Voxel-level Siamese Representation Learning for Abdominal Multi-Organ Segmentation
COMPUTER METHODS AND PROGRAMS IN BIOMEDICINE(2022)
摘要
Background and Objective: Recent works in medical image segmentation have actively explored various deep learning architectures or objective functions to encode high-level features from volumetric data owing to limited image annotations. However, most existing approaches tend to ignore cross-volume global context and define context relations in the decision space. In this work, we propose a novel voxel-level Siamese representation learning method for abdominal multi-organ segmentation to improve representation space. Methods: The proposed method enforces voxel-wise feature relations in the representation space for leveraging limited datasets more comprehensively to achieve better performance. Inspired by recent progress in contrastive learning, we suppressed voxel-wise relations from the same class to be projected to the same point without using negative samples. Moreover, we introduce a multi-resolution context aggregation method that aggregates features from multiple hidden layers, which encodes both the global and local contexts for segmentation. Results: Our experiments on the multi-organ dataset outperformed the existing approaches by 2% in Dice score coefficient. The qualitative visualizations of the representation spaces demonstrate that the improvements were gained primarily by a disentangled feature space. Conclusion: Our new representation learning method successfully encoded high-level features in the representation space by using a limited dataset, which showed superior accuracy in the medical image segmentation task compared to other contrastive loss-based methods. Moreover, our method can be easily applied to other networks without using additional parameters in the inference. (C) 2021 Elsevier B.V. All rights reserved.
更多查看译文
关键词
Abdominal ct segmentation,Medical image segmentation,Multi-organ segmentation,Representation learning,Siamese network
AI 理解论文
溯源树
样例
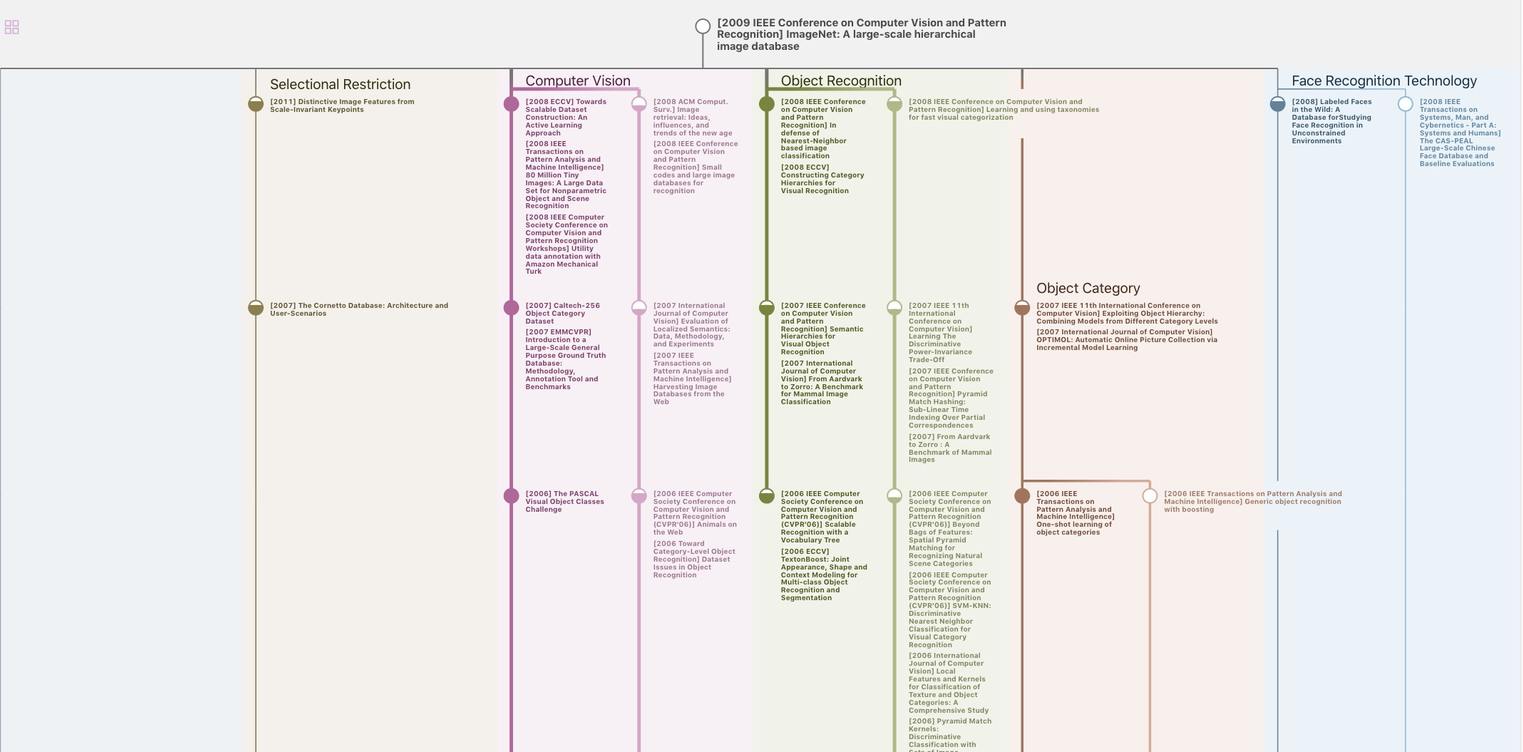
生成溯源树,研究论文发展脉络
Chat Paper
正在生成论文摘要