Switched Iterative Learning Attitude and Structural Control for Solar Power Satellites
Acta Astronautica(2021)SCI 3区SCI 2区
Sun Yat Sen Univ | Jining Med Univ | Northwestern Polytech Univ
Abstract
A switched iterative learning controller (ILC) is proposed for a flexible solar power satellite (SPS) to periodically track the earth (transmitting antenna) and the sun (solar array). ILC is advantageous to deal with batch processes because the feedforward control signal learns from the inputs and errors of previous operations. However, the main difficulty applying ILC to attitude control of the SPS is that the periodic attitude motions are continuous processes instead of batch processes. The ILC would cause sudden change of control inputs and severe oscillation of control errors when the feedforward signals are suddenly added into the controller. To address this problem, a smooth switch is designed to avoid altering the control inputs abruptly. Furthermore, a filter is adopted based on Fourier series to eliminate the adverse influence of useless signals from the previous periods, such as sensor noise and structural vibrations. It is found from numerical simulations that the proposed switched ILC improves the attitude control accuracies by one to two orders of magnitude. Moreover, it reduces the interaction between attitude of the antenna and the structural vibrations of the truss.
MoreTranslated text
Key words
Iterative learning control,Attitude control,Structural control,Solar power satellite,Flexible spacecraft
PDF
View via Publisher
AI Read Science
AI Summary
AI Summary is the key point extracted automatically understanding the full text of the paper, including the background, methods, results, conclusions, icons and other key content, so that you can get the outline of the paper at a glance.
Example
Background
Key content
Introduction
Methods
Results
Related work
Fund
Key content
- Pretraining has recently greatly promoted the development of natural language processing (NLP)
- We show that M6 outperforms the baselines in multimodal downstream tasks, and the large M6 with 10 parameters can reach a better performance
- We propose a method called M6 that is able to process information of multiple modalities and perform both single-modal and cross-modal understanding and generation
- The model is scaled to large model with 10 billion parameters with sophisticated deployment, and the 10 -parameter M6-large is the largest pretrained model in Chinese
- Experimental results show that our proposed M6 outperforms the baseline in a number of downstream tasks concerning both single modality and multiple modalities We will continue the pretraining of extremely large models by increasing data to explore the limit of its performance
Try using models to generate summary,it takes about 60s
Must-Reading Tree
Example
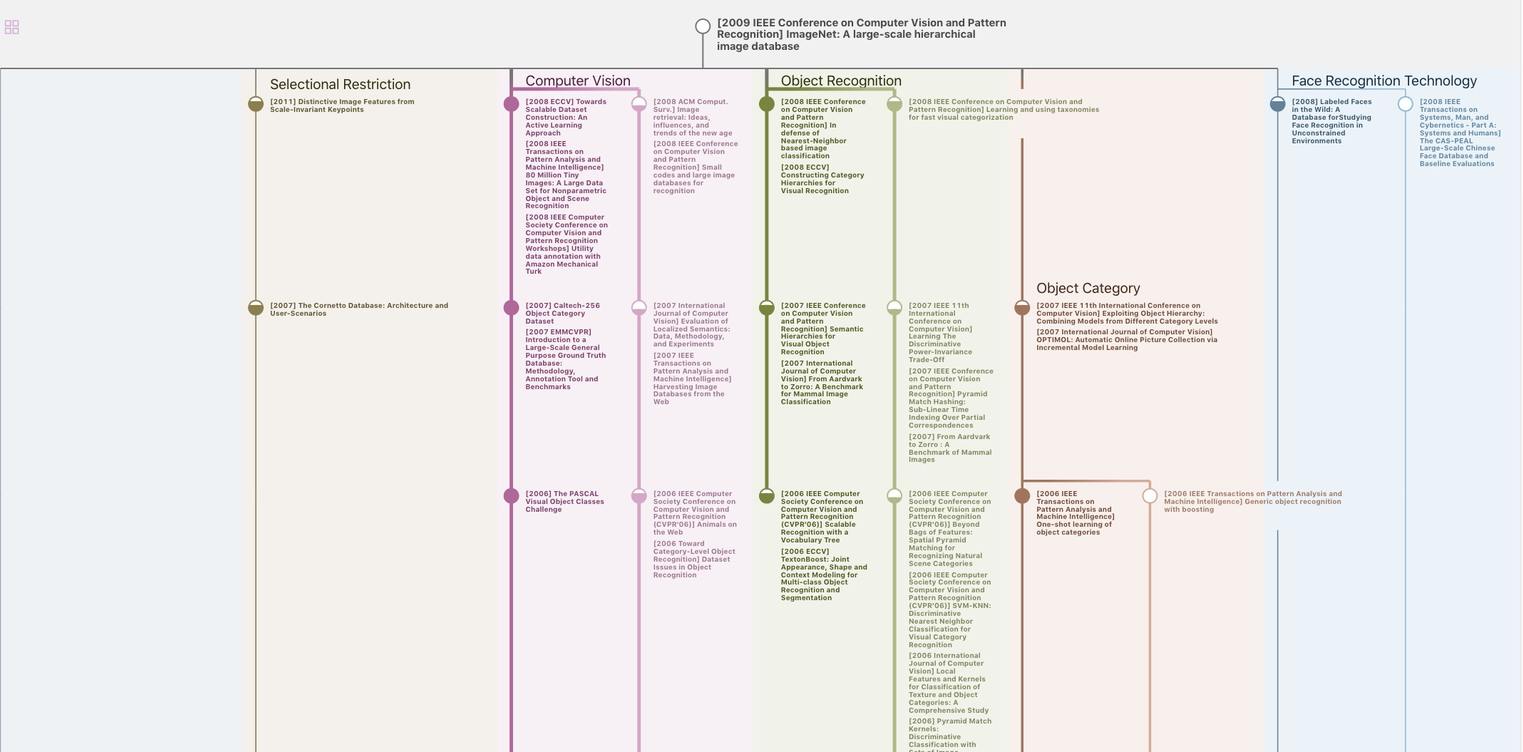
Generate MRT to find the research sequence of this paper
Related Papers
Adaptive Fuzzy Fault-Tolerant Control for the Attitude Tracking of Spacecraft Within Finite Time
ACTA ASTRONAUTICA 2021
被引用19
ENGINEERING APPLICATIONS OF ARTIFICIAL INTELLIGENCE 2024
被引用9
Chattering-free Adaptive Iterative Learning for Attitude Tracking Control of Uncertain Spacecraft.
Automatica 2023
被引用6
AEROSPACE SCIENCE AND TECHNOLOGY 2023
被引用34
JOURNAL OF VIBRATION AND CONTROL 2024
被引用1
Data Disclaimer
The page data are from open Internet sources, cooperative publishers and automatic analysis results through AI technology. We do not make any commitments and guarantees for the validity, accuracy, correctness, reliability, completeness and timeliness of the page data. If you have any questions, please contact us by email: report@aminer.cn
Chat Paper
GPU is busy, summary generation fails
Rerequest