SINr: Fast Computing of Sparse Interpretable Node Representations is Not a Sin!
ADVANCES IN INTELLIGENT DATA ANALYSIS XIX, IDA 2021(2021)
摘要
While graph embedding aims at learning low-dimensional representations of nodes encompassing the graph topology, word embedding focus on learning word vectors that encode semantic properties of the vocabulary. The first finds applications on tasks such as link prediction and node classification while the latter is systematically considered in natural language processing. Most of the time, graph and word embeddings are considered on their own as distinct tasks. However, word co-occurrence matrices, widely used to extract word embeddings, can be seen as graphs. Furthermore, most network embedding techniques rely either on a word embedding methodology (Word2vec) or on matrix factorization, also widely used for word embedding. These methods are usually computationally expensive, parameter dependant and the dimensions of the embedding space are not interpretable. To circumvent these issues, we introduce the Lower Dimension Bipartite Graphs Framework (LDBGF) which takes advantage of the fact that all graphs can be described as bipartite graphs, even in the case of textual data. This underlying bipartite structure may be explicit, like in coauthor networks. However, with LDBGF, we focus on uncovering latent bipartite structures, lying for instance in social or word co-occurrence networks, and especially such structures providing conciser and interpretable representations of the graph at hand. We further propose SINr, an efficient implementation of the LDBGF approach that extracts Sparse Interpretable Node Representations using community structure to approximate the underlying bipartite structure. In the case of graph embedding, our near-linear time method is the fastest of our benchmark, parameter-free and provides state-of-the-art results on the classical link prediction task. We also show that low-dimensional vectors can be derived from SINr using singular value decomposition. In the case of word embedding, our approach proves to be very efficient considering the classical similarity evaluation.
更多查看译文
AI 理解论文
溯源树
样例
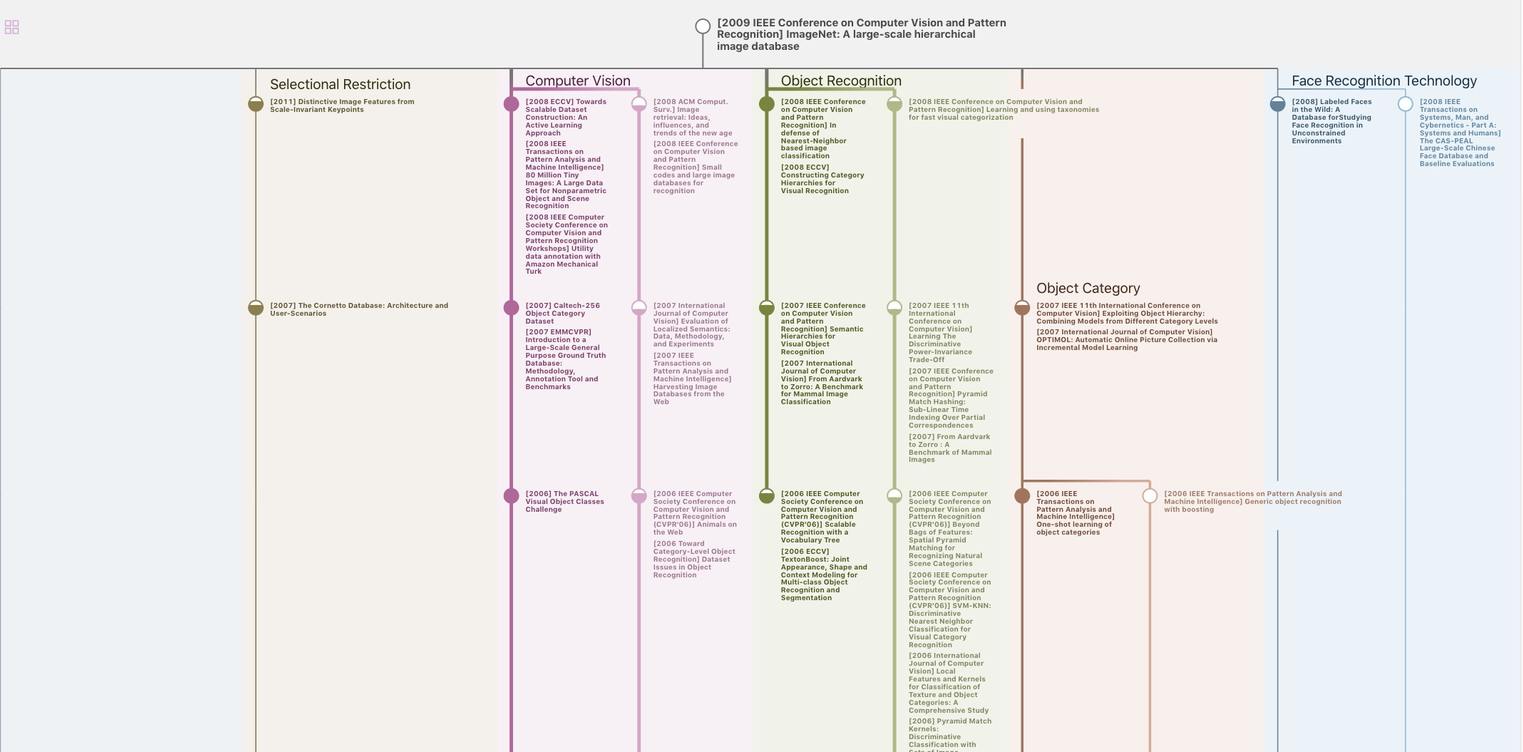
生成溯源树,研究论文发展脉络
Chat Paper
正在生成论文摘要