On-Device Extractive Text Summarization
2021 IEEE 15th International Conference on Semantic Computing (ICSC)(2021)
摘要
With increasing connectivity, there has been an exponential surge in the creation and availability of textual content in the form of news articles, blogs, social media posts and product reviews. A large portion of this data is consumed on mobile devices, and more recently, through wearables and smart speakers. Text summarization involves generating a brief description of a text, which captures the overall intention and the vital information being conveyed in its content. Common techniques for automatic text summarization follow extractive or abstractive approaches and involve large scale models with millions of parameters. While such models can be utilized in web or cloud-based applications, they are impractical for deployment on devices with limited storage and computational capabilities. In this paper, we propose a novel character-level neural architecture for extractive text summarization, with the model size reduced by 99.64% to 97.98% from existing methods, thus making it suitable for deployment on-device such as in mobiles, tabs and smart speakers. We tested the performance of our model on various benchmark datasets and compared it with several strong baselines and models. Despite using only a fraction of the space, our model outperformed the baselines and several state-of-the-art models, while coming close in performance with others. On-device text summarization remains largely an unexplored area, and our model's results show a promising approach towards building summarization models suitable for a constrained environment.
更多查看译文
关键词
Text Summarization,On Device,Character model,Extractive Summarization
AI 理解论文
溯源树
样例
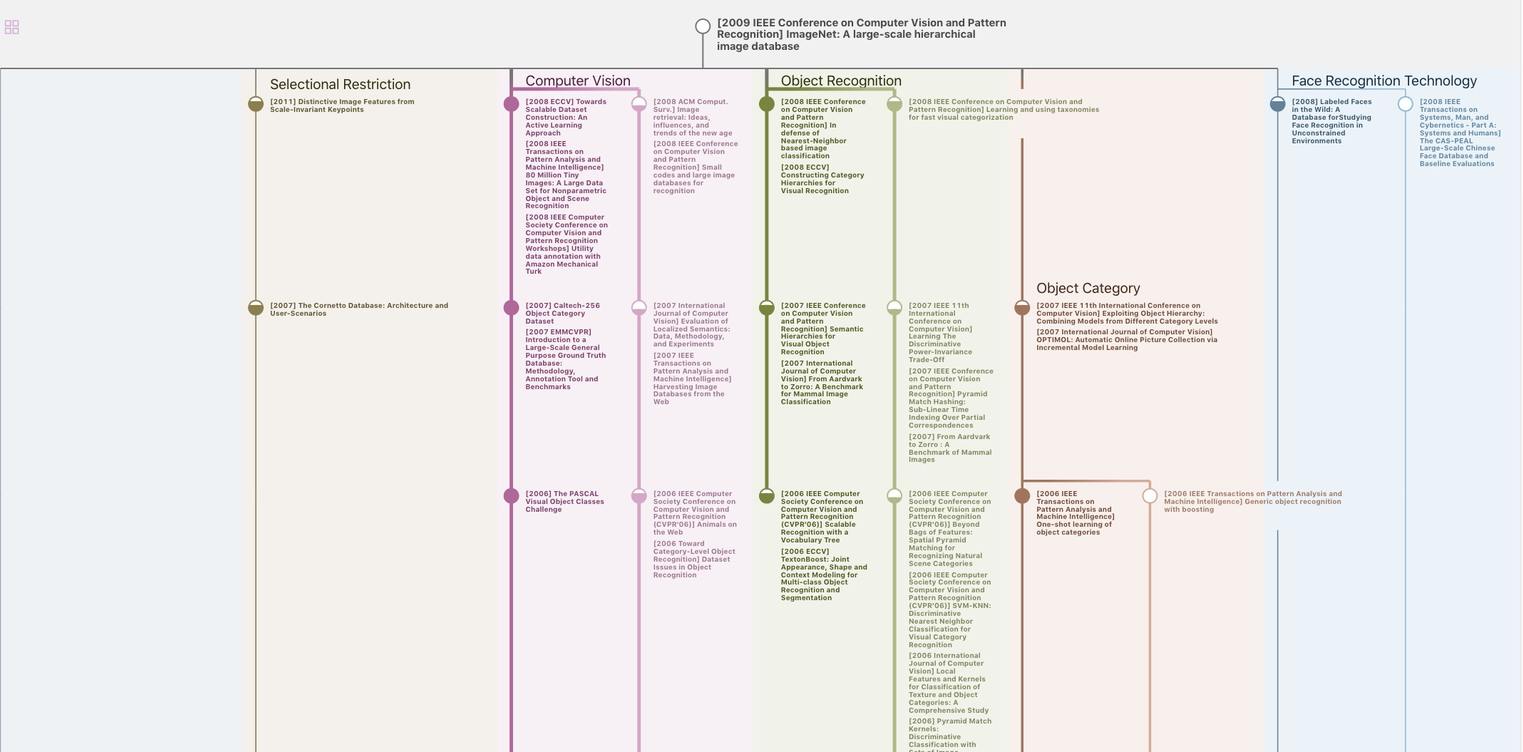
生成溯源树,研究论文发展脉络
Chat Paper
正在生成论文摘要