RAU-Net: U-Net Model Based on Residual and Attention for Kidney and Kidney Tumor Segmentation
2021 IEEE International Conference on Consumer Electronics and Computer Engineering (ICCECE)(2021)
摘要
Various variants based on U-Net model have made great achievements in various medical image segmentation competitions, however their ability to generalize is less than satisfactory. Therefore, RAU-Net, our proposed model is used for renal tumors segmentation. To improve the performance of the model, the work can be summarized as the following four points: Above all, we have proposed an end-to-end automatic segmentation model, which combined with residual and attention, and allowed us to obtain the kidney and kidney tumor just by preconditioning. Second, the weighted dice loss function and the cross entropy loss function enable the model to fully identify the positive samples and improve the tumor sensitivity. Third, the pretreatment and post-treatment combined with traditional methods and machine learning methods provide us with the possibility to accurately segment kidney and kidney tumor, and improve the segmentation results. Finally, in the KiTS19 dataset (a total of 210 patients), we divided the training set and test set by 8:2, and then obtained the average dice of 0.96 and 0.77 for the kidney and tumor segmentation, also gained the global dice of 0.96 and 0.92 for kidney and tumor segmentation respectively.
更多查看译文
关键词
U-Net,RAU-Net,end-to-end,loss function,renal tumors segmentation
AI 理解论文
溯源树
样例
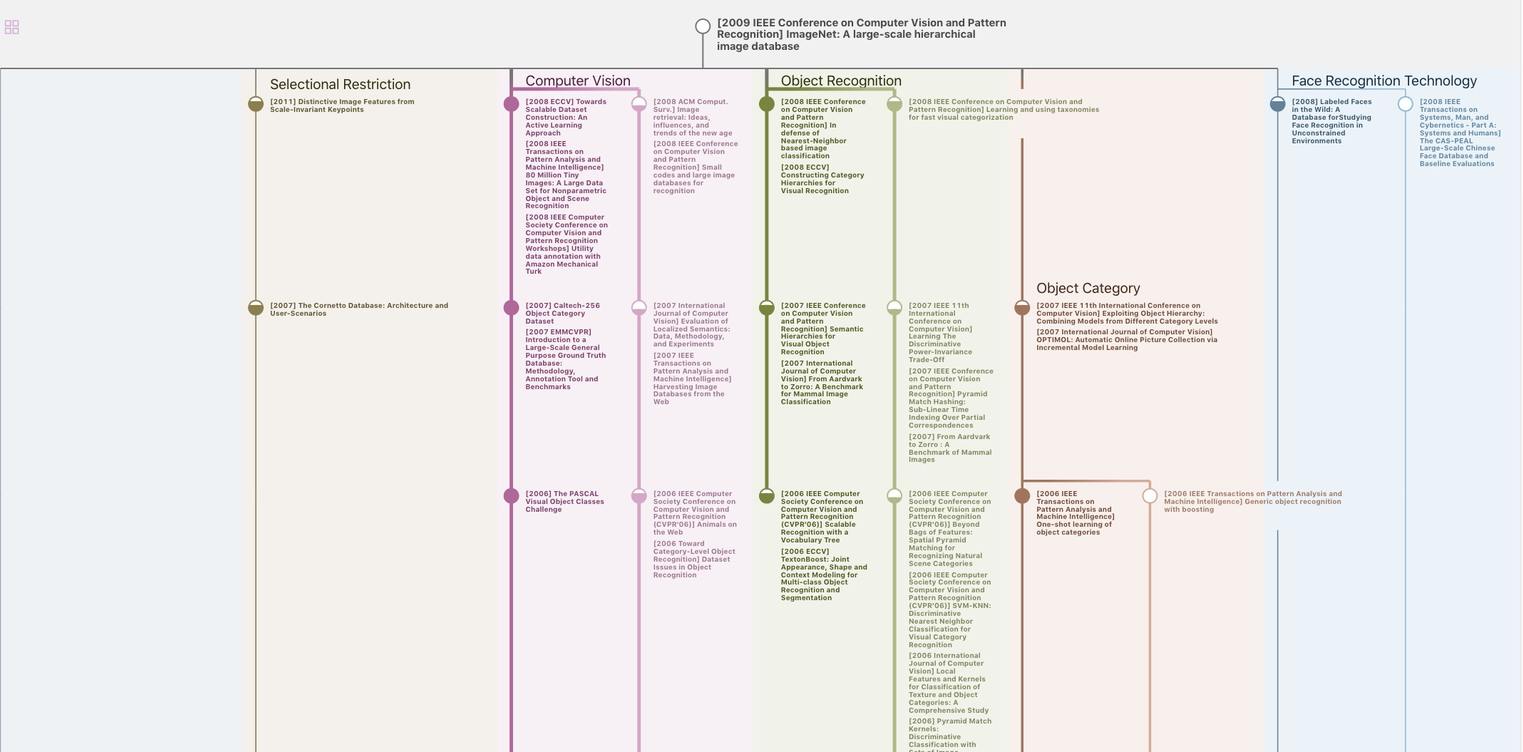
生成溯源树,研究论文发展脉络
Chat Paper
正在生成论文摘要