MCI diagnosis in PD. Key Points • Nine MCI markers were identified using an all-relevant feature selection procedure. • Five of nine markers differed between MCI and NC in PD, but not in general persons. • A random forest model achieved an accuracy of 83.9% for MCI diagnosis in PD.
更多查看译文
关键词
Parkinson disease, Cognition disorders, Diffusion tensor imaging, Magnetic resonance imaging
AI 理解论文
溯源树
样例
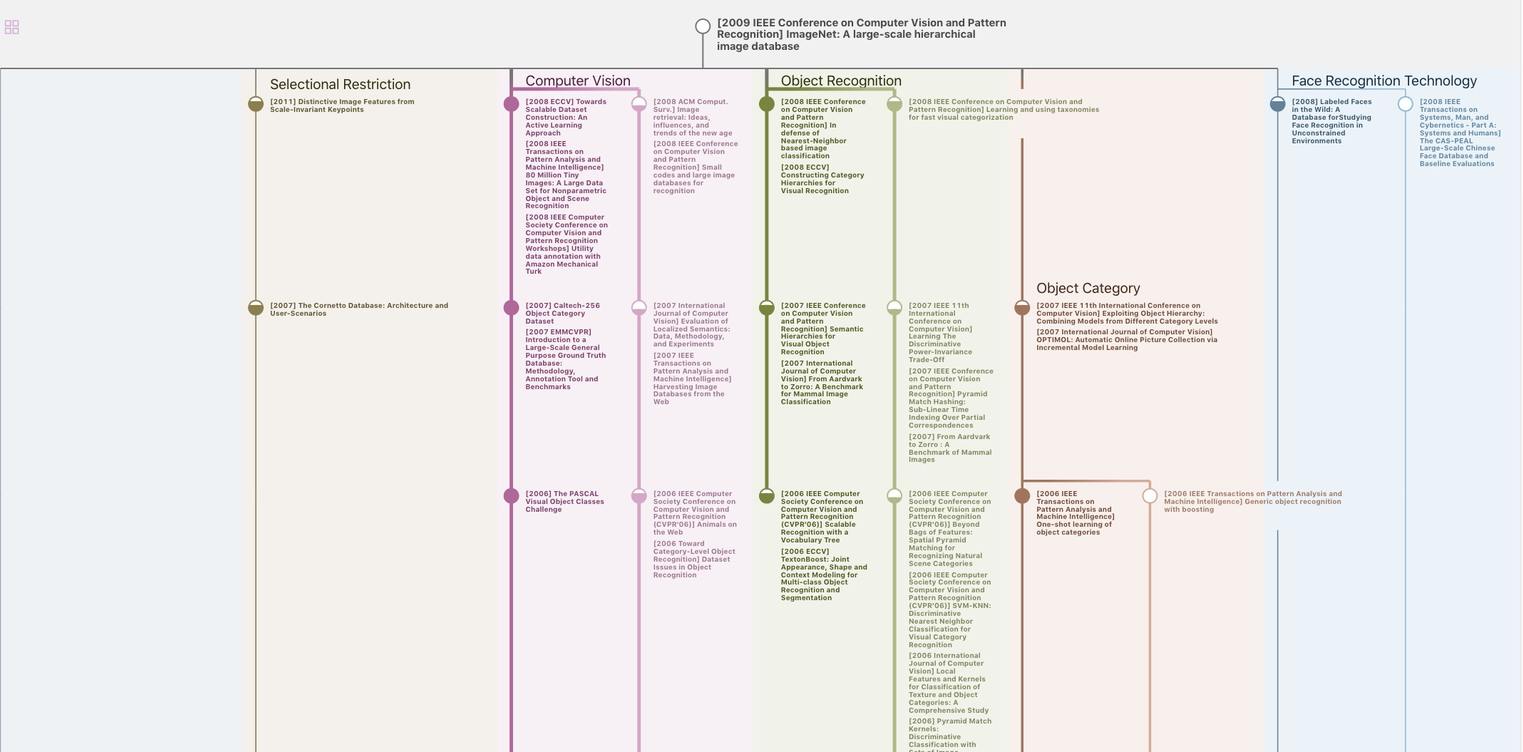
生成溯源树,研究论文发展脉络
Chat Paper
正在生成论文摘要