Learning to Recommend Frame for Interactive Video Object Segmentation in the Wild
2021 IEEE/CVF CONFERENCE ON COMPUTER VISION AND PATTERN RECOGNITION, CVPR 2021(2021)
摘要
This paper proposes a framework for the interactive video object segmentation (VOS) in the wild where users can choose some frames for annotations iteratively. Then, based on the user annotations, a segmentation algorithm refines the masks. The previous interactive VOS paradigm selects the frame with some worst evaluation metric, and the ground truth is required for calculating the evaluation metric, which is impractical in the testing phase. In contrast, in this paper, we advocate that the frame with the worst evaluation metric may not be exactly the most valuable frame that leads to the most performance improvement across the video. Thus, we formulate the frame selection problem in the interactive VOS as a Markov Decision Process, where an agent is learned to recommend the frame under a deep reinforcement learning framework. The learned agent can automatically determine the most valuable frame, making the interactive setting more practical in the wild. Experimental results on the public datasets show the effectiveness of our learned agent without any changes to the underlying VOS algorithms.
更多查看译文
关键词
frame selection problem,deep reinforcement learning framework,learned agent,interactive video object segmentation,user annotations,worst evaluation metric,interactive VOS paradigm,Markov decision process,public datasets
AI 理解论文
溯源树
样例
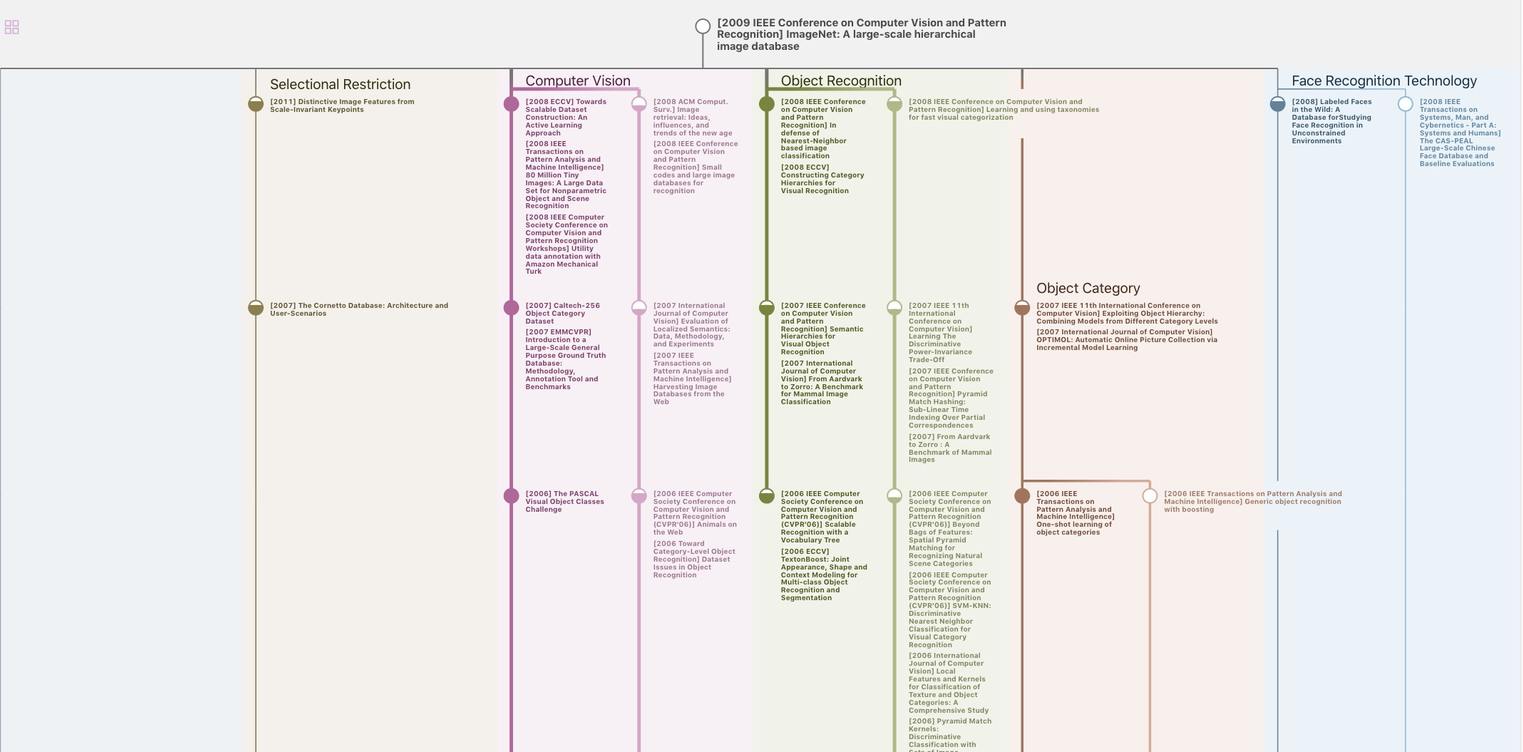
生成溯源树,研究论文发展脉络
Chat Paper
正在生成论文摘要