Embedding Semantic Hierarchy in Discrete Optimal Transport for Risk Minimization.
2021 IEEE INTERNATIONAL CONFERENCE ON ACOUSTICS, SPEECH AND SIGNAL PROCESSING (ICASSP 2021)(2021)
Abstract
The widely-used cross-entropy (CE) loss-based deep networks achieved significant progress w.r.t. the classification accuracy. However, the CE loss can essentially ignore the risk of misclassification which is usually measured by the distance between the prediction and label in a semantic hierarchical tree. In this paper, we propose to incorporate the risk-aware inter-class correlation in a discrete optimal transport (DOT) training framework by configuring its ground distance matrix. The ground distance matrix can be pre-defined following a priori of hierarchical semantic risk. Specifically, we define the tree induced error (TIE) on a hierarchical semantic tree and extend it to its increasing function from the optimization perspective. The semantic similarity in each level of a tree is integrated with the information gain. We achieve promising results on several large scale image classification tasks with a semantic tree structure in a plug and play manner.
MoreTranslated text
Key words
Discrete optimal transport,Tree induced error,Semantic hierarchical tree,Loss function
AI Read Science
Must-Reading Tree
Example
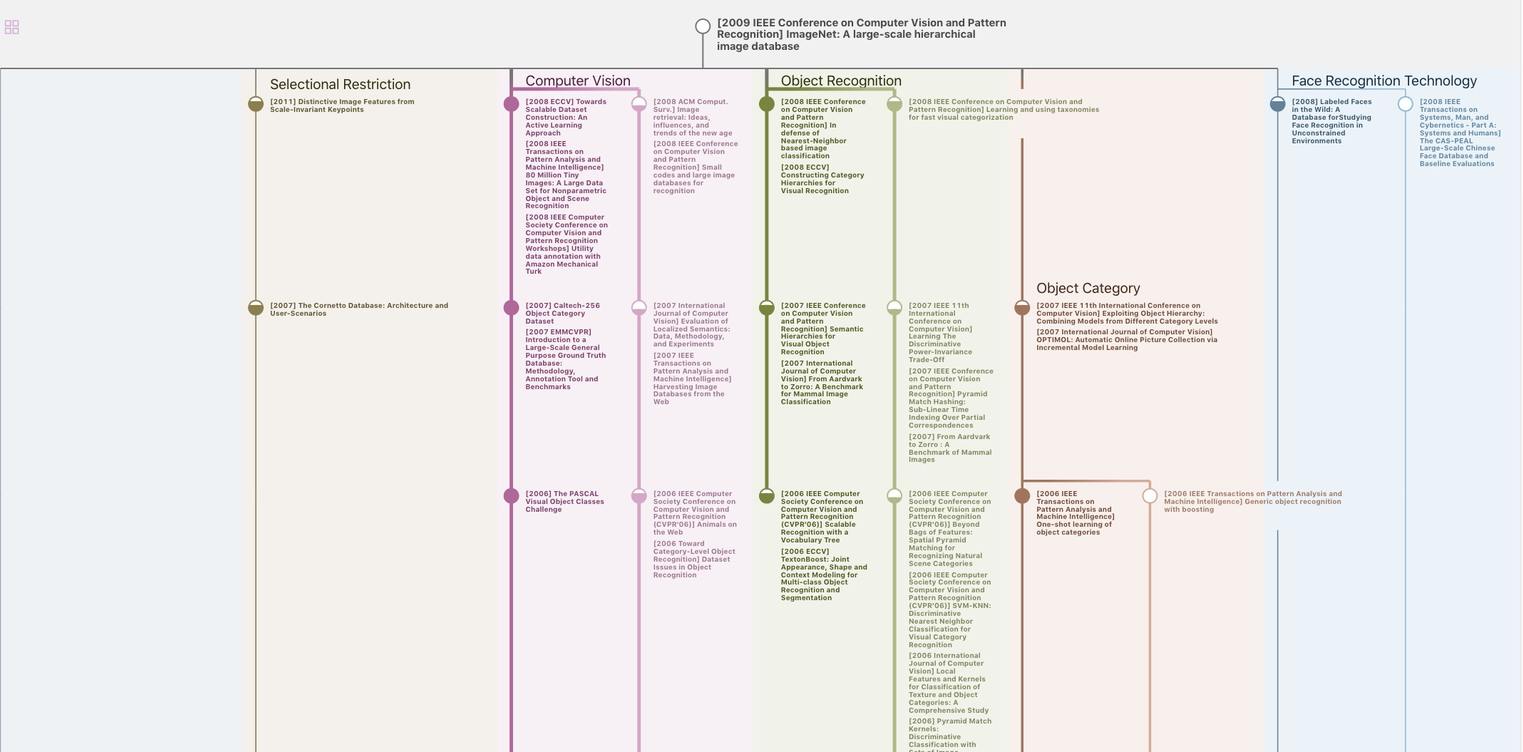
Generate MRT to find the research sequence of this paper
Chat Paper
Summary is being generated by the instructions you defined