DisTop: Discovering a Topological Representation to Learn Diverse and Rewarding Skills
IEEE TRANSACTIONS ON COGNITIVE AND DEVELOPMENTAL SYSTEMS(2023)
摘要
An efficient way for a deep reinforcement learning (RL) agent to explore in sparse-rewards settings can be to learn a set of skills that achieves a uniform distribution of terminal states. We introduce DisTop, a new model that simultaneously learns diverse skills and focuses on improving rewarding skills. DisTop progressively builds a discrete topology of the environment using an unsupervised contrastive loss, a growing network, and a goal-conditioned policy. Using this topology, a state-independent hierarchical policy can select which skill to execute and learn. In turn, the new set of visited states allows an improved learned representation. Our experiments emphasize that DisTop is agnostic to the ground state representation and that the agent can discover the topology of its environment whether the states are high-dimensional binary data, images, or proprioceptive inputs. We demonstrate that this paradigm is competitive on MuJoCo benchmarks with state-of-the-art (SOTA) algorithms on both single-task dense rewards and diverse skill discovery without rewards. By combining these two aspects, we show that DisTop outperforms a SOTA hierarchical RL algorithm when rewards are sparse. We believe DisTop opens new perspectives by showing that bottom-up skill discovery combined with dynamic-aware representation learning can tackle different complex state spaces and reward settings.
更多查看译文
关键词
Deep reinforcement learning (RL),developmental learning,hierarchical learning,intrinsic motivation.
AI 理解论文
溯源树
样例
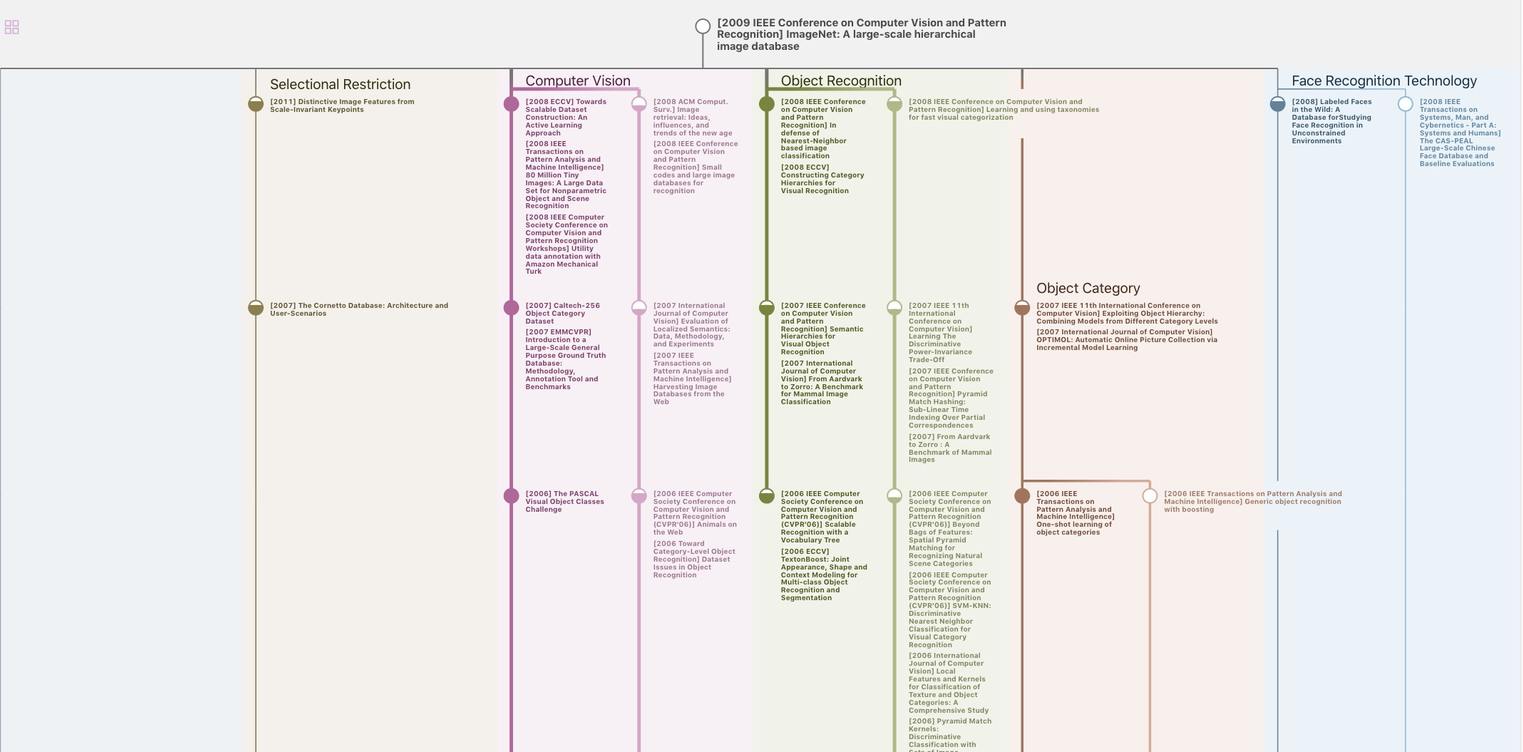
生成溯源树,研究论文发展脉络
Chat Paper
正在生成论文摘要