Dynamic Thermal Corridor May Connect Endangered Loggerhead Sea Turtles Across the Pacific Ocean
Frontiers in Marine Science(2021)SCI 2区SCI 1区
Stanford Univ | NOAA Natl Marine Fisheries Serv | Golden Honu Serv Oceania | Univ Hawaii Manoa | Port Nagoya Publ Aquarium | Kochi Univ | Hawaii Preparatory Acad
Abstract
The North Pacific Loggerhead sea turtle (Caretta caretta) undergoes one of the greatest of all animal migrations, nesting exclusively in Japan and re-emerging several years later along important foraging grounds in the eastern North Pacific. Yet the mechanisms that connect these disparate habitats during what is known as the “lost years” have remained poorly understood. Here, we develop a new hypothesis regarding a possible physical mechanism for habitat connectivity – an intermittent “thermal corridor” – using remotely sensed oceanography and 6 juvenile loggerhead sea turtles that formed part of a 15 year tracking dataset of 231 individuals (1997–2013). While 97% of individuals remained in the Central North Pacific, these 6 turtles (about 3%), continued an eastward trajectory during periods associated with anomalously warm ocean conditions. These few individuals provided a unique opportunity to examine previously unknown recruitment pathways. To support this hypothesis, we employed an independently derived data set using novel stable isotope analyses of bone growth layers and assessed annual recruitment over the same time period (n = 33, 1997–2012). We suggest evidence of a thermal corridor that may allow for pulsed recruitment of loggerheads to the North American coast as a function of ocean conditions. Our findings offer, for the first time, the opportunity to explore the development of a dynamic ocean corridor for this protected species, illuminating a longstanding mystery in sea turtle ecology.
MoreTranslated text
Key words
loggerhead sea turtle,distribution,satellite remote sensing,sea surface temperature,habitat connectivity,migration,corridors
PDF
View via Publisher
AI Read Science
AI Summary
AI Summary is the key point extracted automatically understanding the full text of the paper, including the background, methods, results, conclusions, icons and other key content, so that you can get the outline of the paper at a glance.
Example
Background
Key content
Introduction
Methods
Results
Related work
Fund
Key content
- Pretraining has recently greatly promoted the development of natural language processing (NLP)
- We show that M6 outperforms the baselines in multimodal downstream tasks, and the large M6 with 10 parameters can reach a better performance
- We propose a method called M6 that is able to process information of multiple modalities and perform both single-modal and cross-modal understanding and generation
- The model is scaled to large model with 10 billion parameters with sophisticated deployment, and the 10 -parameter M6-large is the largest pretrained model in Chinese
- Experimental results show that our proposed M6 outperforms the baseline in a number of downstream tasks concerning both single modality and multiple modalities We will continue the pretraining of extremely large models by increasing data to explore the limit of its performance
Try using models to generate summary,it takes about 60s
Must-Reading Tree
Example
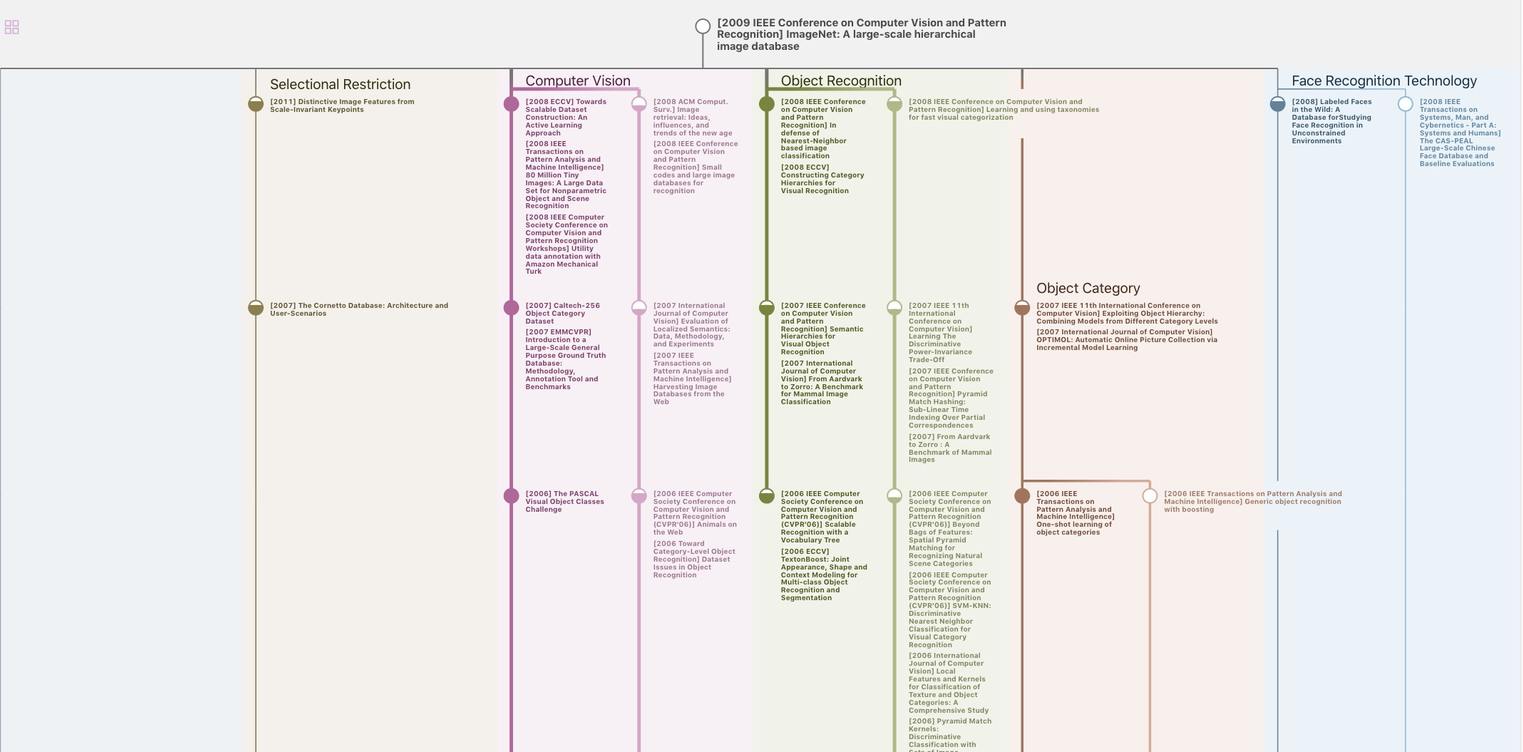
Generate MRT to find the research sequence of this paper
Related Papers
Frontiers in Marine Science 2021
被引用2
Data Disclaimer
The page data are from open Internet sources, cooperative publishers and automatic analysis results through AI technology. We do not make any commitments and guarantees for the validity, accuracy, correctness, reliability, completeness and timeliness of the page data. If you have any questions, please contact us by email: report@aminer.cn
Chat Paper
GPU is busy, summary generation fails
Rerequest