Reservoir-Based Distributed Machine Learning for Edge Operation
MILCOM 2021 - 2021 IEEE Military Communications Conference (MILCOM)(2021)
摘要
We introduce a novel design for in-situ training of machine learning algorithms built into smart sensors, and illustrate distributed training scenarios using radio frequency (RF) spectrum sensors. Current RF sensors at the Edge lack the computational resources to support practical, in-situ training for intelligent signal classification. We propose a solution using Deepdelay Loop Reservoir Computing (DLR), a processing architecture that supports machine learning algorithms on resource-constrained edge-devices by leveraging delayloop reservoir computing in combination with innovative hardware. DLR delivers reductions in form factor, hardware complexity and latency, compared to the State-ofthe- Art (SoA) neural nets. We demonstrate DLR for two applications: RF Specific Emitter Identification (SEI) and wireless protocol recognition. DLR enables mobile edge platforms to authenticate and then track emitters with fast SEI retraining. Once delay loops separate the data classes, traditionally complex, power-hungry classification models are no longer needed for the learning process. Yet, even with simple classifiers such as Ridge Regression (RR), the complexity grows at least quadratically with the input size. DLR with a RR classifier exceeds the SoA accuracy, while further reducing power consumption by leveraging the architecture of parallel (split) loops. To authenticate mobile devices across large regions, DLR can be trained in a distributed fashion with very little additional processing and a small communication cost, all while maintaining accuracy. We illustrate how to merge locally trained DLR classifiers in use cases of interest.
更多查看译文
关键词
Training,Image edge detection,Time series analysis,Neural networks,Transfer learning,Authentication,Reservoirs
AI 理解论文
溯源树
样例
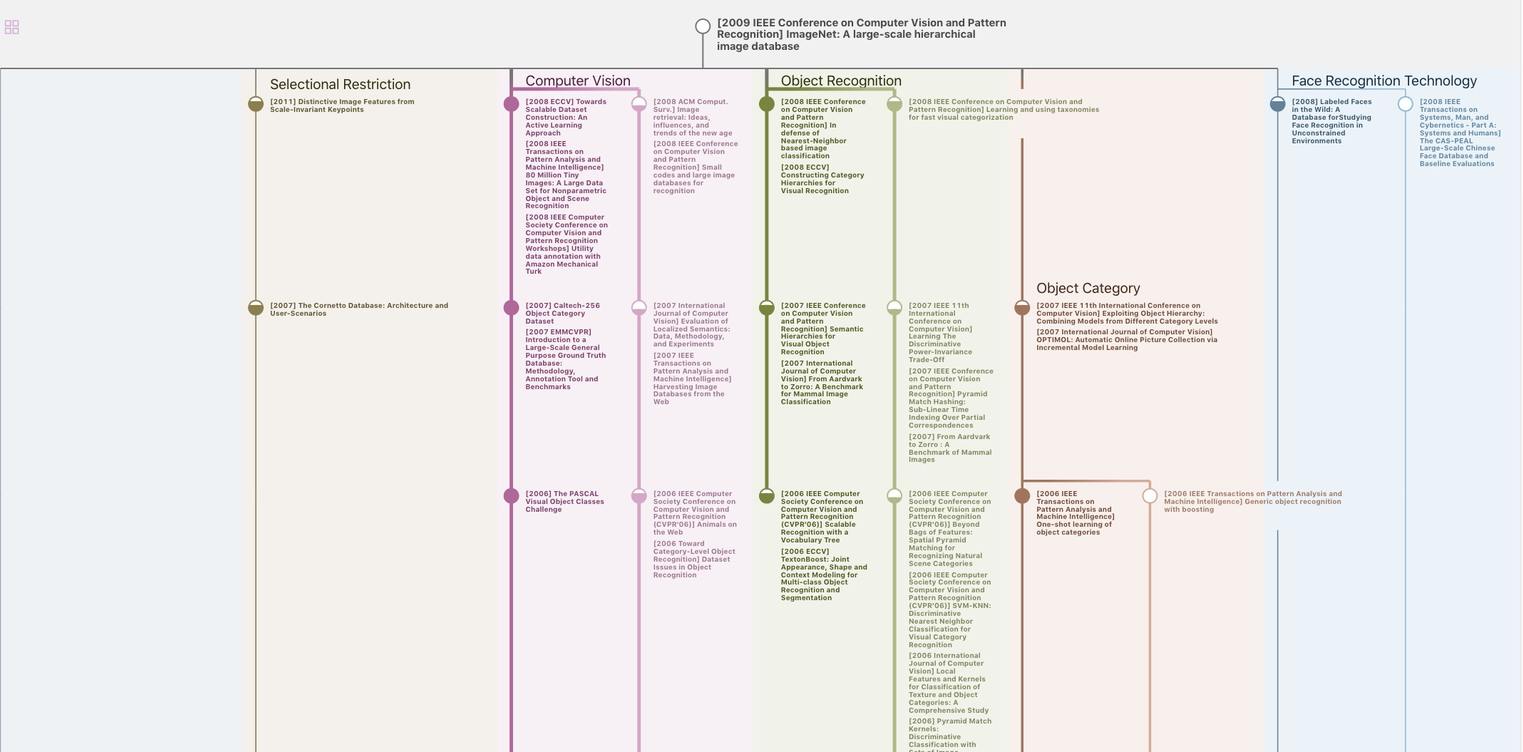
生成溯源树,研究论文发展脉络
Chat Paper
正在生成论文摘要