AutoDim: Field-aware Embedding Dimension Searchin Recommender Systems
International World Wide Web Conference(2021)
摘要
ABSTRACT Practical large-scale recommender systems usually contain thousands of feature fields from users, items, contextual information, and their interactions. Most of them empirically allocate a unified dimension to all feature fields, which is memory inefficient. Thus it is highly desired to assign various embedding dimensions to different feature fields according to their importance and predictability. Due to the large amounts of feature fields and the nuanced relationship between embedding dimensions with feature distributions and neural network architectures, manually allocating embedding dimensions in practical recommender systems can be challenging. To this end, we propose an AutoML-based framework (AutoDim) in this paper, which can automatically select dimensions for different feature fields in a data-driven fashion. Specifically, we first proposed an end-to-end differentiable framework that can calculate the weights over various dimensions in a soft and continuous manner for feature fields, and an AutoML-based optimization algorithm; then, we derive a hard and discrete embedding component architecture according to the maximal weights and retrain the whole recommender framework. We conduct extensive experiments on benchmark datasets to validate the effectiveness of AutoDim.
更多查看译文
关键词
Embedding, Recommender System, AutoML
AI 理解论文
溯源树
样例
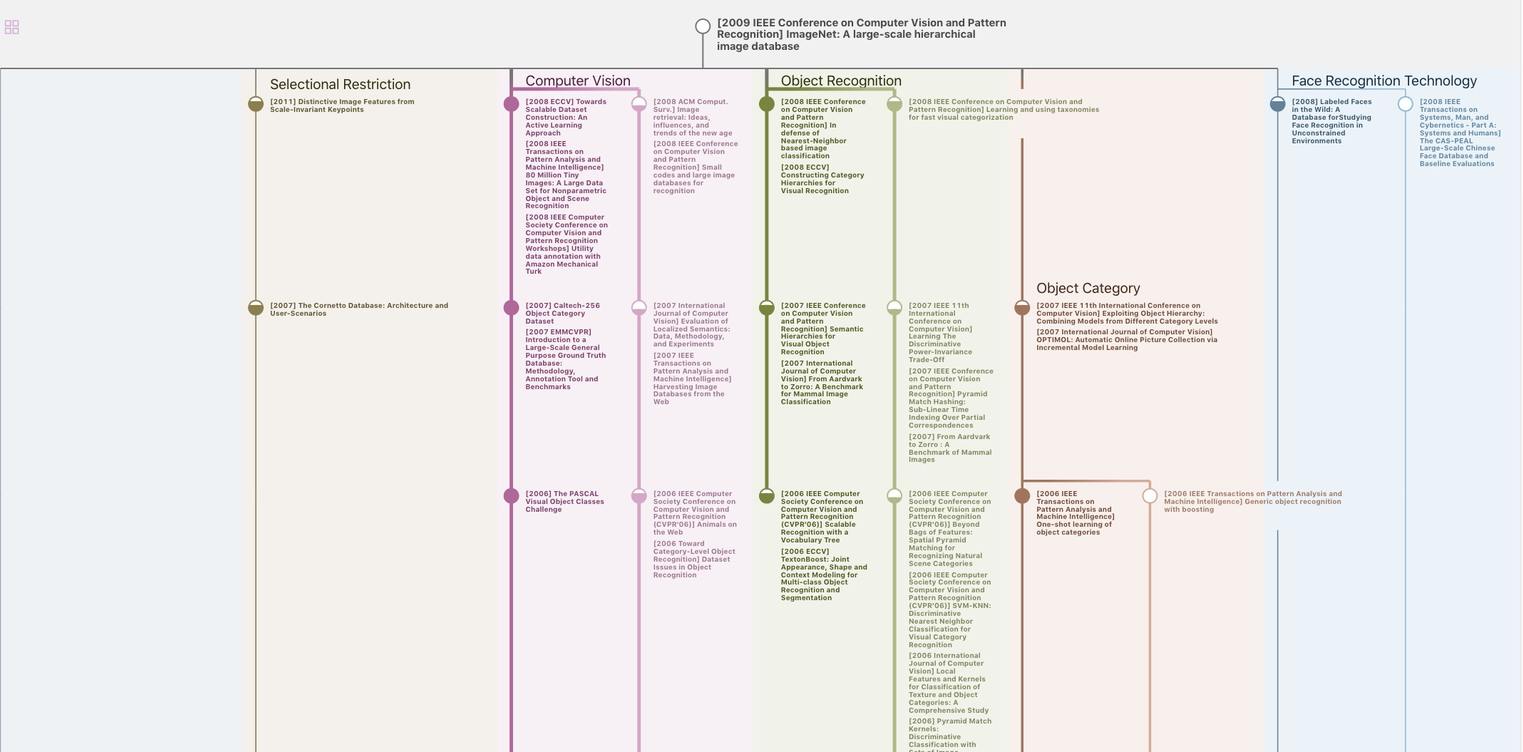
生成溯源树,研究论文发展脉络
Chat Paper
正在生成论文摘要