Self-learning for Received Signal Strength Map Reconstruction with Neural Architecture Search
ARTIFICIAL NEURAL NETWORKS AND MACHINE LEARNING, ICANN 2021, PT V(2021)
摘要
In this paper, we present a Neural Network (NN) model based on Neural Architecture Search (NAS) and self-learning for received signal strength (RSS) map reconstruction out of sparse single-snapshot input measurements, in the case where data-augmentation by side deterministic simulations cannot be performed. The approach first finds an optimal NN architecture and simultaneously train the deduced model over some ground-truth measurements of a given (RSS) map. These ground-truth measurements along with the predictions of the model over a set of randomly chosen points are then used to train a second NN model having the same architecture. Experimental results show that signal predictions of this second model outperforms non-learning based interpolation state-of-the-art techniques and NN models with no architecture search on five large-scale maps of RSS measurements.
更多查看译文
关键词
Neural Architecture Search,Self-learning,Received signal strength,Radio mapping
AI 理解论文
溯源树
样例
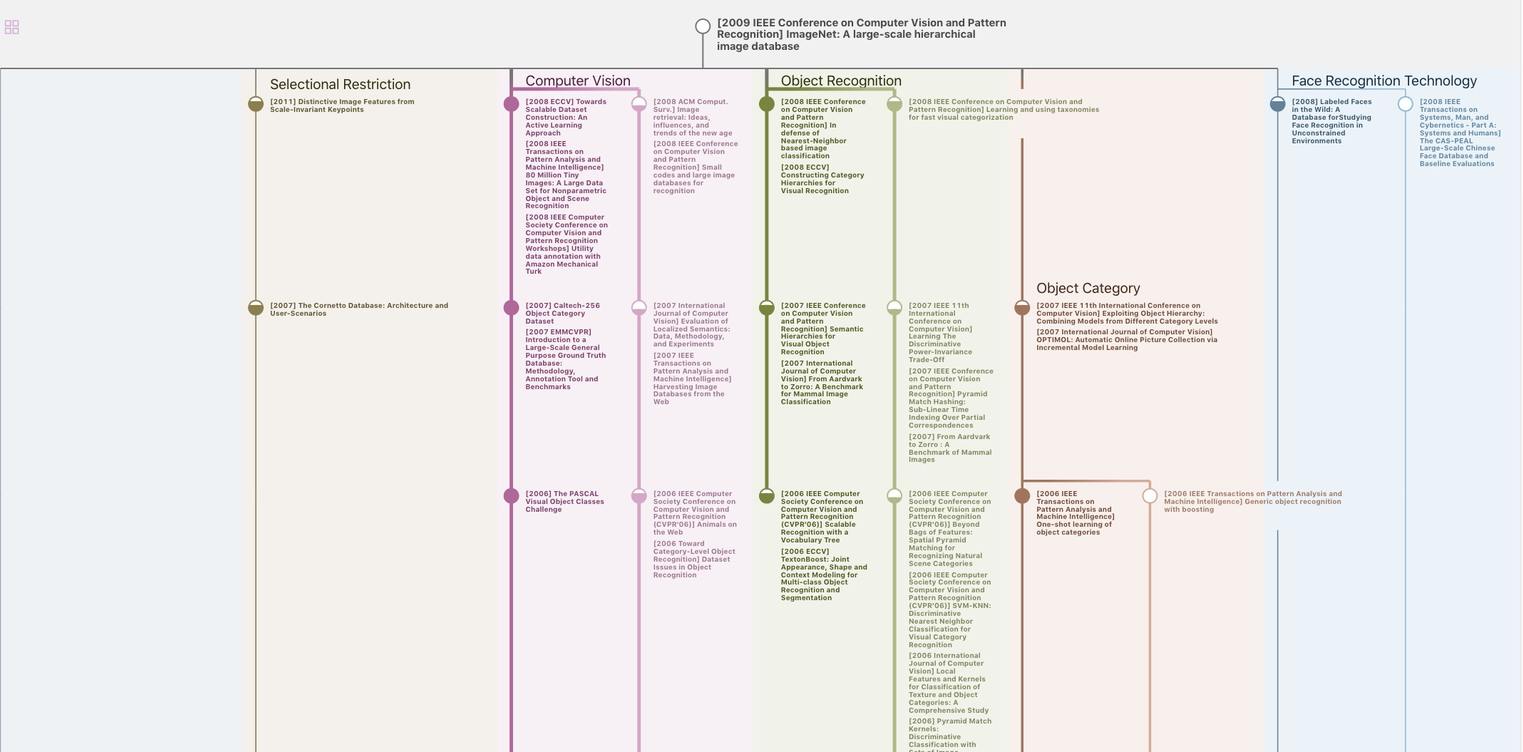
生成溯源树,研究论文发展脉络
Chat Paper
正在生成论文摘要