Optimizing Multi-User Semantic Communication via Transfer Learning and Knowledge Distillation
CoRR(2024)
摘要
Semantic communication, notable for ensuring quality of service by jointly
optimizing source and channel coding, effectively extracts data semantics,
reduces transmission length, and mitigates channel noise. However, most studies
overlook multi-user scenarios and resource availability, limiting real-world
application. This paper addresses this gap by focusing on downlink
communication from a base station to multiple users with varying computing
capacities. Users employ variants of Swin transformer models for source
decoding and a simple architecture for channel decoding. We propose a novel
training regimen, incorporating transfer learning and knowledge distillation to
improve low-computing users' performance. Extensive simulations validate the
proposed methods.
更多查看译文
AI 理解论文
溯源树
样例
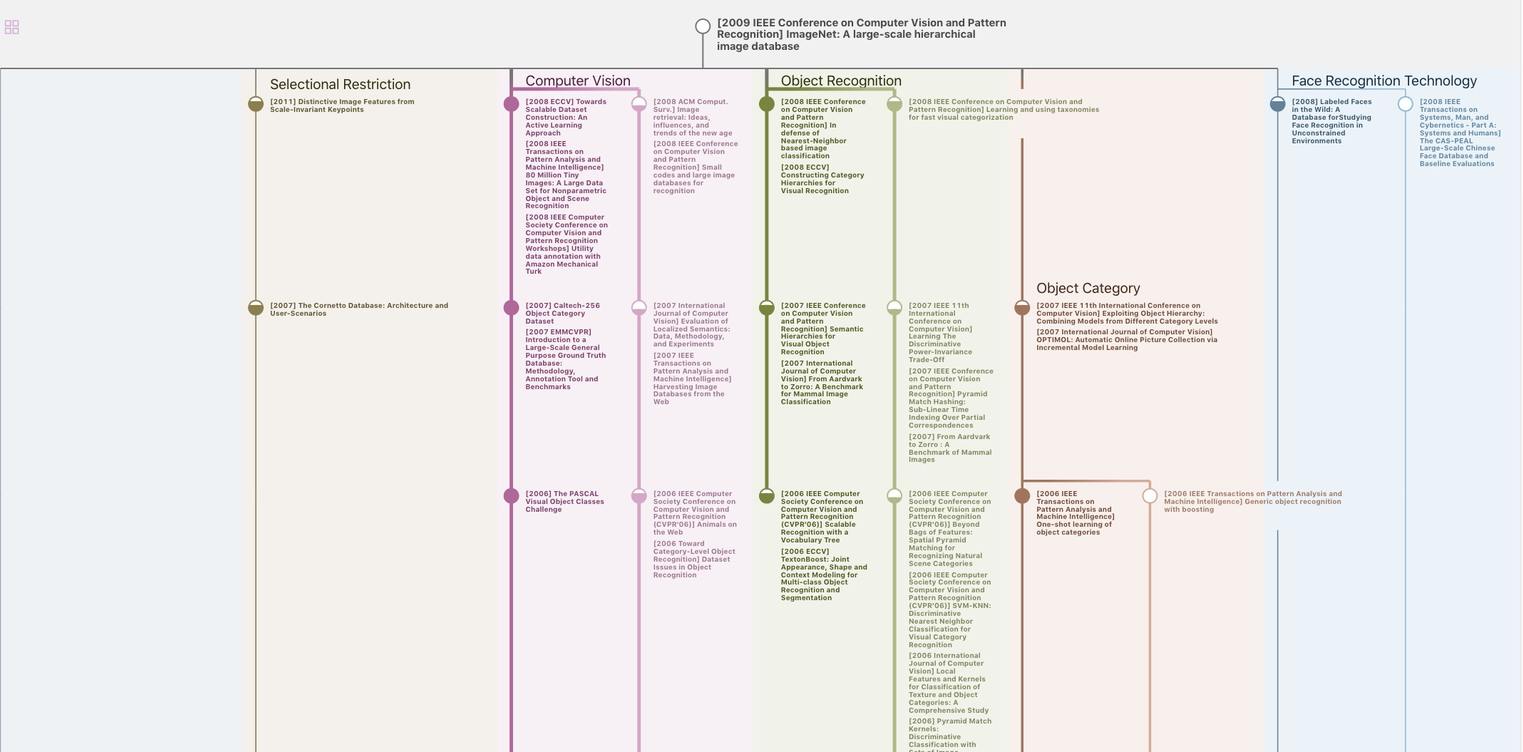
生成溯源树,研究论文发展脉络
Chat Paper
正在生成论文摘要