Development Of Phenotyping System Using Low Altitude Uav Imagery And Deep Learning
INTERNATIONAL JOURNAL OF AGRICULTURAL AND BIOLOGICAL ENGINEERING(2021)
摘要
In this study, a lightweight phenotyping system that combined the advantages of both deep learning-based panicle detection and the photogrammetry based on light consumer-level UAVs was proposed. A two-year experiment was conducted to perform data collection and accuracy validation. A deep learning model, named Mask Region-based Convolutional Neural Network (Mask R-CNN), was trained to detect panicles in complex scenes of paddy fields. A total of 13 857 images were fed into Mask R-CNN, with 80% used for training and 20% used for validation. Scores, precision, recall, Average Precision (AP), and F1-score of the Mask R-CNN, were 82.46%, 80.60%, 79.46%, and 79.66%, respectively. A complete workflow was proposed to preprocess flight trajectories and remove repeated detection and noises. Eventually, the evident changed in rice growth during the heading stage was visualized with geographic distributions, and the total number of panicles was predicted before harvest. The average error of the predicted amounts of panicles was 33.98%. Experimental results showed the feasibility of using the developed system as the high-throughput phenotyping approach.
更多查看译文
关键词
panicle detection, vision-based phenotyping, deep learning, unmanned aerial vehicle (UAV)
AI 理解论文
溯源树
样例
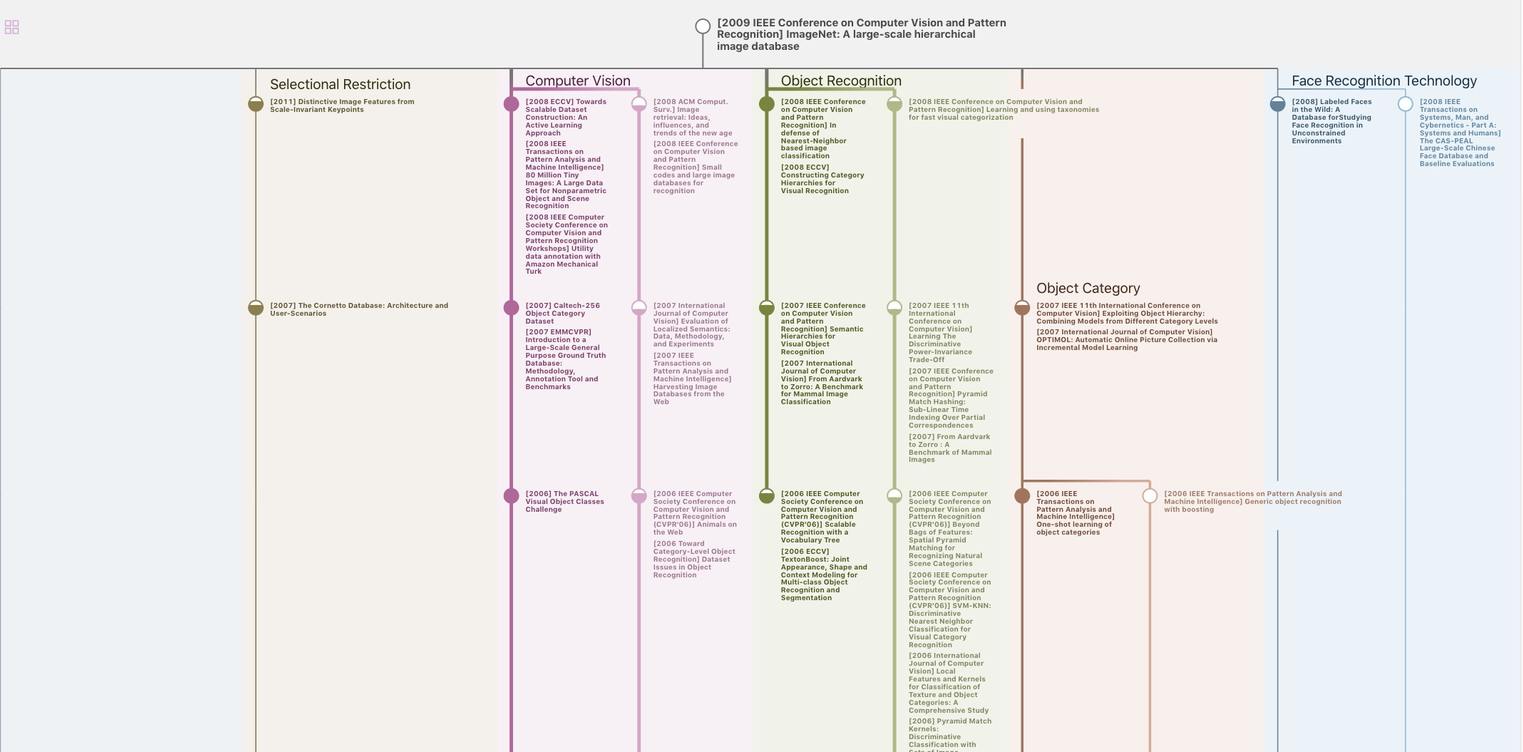
生成溯源树,研究论文发展脉络
Chat Paper
正在生成论文摘要