FedGL: Federated graph learning framework with global self-supervision
INFORMATION SCIENCES(2024)
摘要
Graph data are ubiquitous in the real world. Graph learning (GL) attempts to mine and analyze graph data so that valuable information can be discovered. Existing GL methods are designed for centralized scenarios. However, in practical scenarios, graph data are usually distributed across different organizations, resulting in isolated data silos. To address this problem, we incorporate federated learning into GL and propose a general Federated Graph Learning framework called FedGL. FedGL is capable of obtaining a high-quality global graph model while protecting data privacy by discovering the global self-supervision information during the federated training. Specifically, we propose uploading prediction results and node embeddings to the server for discovering the global pseudo labels and global pseudo graph. These are then distributed to each client to enrich the training labels and complement the graph structure respectively, thereby improving the quality of each local model. Moreover, the global self-supervision enables information of each client to flow and be shared in a privacy-preserving manner, thus alleviating the heterogeneity and utilizing the complementarity of graph data among different clients. Finally, experimental results show that FedGL significantly outperforms baselines on four widely used graph datasets.
更多查看译文
关键词
Federated learning,Graph learning,Graph neural networks,Self-supervised learning
AI 理解论文
溯源树
样例
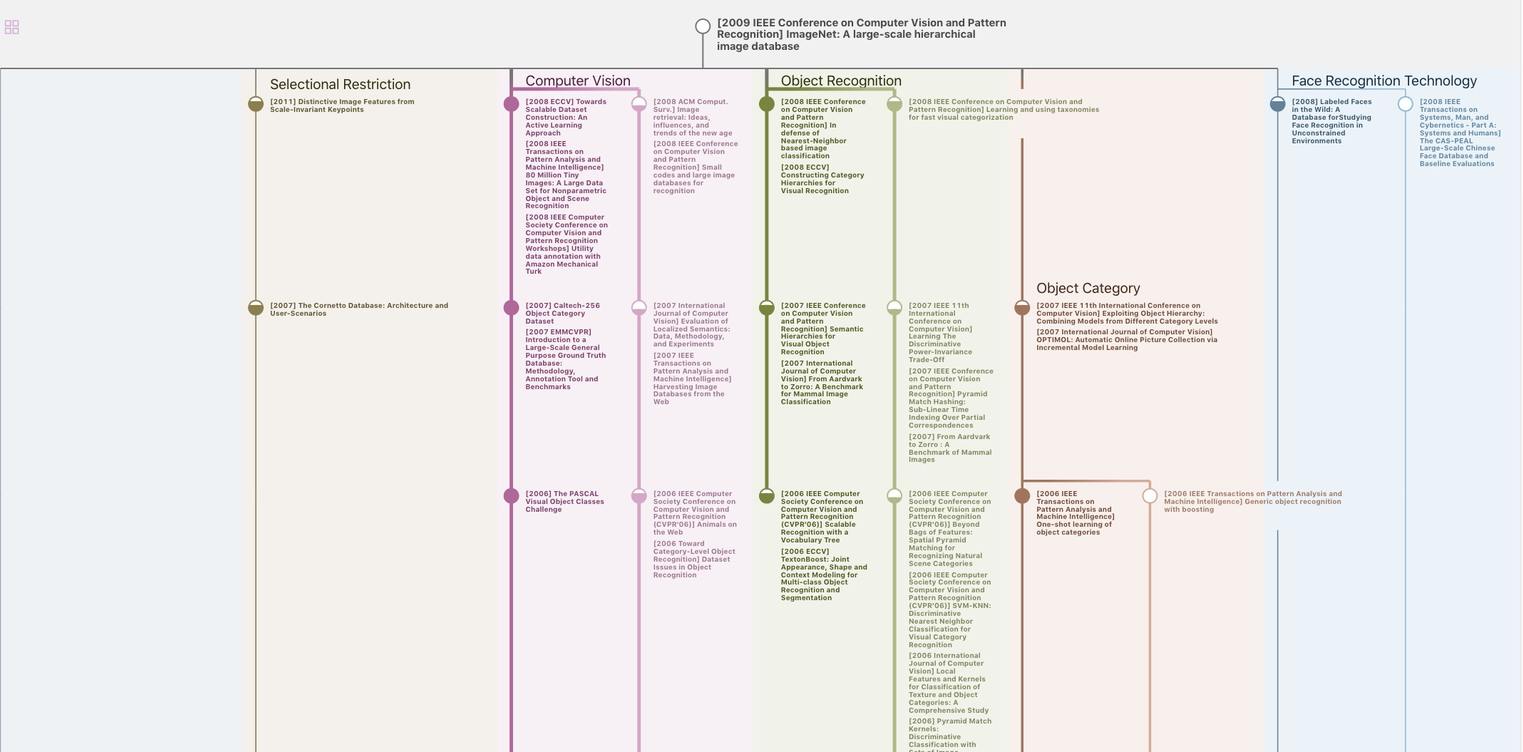
生成溯源树,研究论文发展脉络
Chat Paper
正在生成论文摘要