Do Word Embeddings Really Understand Loughran-McDonald's Polarities?
arxiv(2021)
摘要
In this paper we perform a rigorous mathematical analysis of the word2vec model, especially when it is equipped with the Skip-gram learning scheme. Our goal is to explain how embeddings, that are now widely used in NLP (Natural Language Processing), are influenced by the distribution of terms in the documents of the considered corpus. We use a mathematical formulation to shed light on how the decision to use such a model makes implicit assumptions on the structure of the language. We show how Markovian assumptions, that we discuss, lead to a very clear theoretical understanding of the formation of embeddings, and in particular the way it captures what we call frequentist synonyms. These assumptions allow to produce generative models and to conduct an explicit analysis of the loss function commonly used by these NLP techniques. Moreover, we produce synthetic corpora with different levels of structure and show empirically how the word2vec algorithm succeed, or not, to learn them. It leads us to empirically assess the capability of such models to capture structures on a corpus of around 42 millions of financial News covering 12 years. That for, we rely on the Loughran-McDonald Sentiment Word Lists largely used on financial texts and we show that embeddings are exposed to mixing terms with opposite polarity, because of the way they can treat antonyms as frequentist synonyms. Beside we study the non-stationarity of such a financial corpus, that has surprisingly not be documented in the literature. We do it via time series of cosine similarity between groups of polarized words or company names, and show that embedding are indeed capturing a mix of English semantics and joined distribution of words that is difficult to disentangle.
更多查看译文
关键词
word embeddings,polarities,loughran-mcdonald
AI 理解论文
溯源树
样例
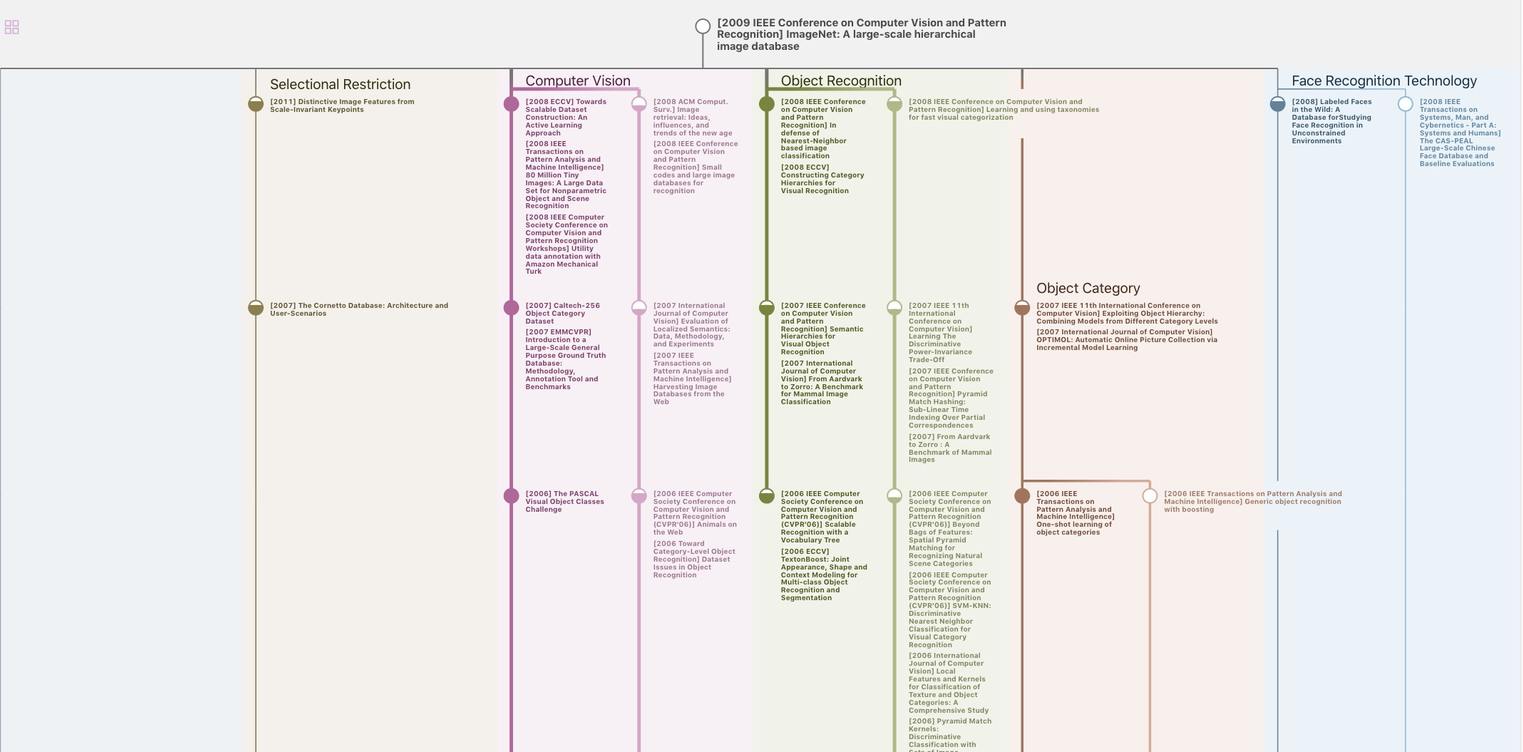
生成溯源树,研究论文发展脉络
Chat Paper
正在生成论文摘要