A semigroup method for high dimensional elliptic PDEs and eigenvalue problems based on neural networks
Journal of Computational Physics(2022)
摘要
In this paper, we propose a semigroup method for solving high-dimensional elliptic partial differential equations (PDEs) and the associated eigenvalue problems based on neural networks. For the PDE problems, we reformulate the original equations as variational problems with the help of semigroup operators and then solve the variational problems with neural network (NN) parameterization. The main advantages are that no mixed second-order derivative computation is needed during the stochastic gradient descent training and that the boundary conditions are taken into account automatically by the semigroup operator. Unlike popular methods like PINN [16] and Deep Ritz [5] where the Dirichlet boundary condition is enforced solely through penalty functions and thus changes the true solution, the proposed method is able to address the boundary conditions without penalty functions and it gives the correct true solution even when penalty functions are added, thanks to the semigroup operator. For eigenvalue problems, a primal-dual method is proposed, efficiently resolving the constraint with a simple scalar dual variable and resulting in a faster algorithm compared with the BSDE solver [8] in certain problems such as the eigenvalue problem associated with the linear Schrödinger operator. Numerical results are provided to demonstrate the performance of the proposed methods.
更多查看译文
关键词
Partial differential equation,Eigenvalue problem,Neural network,Semigroup method
AI 理解论文
溯源树
样例
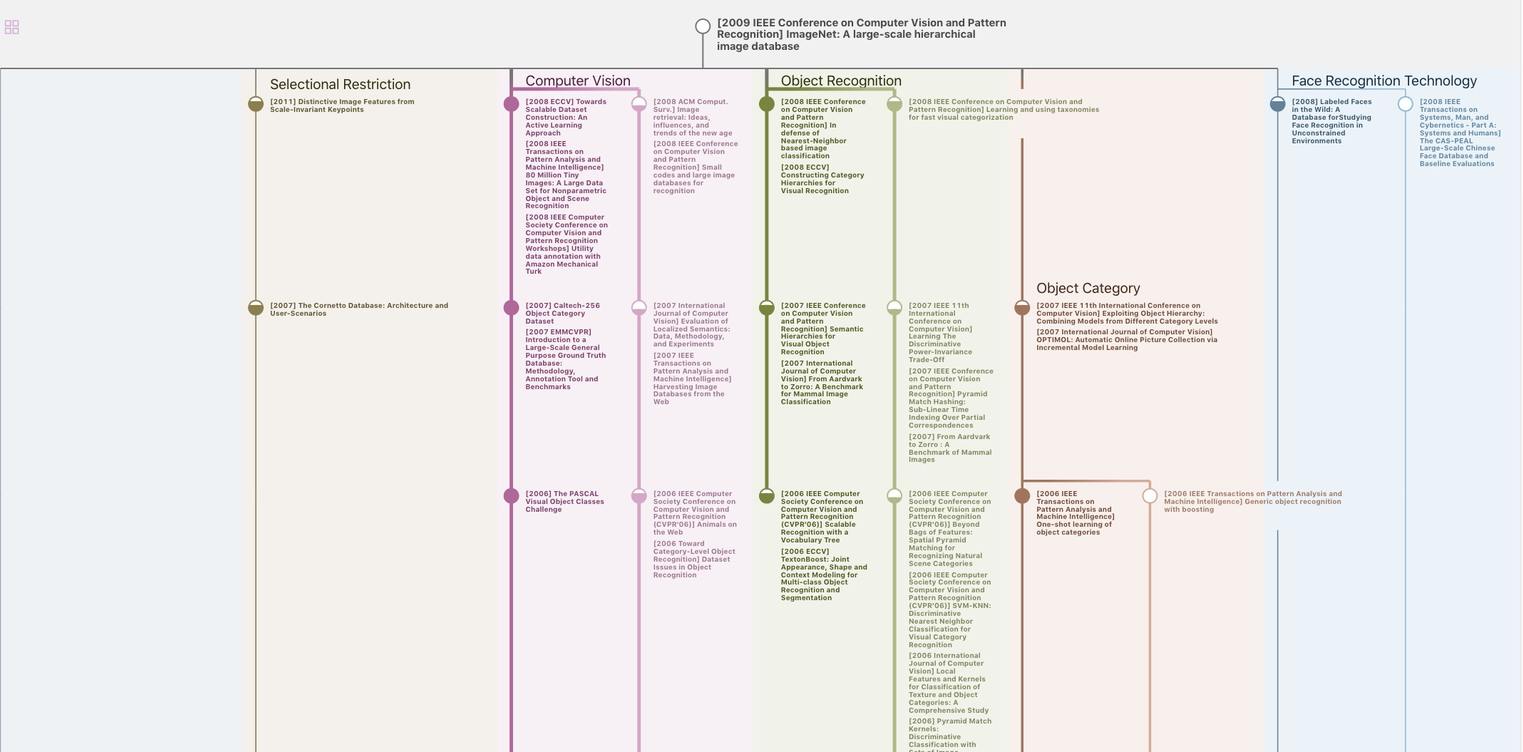
生成溯源树,研究论文发展脉络
Chat Paper
正在生成论文摘要