Curriculum-Based Reinforcement Learning for Quadrupedal Jumping: A Reference-free Design
arXiv (Cornell University)(2024)
Abstract
Deep reinforcement learning (DRL) has emerged as a promising solution tomastering explosive and versatile quadrupedal jumping skills. However, currentDRL-based frameworks usually rely on well-defined reference trajectories, whichare obtained by capturing animal motions or transferring experience fromexisting controllers. This work explores the possibility of learning dynamicjumping without imitating a reference trajectory. To this end, we incorporate acurriculum design into DRL so as to accomplish challenging tasks progressively.Starting from a vertical in-place jump, we then generalize the learned policyto forward and diagonal jumps and, finally, learn to jump across obstacles.Conditioned on the desired landing location, orientation, and obstacledimensions, the proposed approach contributes to a wide range of jumpingmotions, including omnidirectional jumping and robust jumping, alleviating theeffort to extract references in advance. Particularly, without constraints fromthe reference motion, a 90cm forward jump is achieved, exceeding previousrecords for similar robots reported in the existing literature. Additionally,continuous jumping on the soft grassy floor is accomplished, even when it isnot encountered in the training stage. A supplementary video showing ourresults can be found at https://youtu.be/nRaMCrwU5X8 .
MoreTranslated text
Key words
Cognitive Development
AI Read Science
Must-Reading Tree
Example
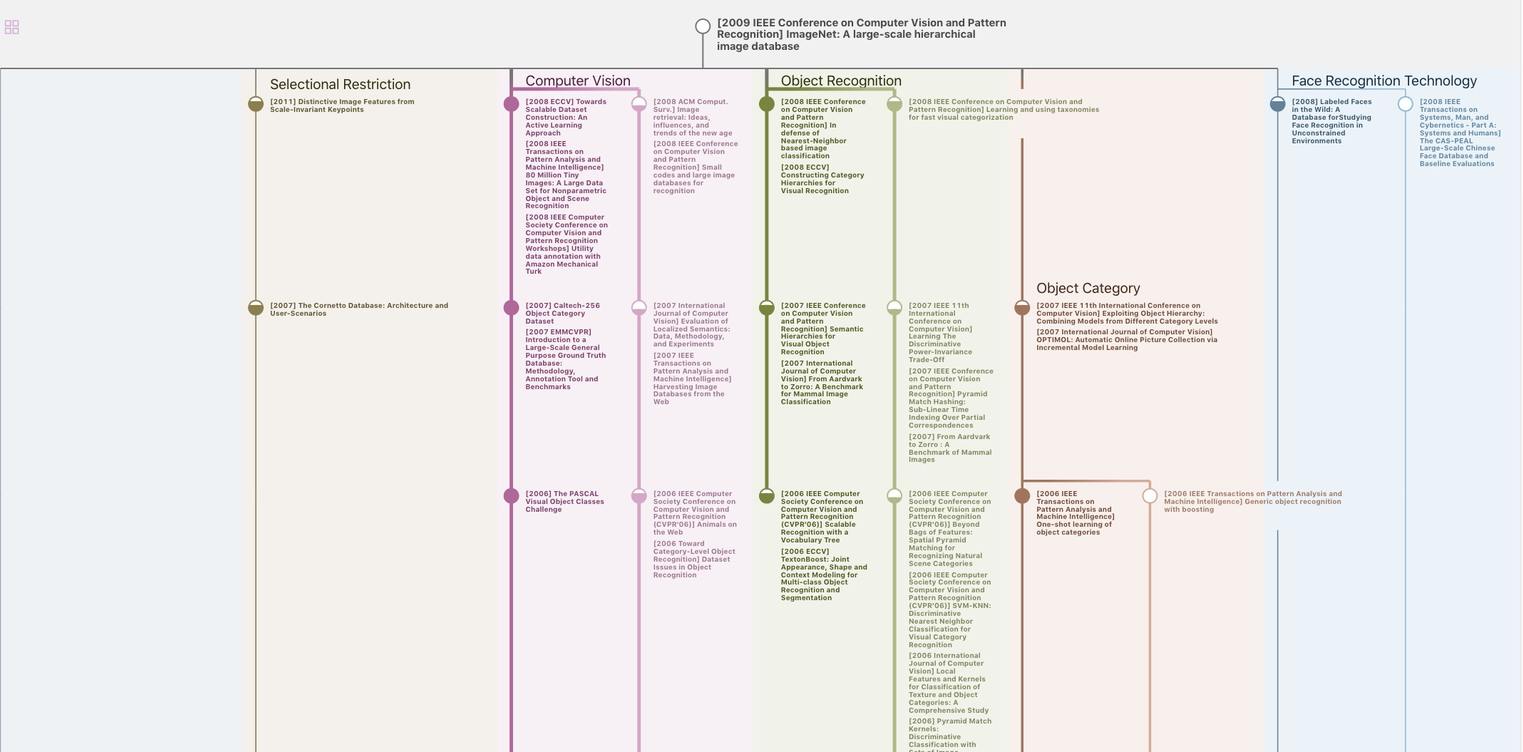
Generate MRT to find the research sequence of this paper
Chat Paper
Summary is being generated by the instructions you defined