Sparse NMF with Archetypal Regularization: Computational and Robustness Properties
JOURNAL OF MACHINE LEARNING RESEARCH(2024)
摘要
We consider the problem of sparse nonnegative matrix factorization (NMF) using archetypal regularization. The goal is to represent a collection of data points as nonnegative linear combinations of a few nonnegative sparse factors with appealing geometric properties, arising from the use of archetypal regularization. We generalize the notion of robustness studied in Javadi and Montanari (2019) (without sparsity) to the notions of (a) strong robustness that implies each estimated archetype is close to the underlying archetypes and (b) weak robustness that implies there exists at least one recovered archetype that is close to the underlying archetypes. Our theoretical results on robustness guarantees hold under minimal assumptions on the underlying data, and applies to settings where the underlying archetypes need not be sparse. We present theoretical results and illustrative examples to strengthen the insights underlying the notions of robustness. We propose new algorithms for our optimization problem; and present numerical experiments on synthetic and real data sets that shed further insights into our proposed framework and theoretical developments.
更多查看译文
关键词
Sparse Nonnegative Matrix Factorization,Archetypal Analysis,Robustness to Perturbation,Model misspecification,Nonconvex Optimization
AI 理解论文
溯源树
样例
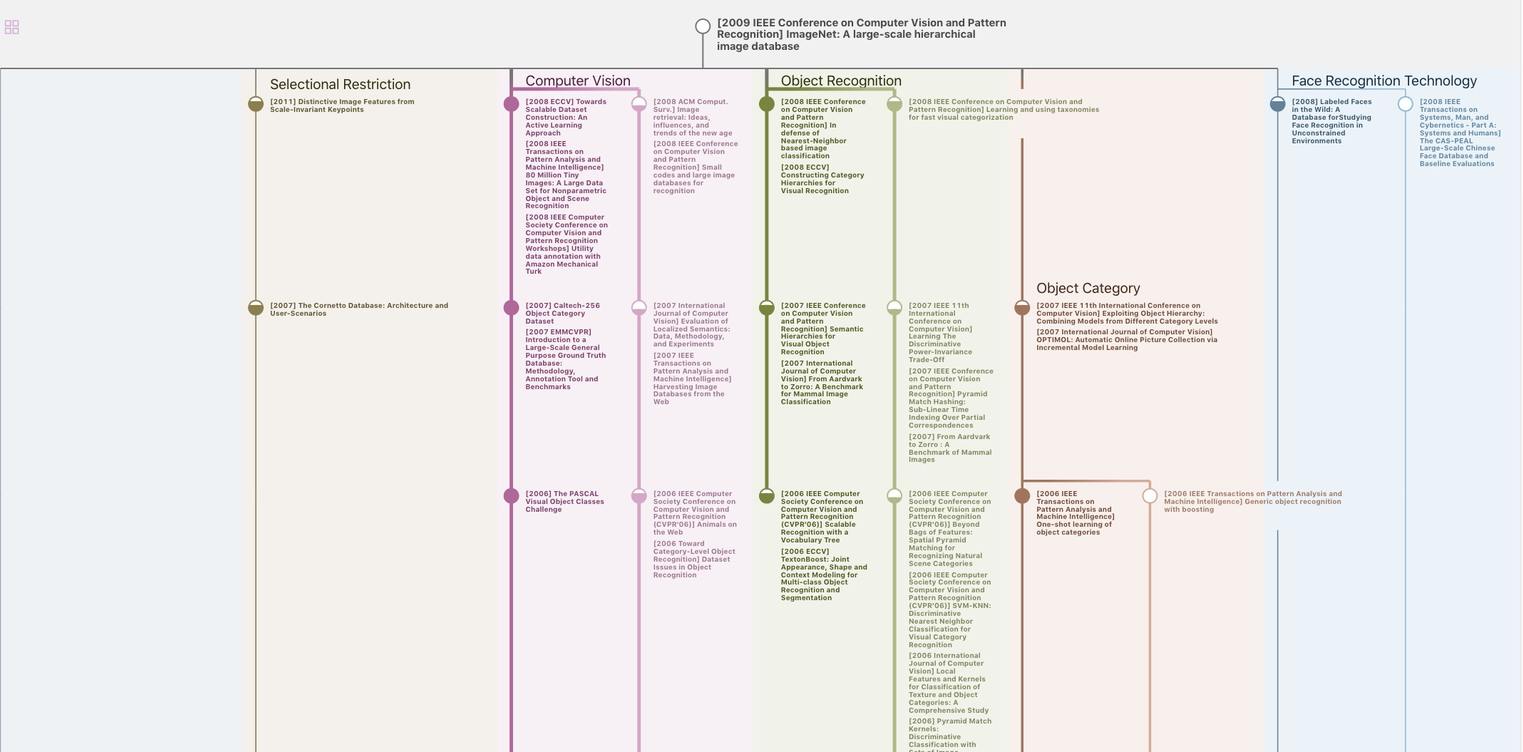
生成溯源树,研究论文发展脉络
Chat Paper
正在生成论文摘要