Potential Convolution: Embedding Point Clouds into Potential Fields
CoRR(2021)
摘要
Recently, various convolutions based on continuous or discrete kernels for point cloud processing have been widely studied, and achieve impressive performance in many applications, such as shape classification, scene segmentation and so on. However, they still suffer from some drawbacks. For continuous kernels, the inaccurate estimation of the kernel weights constitutes a bottleneck for further improving the performance; while for discrete ones, the kernels represented as the points located in the 3D space are lack of rich geometry information. In this work, rather than defining a continuous or discrete kernel, we directly embed convolutional kernels into the learnable potential fields, giving rise to potential convolution. It is convenient for us to define various potential functions for potential convolution which can generalize well to a wide range of tasks. Specifically, we provide two simple yet effective potential functions via point-wise convolution operations. Comprehensive experiments demonstrate the effectiveness of our method, which achieves superior performance on the popular 3D shape classification and scene segmentation benchmarks compared with other state-of-the-art point convolution methods.
更多查看译文
关键词
potential fields,potential convolution,point clouds
AI 理解论文
溯源树
样例
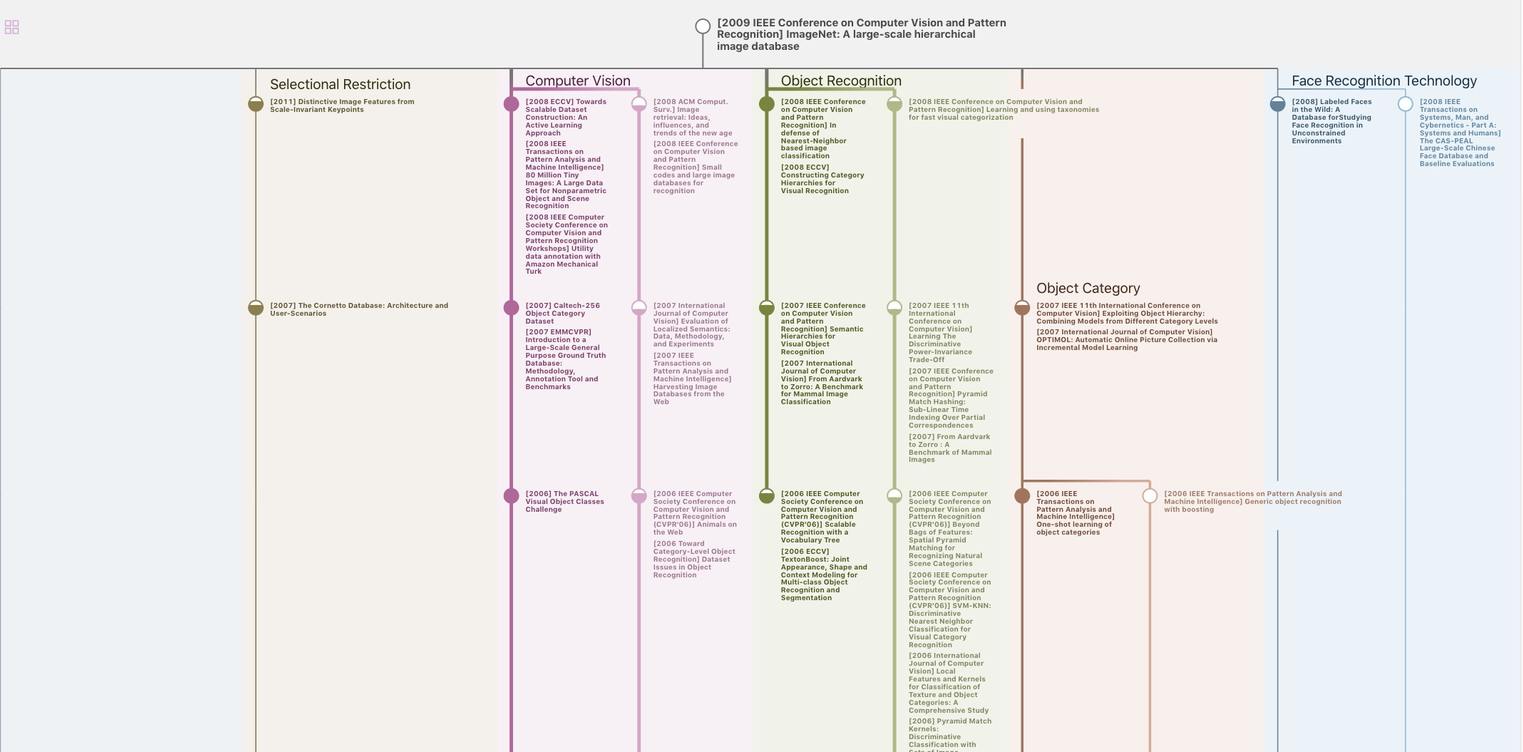
生成溯源树,研究论文发展脉络
Chat Paper
正在生成论文摘要