Risk of Cardiac Implantable Electronic Device Malfunctioning During Pencil Beam Proton Scanning in an in Vitro Setting.
International Journal of Radiation OncologyBiologyPhysics(2021)
AI Read Science
Must-Reading Tree
Example
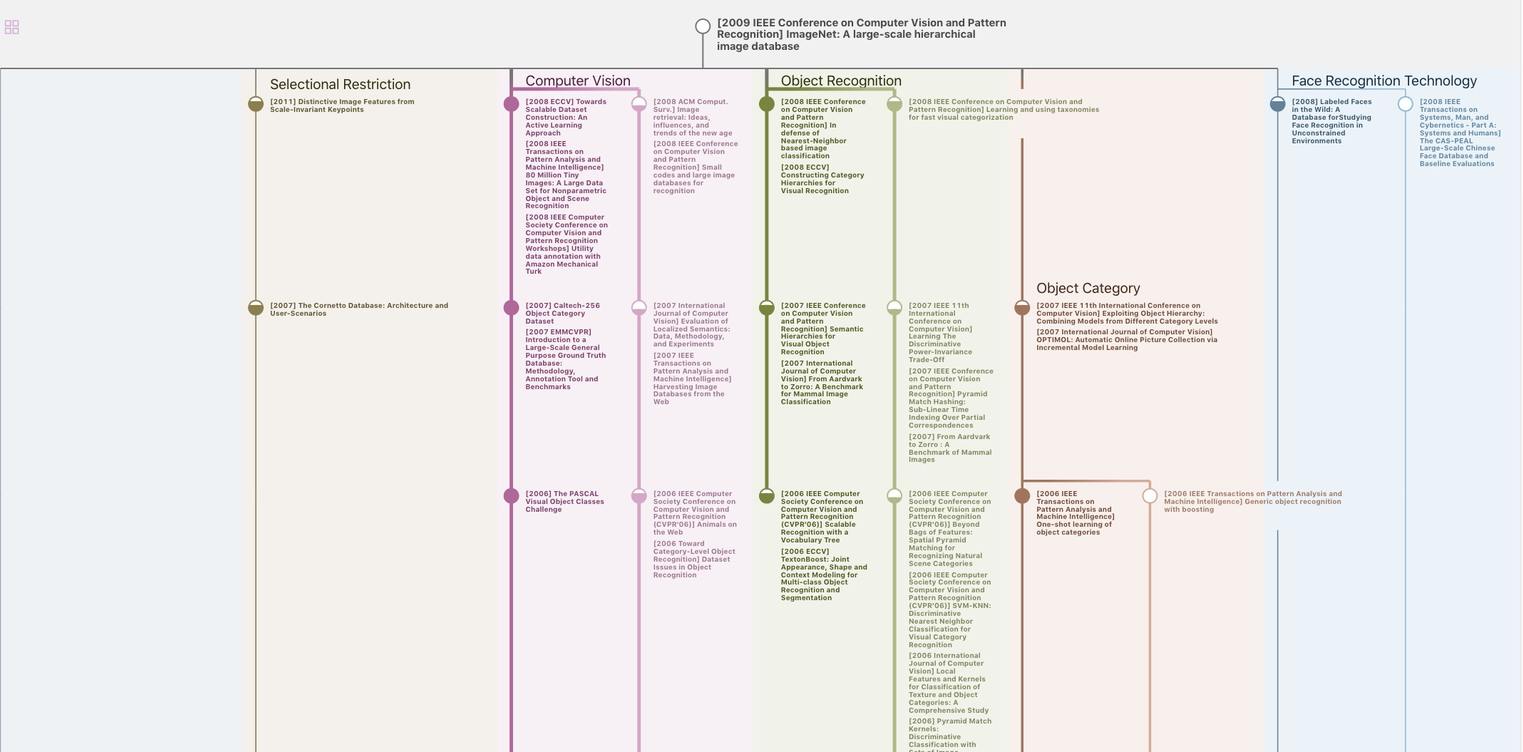
Generate MRT to find the research sequence of this paper
Chat Paper
Summary is being generated by the instructions you defined