DDR-Net: Learning Multi-Stage Multi-View Stereo with Dynamic Depth Range
arXiv (Cornell University)(2021)
摘要
To obtain high-resolution depth maps, some previous learning-based multi-view stereo methods build a cost volume pyramid in a coarse-to-fine manner. These approaches leverage fixed depth range hypotheses to construct cascaded plane sweep volumes. However, it is inappropriate to set identical range hypotheses for each pixel since the uncertainties of previous per-pixel depth predictions are spatially varying. Distinct from these approaches, we propose a Dynamic Depth Range Network (DDR-Net) to determine the depth range hypotheses dynamically by applying a range estimation module (REM) to learn the uncertainties of range hypotheses in the former stages. Specifically, in our DDR-Net, we first build an initial depth map at the coarsest resolution of an image across the entire depth range. Then the range estimation module (REM) leverages the probability distribution information of the initial depth to estimate the depth range hypotheses dynamically for the following stages. Moreover, we develop a novel loss strategy, which utilizes learned dynamic depth ranges to generate refined depth maps, to keep the ground truth value of each pixel covered in the range hypotheses of the next stage. Extensive experimental results show that our method achieves superior performance over other state-of-the-art methods on the DTU benchmark and obtains comparable results on the Tanks and Temples benchmark. The code is available at https://github.com/Tangshengku/DDR-Net.
更多查看译文
关键词
Depth Estimation,Multi-View Stereo,Monocular Depth Estimation,Stereo Vision
AI 理解论文
溯源树
样例
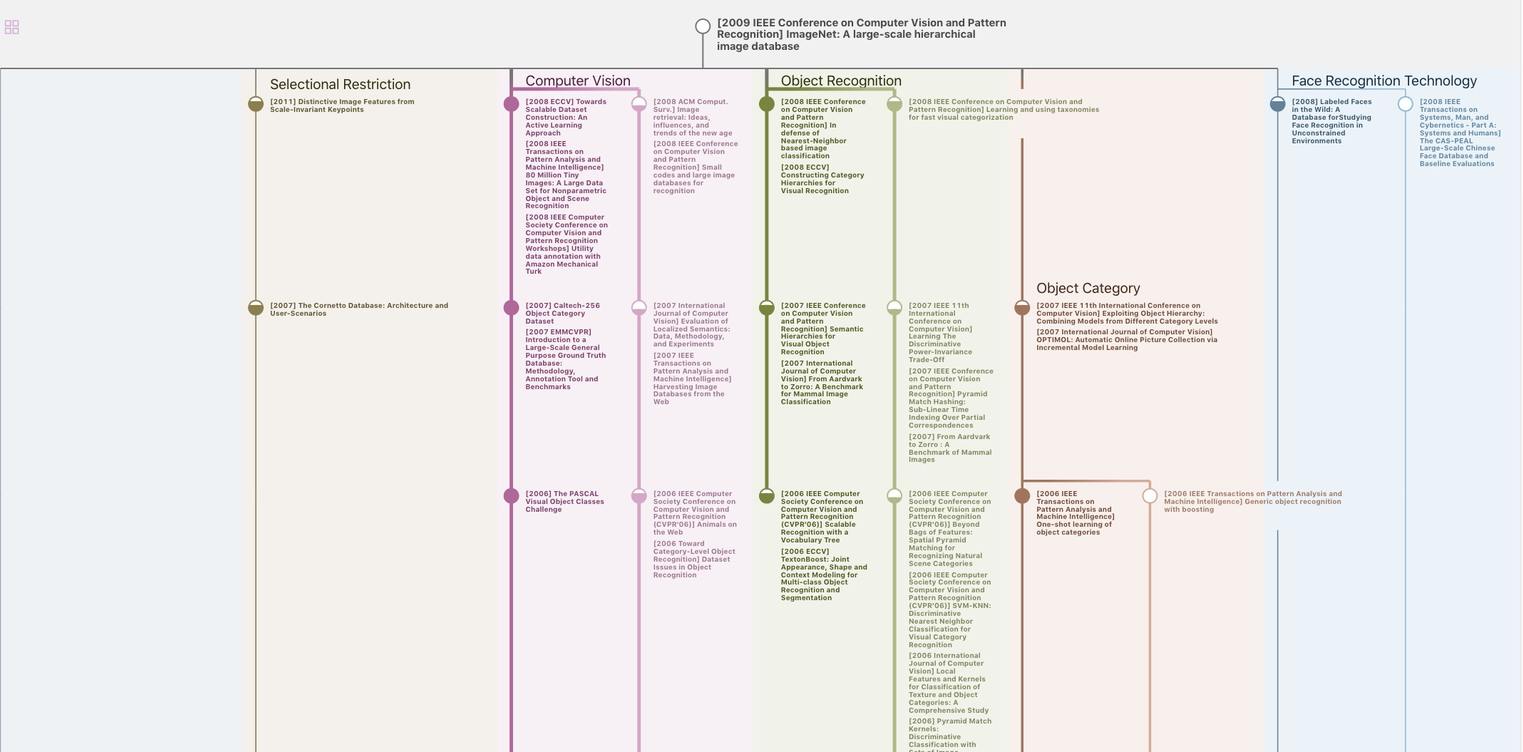
生成溯源树,研究论文发展脉络
Chat Paper
正在生成论文摘要