Data-Driven Failure Mode And Effect Analysis (Fmea) To Enhance Maintenance Planning
COMPUTERS IN INDUSTRY(2021)
摘要
Nowadays, the availability of data from the manufacturing environment, such as process and operation related data or past maintenance activities enable new possibilities for advanced data analytics like prediction of failure behavior. Possible predictions could consider faults of specific components or even the current product and component properties.The paper presents a data-driven Failure Mode and Effect Analysis (FMEA) methodology by using deep learning models on historical and operational data from the use stage of industrial investment goods.The developed methodology is supposed to support the maintenance planning for industrial investment goods by enhancing transparency and providing decision support. The developed framework is applied to and validated by a case study from the aviation sector. The results show that the accuracy of the fault prediction is around 95 %. By integrating these results into a data-driven FMEA framework, risk and failure occurrence estimations are no longer subjective. Especially the estimation of failure probabilities no longer solely depends on the experience and knowledge from employees.(c) 2021 Elsevier B.V. All rights reserved.
更多查看译文
关键词
Failure mode and effect analysis (FMEA), Maintenance, Fault prediction, Decision support, Data analytics, Deep learning, Artificial intelligence
AI 理解论文
溯源树
样例
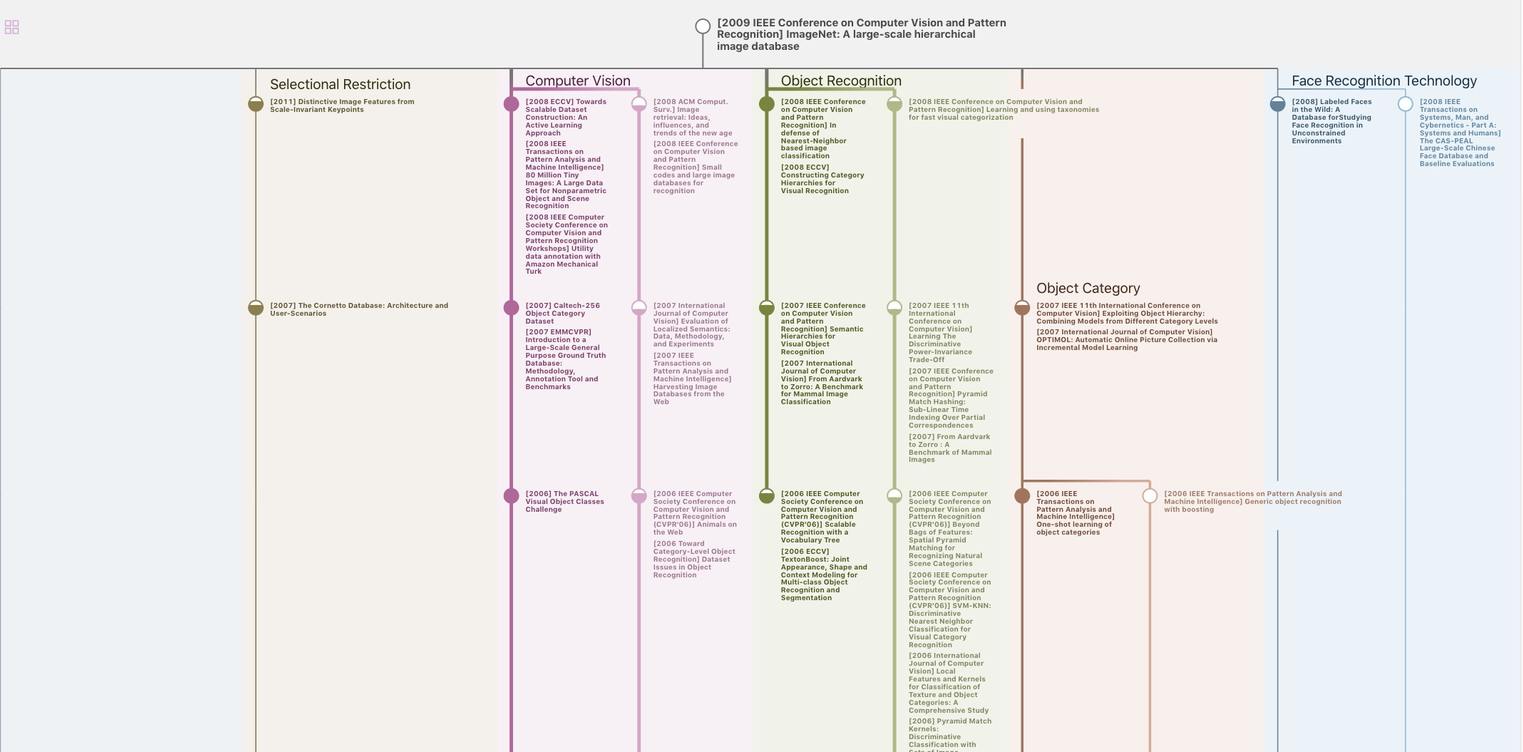
生成溯源树,研究论文发展脉络
Chat Paper
正在生成论文摘要