Learning to Select: A Fully Attentive Approach for Novel Object Captioning
International Multimedia Conference(2021)
摘要
ABSTRACTImage captioning models have lately shown impressive results when applied to standard datasets. Switching to real-life scenarios, however, constitutes a challenge due to the larger variety of visual concepts which are not covered in existing training sets. For this reason, novel object captioning (NOC) has recently emerged as a paradigm to test captioning models on objects which are unseen during the training phase. In this paper, we present a novel approach for NOC that learns to select the most relevant objects of an image, regardless of their adherence to the training set, and to constrain the generative process of a language model accordingly. Our architecture is fully-attentive and end-to-end trainable, also when incorporating constraints. We perform experiments on the held-out COCO dataset, where we demonstrate improvements over the state of the art, both in terms of adaptability to novel objects and caption quality.
更多查看译文
关键词
novel object captioning, region selector, constrained beam search
AI 理解论文
溯源树
样例
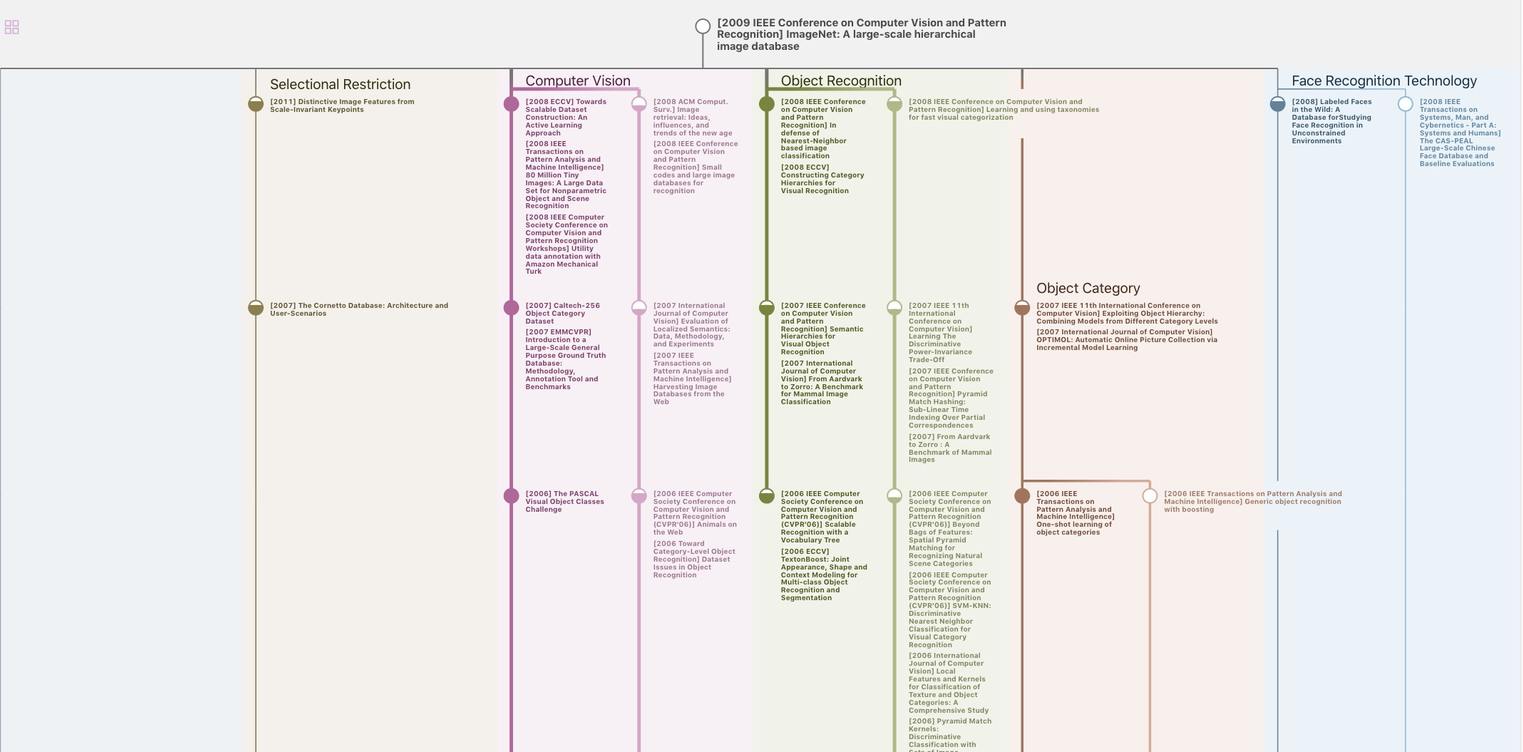
生成溯源树,研究论文发展脉络
Chat Paper
正在生成论文摘要