A Cascade Model-Aware Generative Adversarial Example Detection Method
Tsinghua science and technology/Tsinghua Science and Technology(2021)
摘要
Deep Neural Networks(DNNs) are demonstrated to be vulnerable to adversarial examples, which are elaborately crafted to fool learning models. Since the accuracy and robustness of DNNs are at odds for the adversarial training method, the adversarial example detection algorithms check whether the specific example is adversarial, which is promising to solve the issue of the adversarial example. However, among the existing methods,model-aware detection methods do not generalize well, while the detection accuracies of the generative-based methods are lower compared to the model-aware methods. In this paper, we propose a cascade model-aware generative adversarial example detection method, namely CMAG. CMAG consists of two first-order reconstructors and a second-order reconstructor, which can illustrate what the model sees to the human by reconstructing the logit and feature maps of the last convolution layer. Experimental results demonstrate that our method is effective and is more interpretable compared to some state-of-the-art methods.
更多查看译文
关键词
information security,Deep Neural Network (DNN),adversarial example detection
AI 理解论文
溯源树
样例
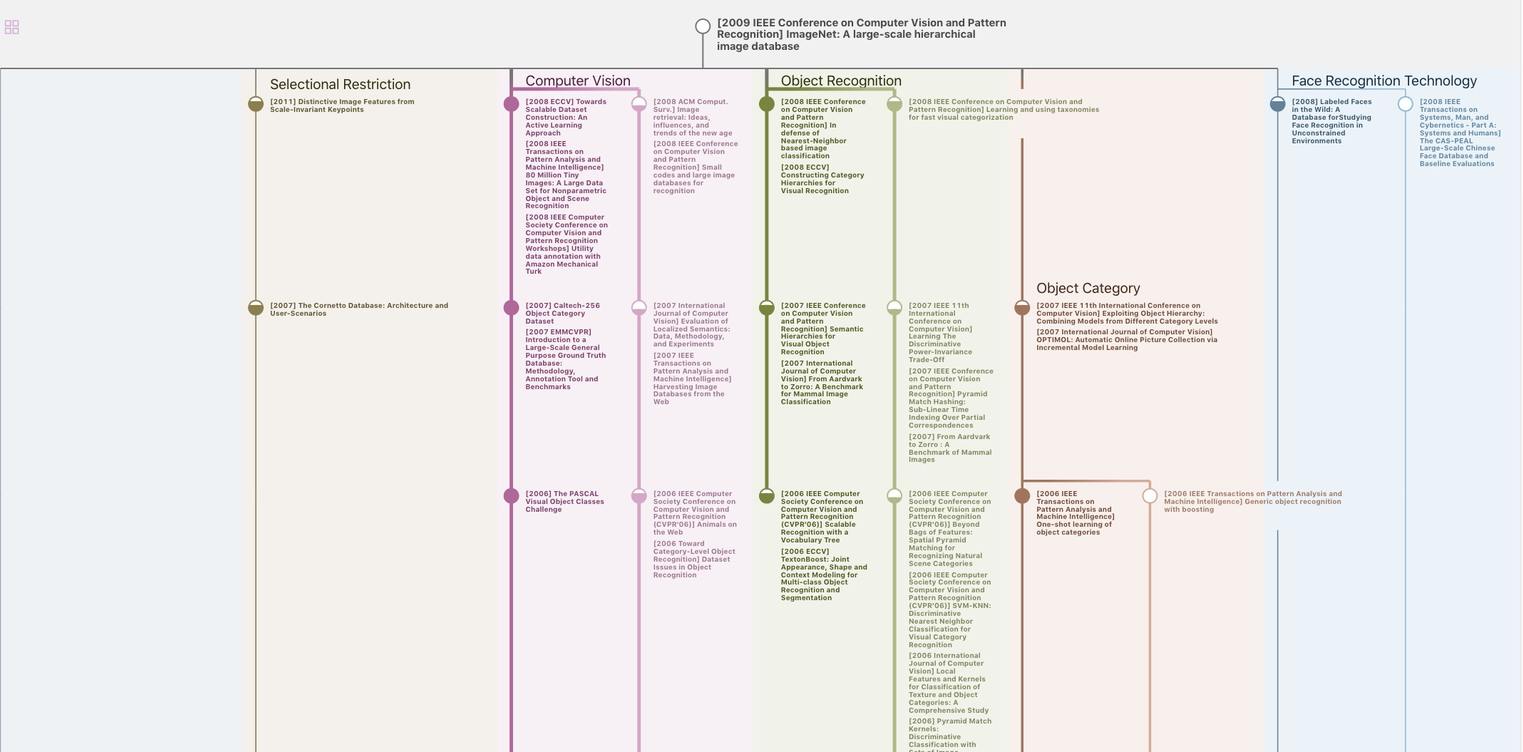
生成溯源树,研究论文发展脉络
Chat Paper
正在生成论文摘要