Using Tri-Axial Accelerometer Loggers to Identify Spawning Behaviours of Large Pelagic Fish.
Movement Ecology(2021)
Flinders University | Harry Butler Institute | School of Natural Sciences
Abstract
Background Tri-axial accelerometers have been used to remotely describe and identify in situ behaviours of a range of animals without requiring direct observations. Datasets collected from these accelerometers (i.e. acceleration, body position) are often large, requiring development of semi-automated analyses to classify behaviours. Marine fishes exhibit many “burst” behaviours with high amplitude accelerations that are difficult to interpret and differentiate. This has constrained the development of accurate automated techniques to identify different “burst” behaviours occurring naturally, where direct observations are not possible. Methods We trained a random forest machine learning algorithm based on 624 h of accelerometer data from six captive yellowtail kingfish during spawning periods. We identified five distinct behaviours (swim, feed, chafe, escape, and courtship), which were used to train the model based on 58 predictive variables. Results Overall accuracy of the model was 94%. Classification of each behavioural class was variable; F 1 scores ranged from 0.48 (chafe) – 0.99 (swim). The model was subsequently applied to accelerometer data from eight free-ranging kingfish, and all behaviour classes described from captive fish were predicted by the model to occur, including 19 events of courtship behaviours ranging from 3 s to 108 min in duration. Conclusion Our findings provide a novel approach of applying a supervised machine learning model on free-ranging animals, which has previously been predominantly constrained to direct observations of behaviours and not predicted from an unseen dataset. Additionally, our findings identify typically ambiguous spawning and courtship behaviours of a large pelagic fish as they naturally occur.
MoreTranslated text
Key words
Biologging,Courtship,Kingfish,Captive,Machine learning
PDF
View via Publisher
AI Read Science
Must-Reading Tree
Example
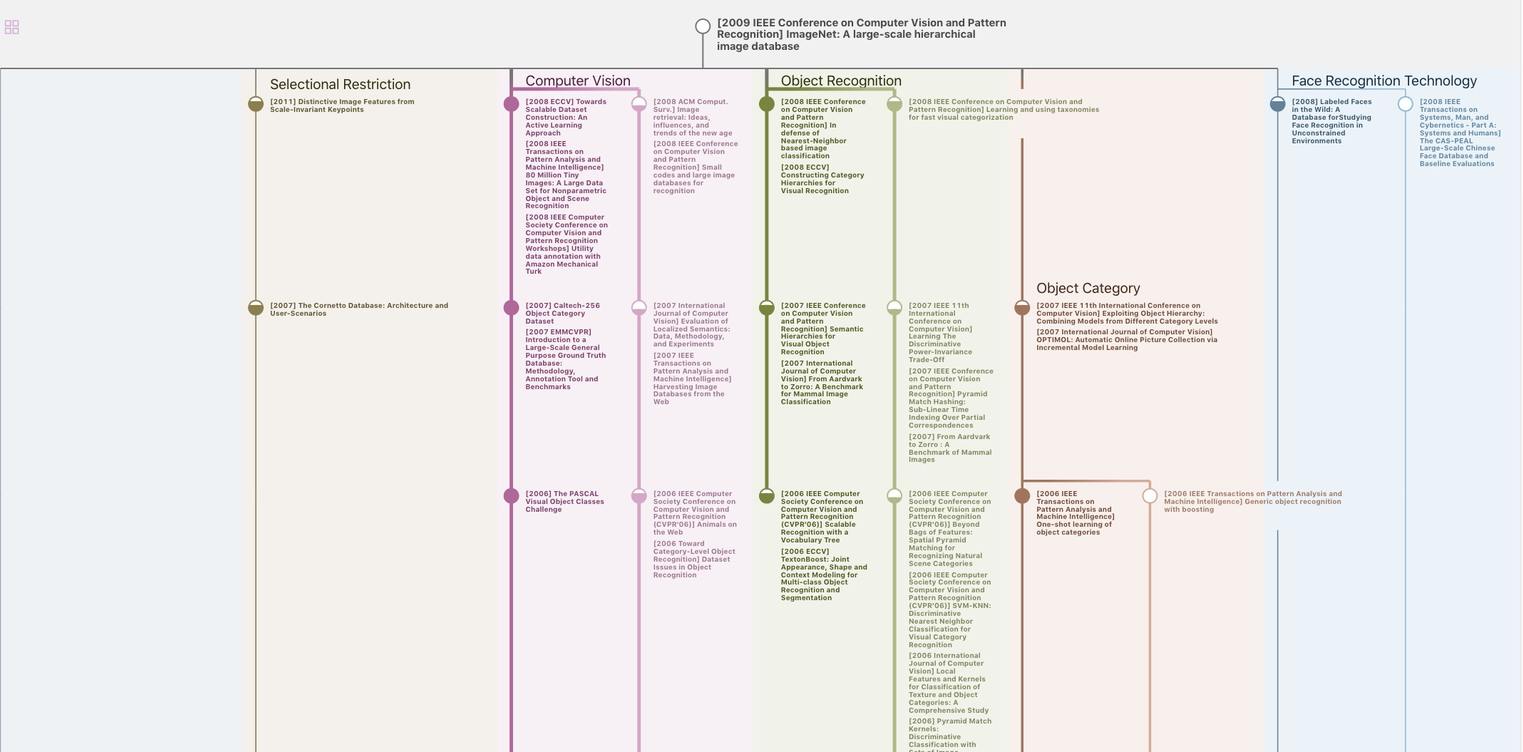
Generate MRT to find the research sequence of this paper
Related Papers
2009
被引用143 | 浏览
2005
被引用125 | 浏览
2008
被引用48 | 浏览
2009
被引用52 | 浏览
2001
被引用185 | 浏览
2003
被引用59 | 浏览
2001
被引用118 | 浏览
2006
被引用554 | 浏览
2012
被引用249 | 浏览
2009
被引用350 | 浏览
2015
被引用112 | 浏览
2015
被引用43 | 浏览
2011
被引用110 | 浏览
2014
被引用56 | 浏览
2016
被引用29 | 浏览
2018
被引用20 | 浏览
2017
被引用26 | 浏览
Data Disclaimer
The page data are from open Internet sources, cooperative publishers and automatic analysis results through AI technology. We do not make any commitments and guarantees for the validity, accuracy, correctness, reliability, completeness and timeliness of the page data. If you have any questions, please contact us by email: report@aminer.cn
Chat Paper
GPU is busy, summary generation fails
Rerequest