The Landscape and Gaps in Open Source Fairness Toolkits.
ACM Conference on Human Factors in Computing Systems (CHI)(2020)CCF A
Abstract
With the surge in literature focusing on the assessment and mitigation of unfair outcomes in algorithms, several open source ‘fairness toolkits’ recently emerged to make such methods widely accessible. However, little studied are the differences in approach and capabilities of existing fairness toolkits, and their fit-for-purpose in commercial contexts. Towards this, this paper identifies the gaps between the existing open source fairness toolkit capabilities and the industry practitioners’ needs. Specifically, we undertake a comparative assessment of the strengths and weaknesses of six prominent open source fairness toolkits, and investigate the current landscape and gaps in fairness toolkits through an exploratory focus group, a semi-structured interview, and an anonymous survey of data science/machine learning (ML) practitioners. We identify several gaps between the toolkits’ capabilities and practitioner needs, highlighting areas requiring attention and future directions towards tooling that better support ‘fairness in practice.’
MoreTranslated text
Key words
fairness,bias,algorithm auditing,open source toolkits,fairness toolkits,algorithmic fairness,bias detection,bias mitigation
PDF
View via Publisher
AI Read Science
AI Summary
AI Summary is the key point extracted automatically understanding the full text of the paper, including the background, methods, results, conclusions, icons and other key content, so that you can get the outline of the paper at a glance.
Example
Background
Key content
Introduction
Methods
Results
Related work
Fund
Key content
- Pretraining has recently greatly promoted the development of natural language processing (NLP)
- We show that M6 outperforms the baselines in multimodal downstream tasks, and the large M6 with 10 parameters can reach a better performance
- We propose a method called M6 that is able to process information of multiple modalities and perform both single-modal and cross-modal understanding and generation
- The model is scaled to large model with 10 billion parameters with sophisticated deployment, and the 10 -parameter M6-large is the largest pretrained model in Chinese
- Experimental results show that our proposed M6 outperforms the baseline in a number of downstream tasks concerning both single modality and multiple modalities We will continue the pretraining of extremely large models by increasing data to explore the limit of its performance
Try using models to generate summary,it takes about 60s
Must-Reading Tree
Example
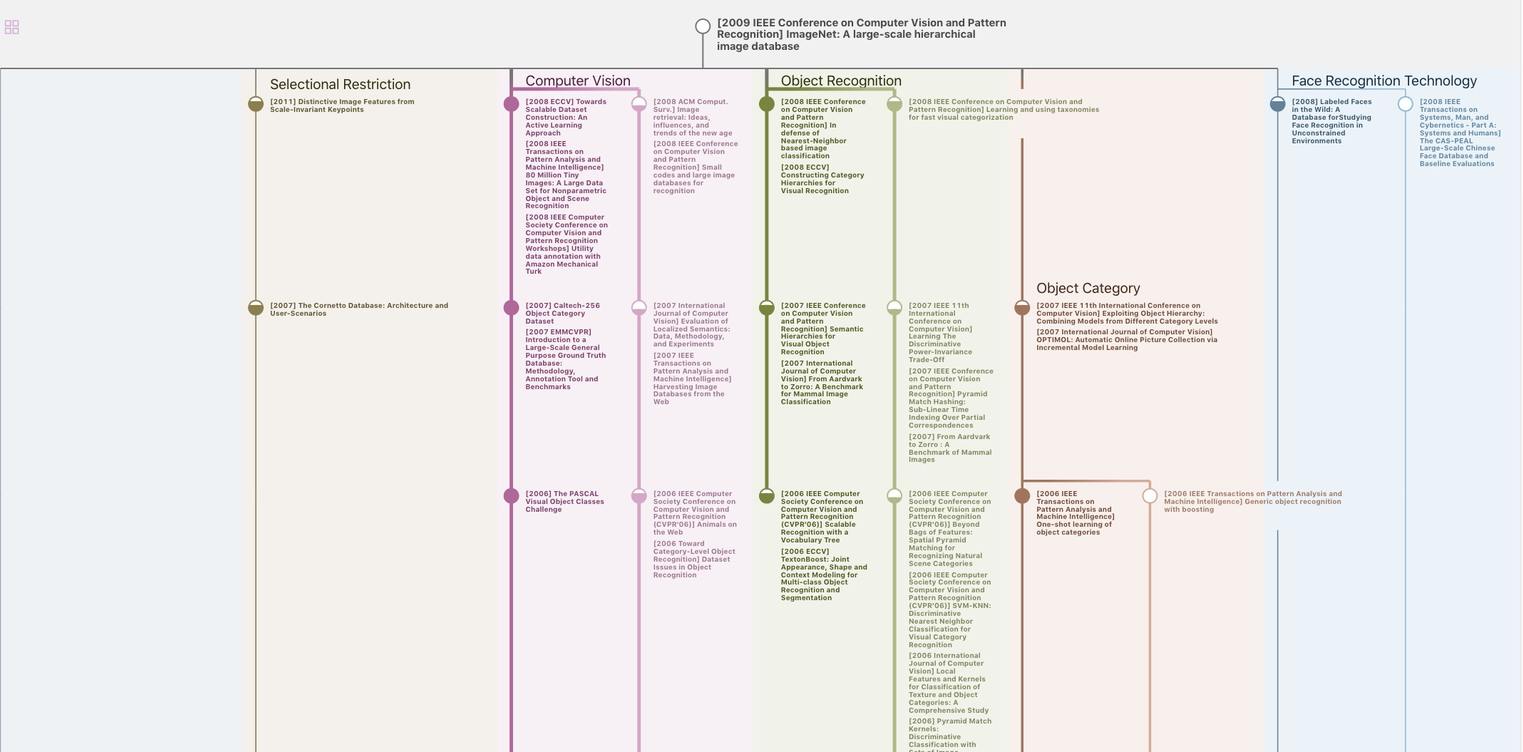
Generate MRT to find the research sequence of this paper
Related Papers
2011
被引用761 | 浏览
2018
被引用54 | 浏览
2018
被引用569 | 浏览
2019
被引用192 | 浏览
2019
被引用1018 | 浏览
2018
被引用1808 | 浏览
2020
被引用15 | 浏览
2019
被引用1808 | 浏览
2021
被引用110 | 浏览
Data Disclaimer
The page data are from open Internet sources, cooperative publishers and automatic analysis results through AI technology. We do not make any commitments and guarantees for the validity, accuracy, correctness, reliability, completeness and timeliness of the page data. If you have any questions, please contact us by email: report@aminer.cn
Chat Paper
去 AI 文献库 对话