Lightweight Pixel-Wise Segmentation For Efficient Concrete Crack Detection Using Hierarchical Convolutional Neural Network
SMART MATERIALS AND STRUCTURES(2021)
摘要
The aging of concrete structures is a threat to public safety; therefore, maintenance and repair of these structures have been highly emphasized. However, regular inspections to detect concrete cracks that rely on operators lack objectivity and consume a lot of time. To overcome this limitation, high-resolution image processing and deep learning have been adopted. Nevertheless, cracks on structure surfaces are still challenging to detect owing to the variety of shapes of cracks and the dependence of recognition performance on image conditions. Herein, we propose a new concrete crack detection method that applies the semantic segmentation technique using 1196 concrete crack images and labeled images produced in this study. A new segmentation algorithm is developed using a hierarchical convolutional neural network to improve speed, and a multi-loss update method is proposed to improve accuracy. The performance of the proposed network is evaluated in terms of accuracy and speed. The results show that the proposed network produces a 2.165% increase in the intersection over union of crack, 65.90% decrease in the average inference time, and 99.90% decrease in the number of parameters compared with the best accuracy results using existing segmentation networks. It is expected that the application of this improved crack detection method will result in faster and more accurate crack detection and, consequently, improved safety, thereby making it suitable for application in structure safety inspections.
更多查看译文
关键词
crack detection, deep learning, infrastructure maintenance, semantic segmentation
AI 理解论文
溯源树
样例
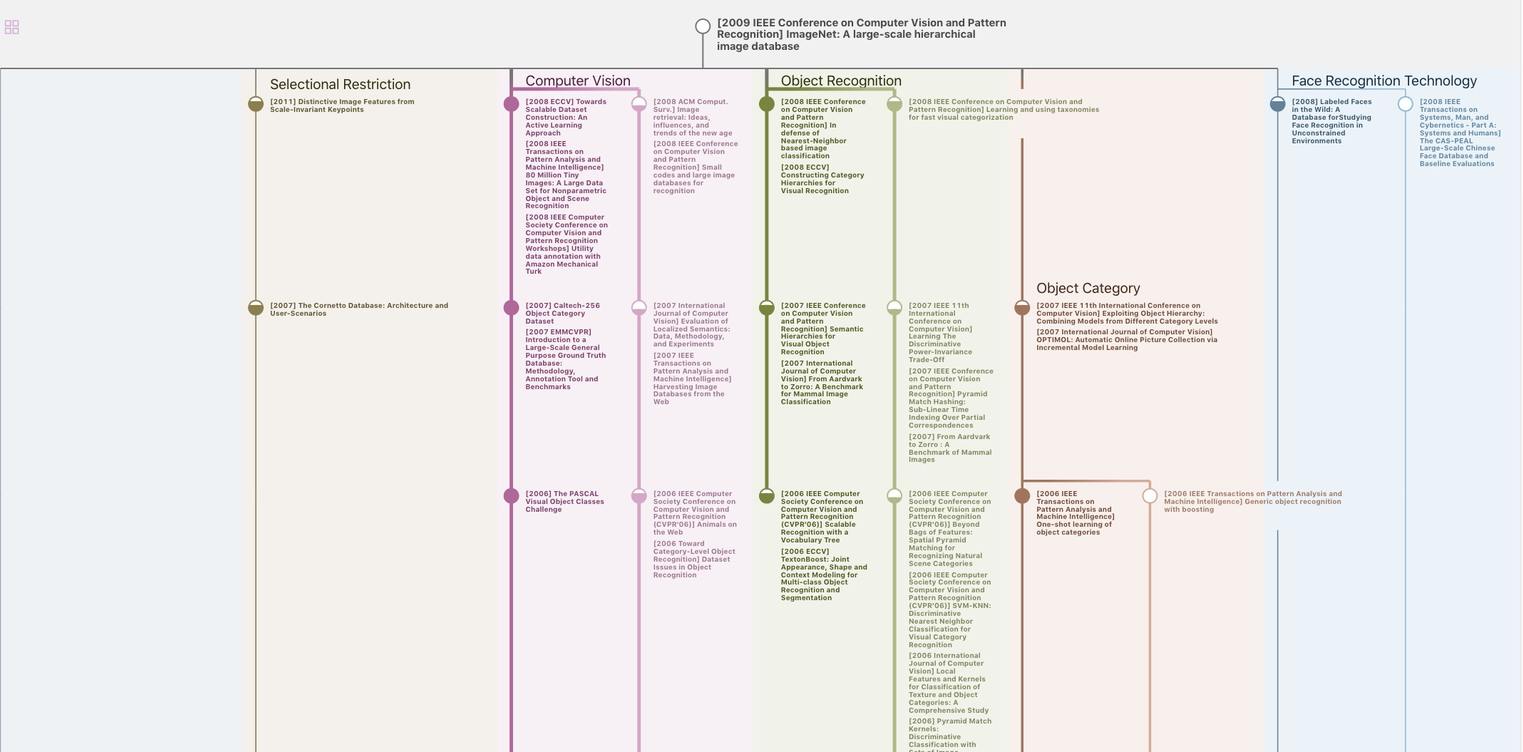
生成溯源树,研究论文发展脉络
Chat Paper
正在生成论文摘要