Deep Mutual Information Maximin For Cross-Modal Clustering
THIRTY-FIFTH AAAI CONFERENCE ON ARTIFICIAL INTELLIGENCE, THIRTY-THIRD CONFERENCE ON INNOVATIVE APPLICATIONS OF ARTIFICIAL INTELLIGENCE AND THE ELEVENTH SYMPOSIUM ON EDUCATIONAL ADVANCES IN ARTIFICIAL INTELLIGENCE(2021)
摘要
Cross-modal clustering (CMC) aims to enhance the clustering performance by exploring complementary information from multiple modalities. However, the performances of existing CMC algorithms are still unsatisfactory due to the conflict of heterogeneous modalities and the high-dimensional non-linear property of individual modality. In this paper, a novel deep mutual information maximin (DMIM) method for cross-modal clustering is proposed to maximally preserve the shared information of multiple modalities while eliminating the superfluous information of individual modalities in an end-to-end manner. Specifically, a multi-modal shared encoder is firstly built to align the latent feature distributions by sharing parameters across modalities. Then, DMIM formulates the complementarity of multi-modalities representations as a mutual information maximin objective function, in which the shared information of multiple modalities and the superfluous information of individual modalities are identified by mutual information maximization and minimization respectively. To solve the DMIM objective function, we propose a variational optimization method to ensure it converge to a local optimal solution. Moreover, an auxiliary overclustering mechanism is employed to optimize the clustering structure by introducing more detailed clustering classes. Extensive experimental results demonstrate the superiority of DMIM method over the state-of-the-art cross-modal clustering methods on IAPR-TC12, ESP-Game, MIRFlickr and NUSWide datasets.
更多查看译文
关键词
clustering,information,cross-modal
AI 理解论文
溯源树
样例
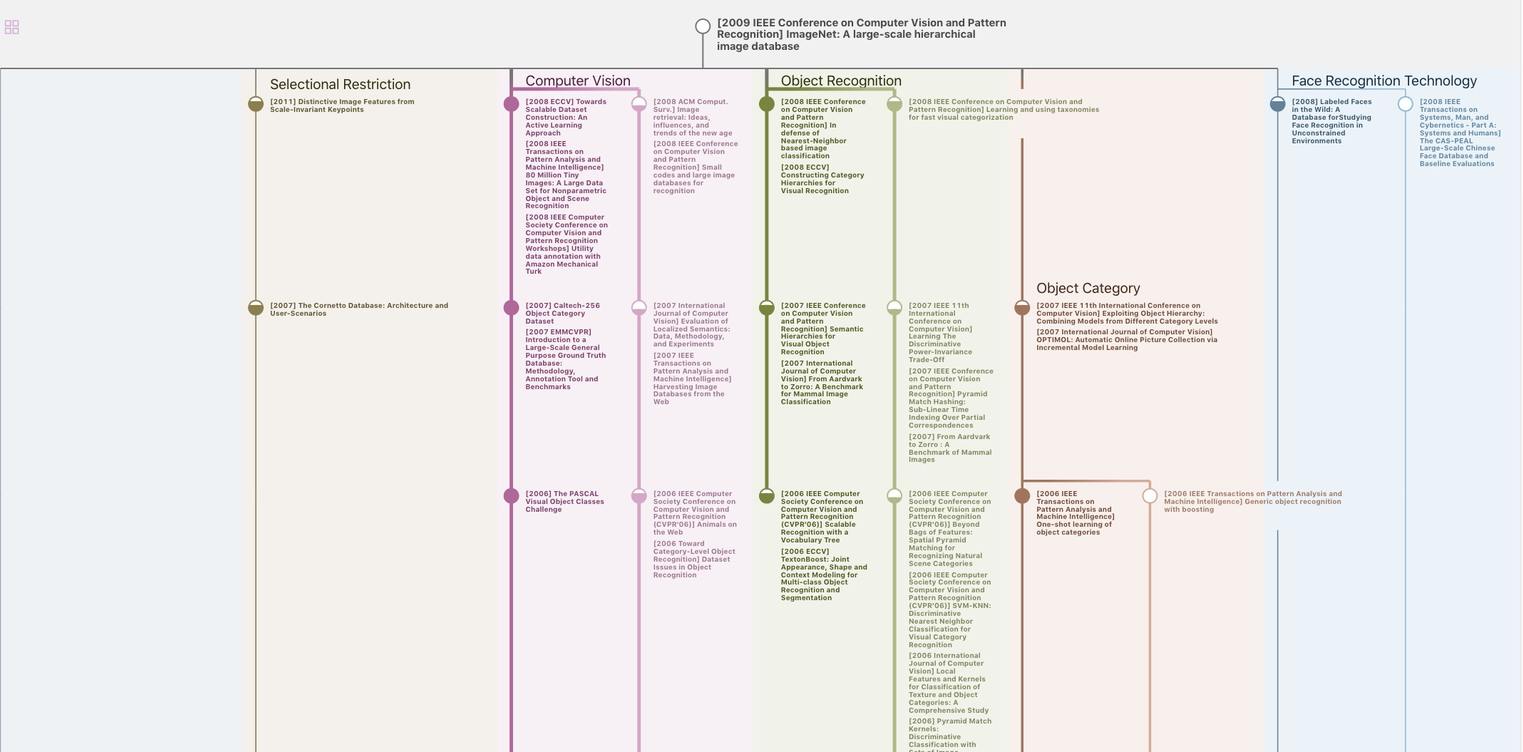
生成溯源树,研究论文发展脉络
Chat Paper
正在生成论文摘要