Generative adversarial network for Table-to-Text generation
Neurocomputing(2021)
摘要
Table-to-Text generation aims to generate descriptions which can be viewed as a set of field-value records for factual tables. Despite the significant progress, the state-of-the-art models suffer from two major issues: Nonfluency and Divergence. Nonfluency means descriptions generated by models are not as fluent as those generated by humans, and thus can be distinguished easily. Divergence refers to the fact that the generated sentences contain information which can not be concluded from factual tables. This could be attributed to that most neural models are trained with the Maximum Likelihood Estimation (MLE) loss and use divergence-contained references as the ground truth, which forces the models to learn what cannot be inferred from the source to some extent. Motivated by the limitations of current models, we propose a novel GAN-based model with adversarial learning mechanism, which simultaneously trains a generative model G and a discriminative model D, to address Nonfluency and Divergence issues in Table-to-Text generation. Specifically, we build the generator G as an agent of reinforcement learning with a sequence-to-sequence architecture, which takes the raw data as input and predicts the generated sentences. Meanwhile, we build the discriminator D with a Convolutional Neural Network (CNN) to calculate rewards to measure the fluency of generations. To judge the fidelity of generations with regard to the original table more accurately, we also calculate the rewards from BLEU Table. With the fusion rewards from CNN and BLEU-Table, our methods outperform the baselines by a large margin on the WikiBio and Wiki3C benchmarks evaluated with BLEU, ROURGE, and PARENT. Specifically, our models achieve 49.0 (BLEU-4), 37.8 (ROUGE-4) and 45.4 (PARENT) on WikiBio, as well as 12.9 (BLEU-4) and 6.9 (ROUGE-4) on Wiki3C. More importantly, we construct a new Wiki3C dataset that improves the insufficiency of datasets and promote the progress in Table-to-Text generation. (c) 2021 Elsevier B.V. All rights reserved.
更多查看译文
关键词
Table-to-Text generation,Natural language generation,Generative adversarial network
AI 理解论文
溯源树
样例
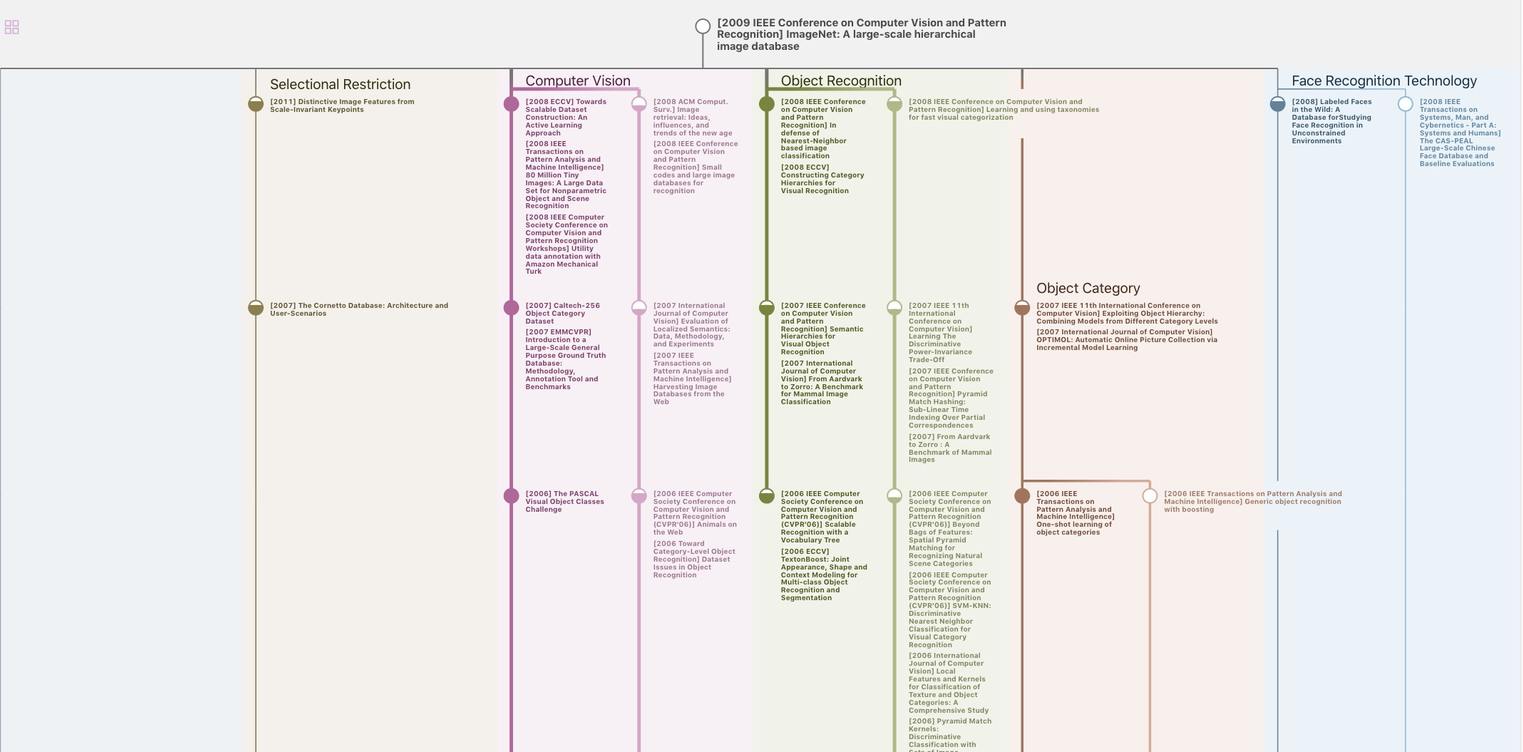
生成溯源树,研究论文发展脉络
Chat Paper
正在生成论文摘要