A Comparison of FIML- versus Multiple-imputation-based methods to test measurement invariance with incomplete ordinal variables
STRUCTURAL EQUATION MODELING-A MULTIDISCIPLINARY JOURNAL(2021)
摘要
To ensure meaningful comparison of test scores across groups or time, measurement invariance (i.e., invariance of the general factor structure and the values of the measurement parameters) across groups or time must be examined. However, many empirical examinations of measurement invariance of psychological/educational questionnaires need to address two issues: Using the appropriate model for ordinal variables (e.g., Likert scale items), and handling missing data. In two Monte Carlo simulations, this study examined the performance of one full-information-maximum-likelihood-based method and five multiple-imputation-based methods to obtain tests of measurement invariance across groups for ordinal variables that have missing data. Our results indicate that the full-information-maximum-likelihood-based method and one of the multiple-imputation-based methods generally have better performance than the other examined methods, though they also have their own limitations.
更多查看译文
关键词
Missing data,ordinal data,measurement invariance,full information maximum likelihood,multiple imputation
AI 理解论文
溯源树
样例
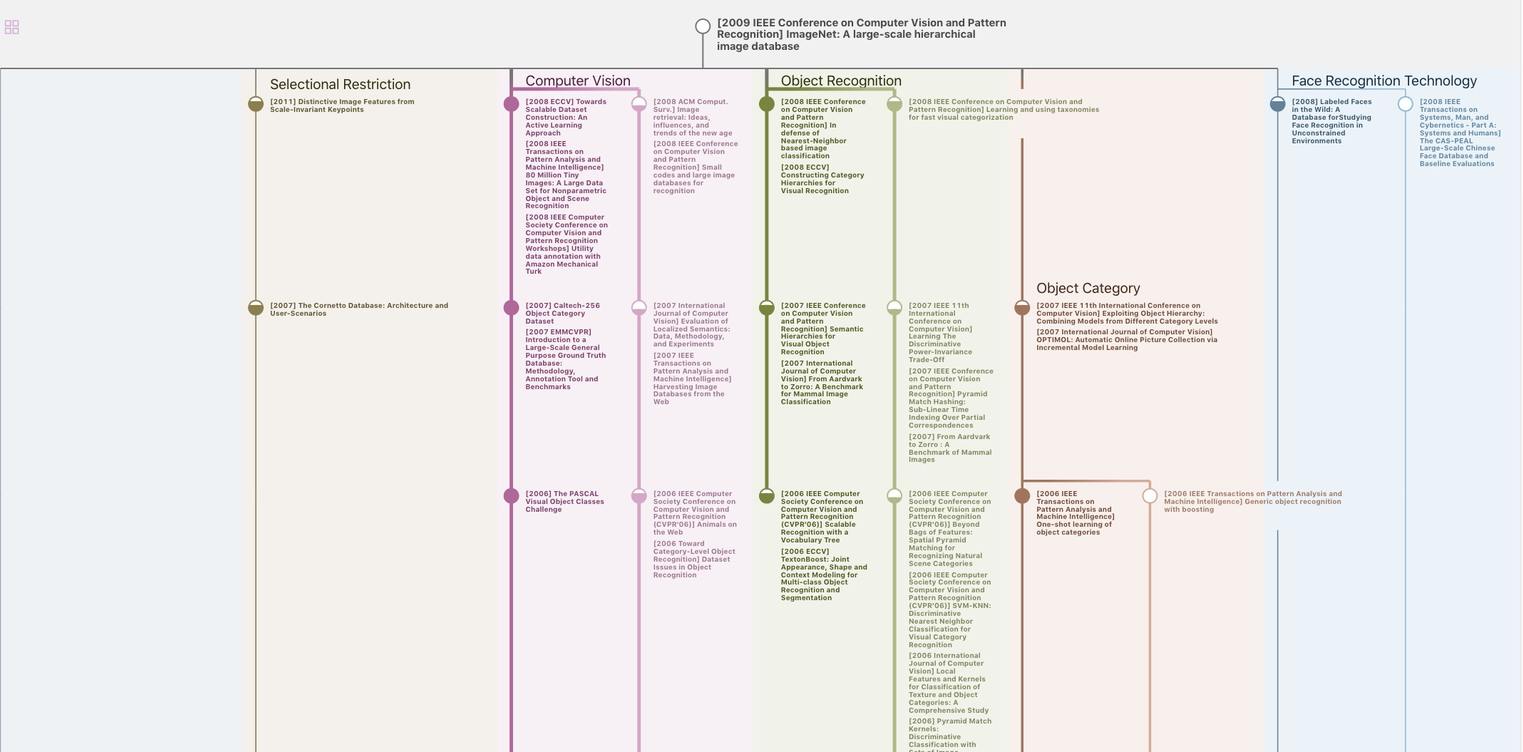
生成溯源树,研究论文发展脉络
Chat Paper
正在生成论文摘要