Cosmic Reionization on Computers: Statistical Properties of the Distributions of Mean Opacities
arxiv(2025)
Abstract
Quasar absorption lines provide a unique window to the relationship between galaxies and the intergalactic medium during the Epoch of Reionization. In particular, high redshift quasars enable measurements of the neutral hydrogen content of the universe. However, the limited sample size of observed quasar spectra, particularly at the highest redshifts, hampers our ability to fully characterize the intergalactic medium during this epoch from observations alone. In this work, we characterize the distributions of mean opacities of the intergalactic medium in simulations from the Cosmic Reionization on Computers (CROC) project. We find that the distribution of mean opacities along sightlines follows a non-trivial distribution that cannot be easily approximated by a known distribution. When comparing the cumulative distribution function of mean opacities measurements in subsamples of sample sizes similar to observational measurements from the literature, we find consistency between CROC and observations at redshifts z≲ 5.7. However, at higher redshifts (z≳5.7), the cumulative distribution function of mean opacities from CROC is notably narrower than those from observed quasar sightlines implying that observations probe a systematically more opaque intergalactic medium at higher redshifts than the intergalactic medium in CROC boxes at these same redshifts. This is consistent with previous analyses that indicate that the universe is reionized too early in CROC simulations.
MoreTranslated text
PDF
View via Publisher
AI Read Science
AI Summary
AI Summary is the key point extracted automatically understanding the full text of the paper, including the background, methods, results, conclusions, icons and other key content, so that you can get the outline of the paper at a glance.
Example
Background
Key content
Introduction
Methods
Results
Related work
Fund
Key content
- Pretraining has recently greatly promoted the development of natural language processing (NLP)
- We show that M6 outperforms the baselines in multimodal downstream tasks, and the large M6 with 10 parameters can reach a better performance
- We propose a method called M6 that is able to process information of multiple modalities and perform both single-modal and cross-modal understanding and generation
- The model is scaled to large model with 10 billion parameters with sophisticated deployment, and the 10 -parameter M6-large is the largest pretrained model in Chinese
- Experimental results show that our proposed M6 outperforms the baseline in a number of downstream tasks concerning both single modality and multiple modalities We will continue the pretraining of extremely large models by increasing data to explore the limit of its performance
Try using models to generate summary,it takes about 60s
Must-Reading Tree
Example
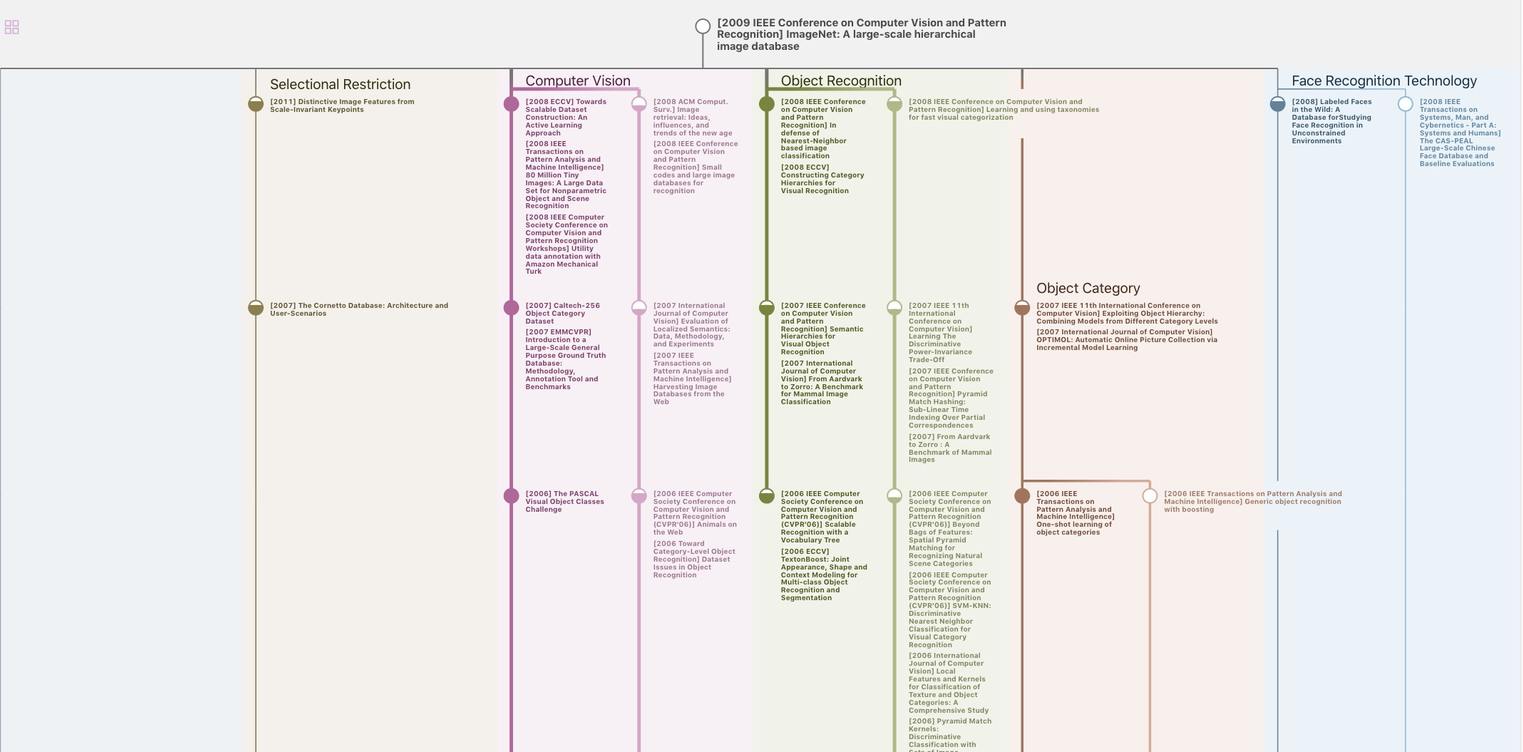
Generate MRT to find the research sequence of this paper
Data Disclaimer
The page data are from open Internet sources, cooperative publishers and automatic analysis results through AI technology. We do not make any commitments and guarantees for the validity, accuracy, correctness, reliability, completeness and timeliness of the page data. If you have any questions, please contact us by email: report@aminer.cn
Chat Paper
去 AI 文献库 对话