An Improvised Machine Learning Model Based on Mutual Information Feature Selection Approach for Microbes Classification.
Entropy(2021)
摘要
The accurate classification of microbes is critical in today's context for monitoring the ecological balance of a habitat. Hence, in this research work, a novel method to automate the process of identifying microorganisms has been implemented. To extract the bodies of microorganisms accurately, a generalized segmentation mechanism which consists of a combination of convolution filter (Kirsch) and a variance-based pixel clustering algorithm (Otsu) is proposed. With exhaustive corroboration, a set of twenty-five features were identified to map the characteristics and morphology for all kinds of microbes. Multiple techniques for feature selection were tested and it was found that mutual information (MI)-based models gave the best performance. Exhaustive hyperparameter tuning of multilayer layer perceptron (MLP), k-nearest neighbors (KNN), quadratic discriminant analysis (QDA), logistic regression (LR), and support vector machine (SVM) was done. It was found that SVM radial required further improvisation to attain a maximum possible level of accuracy. Comparative analysis between SVM and improvised SVM (ISVM) through a 10-fold cross validation method ultimately showed that ISVM resulted in a 2% higher performance in terms of accuracy (98.2%), precision (98.2%), recall (98.1%), and F1 score (98.1%).
更多查看译文
关键词
mutual information,classification,k-fold cross validation,machine learning modeling,image segmentation,microorganisms
AI 理解论文
溯源树
样例
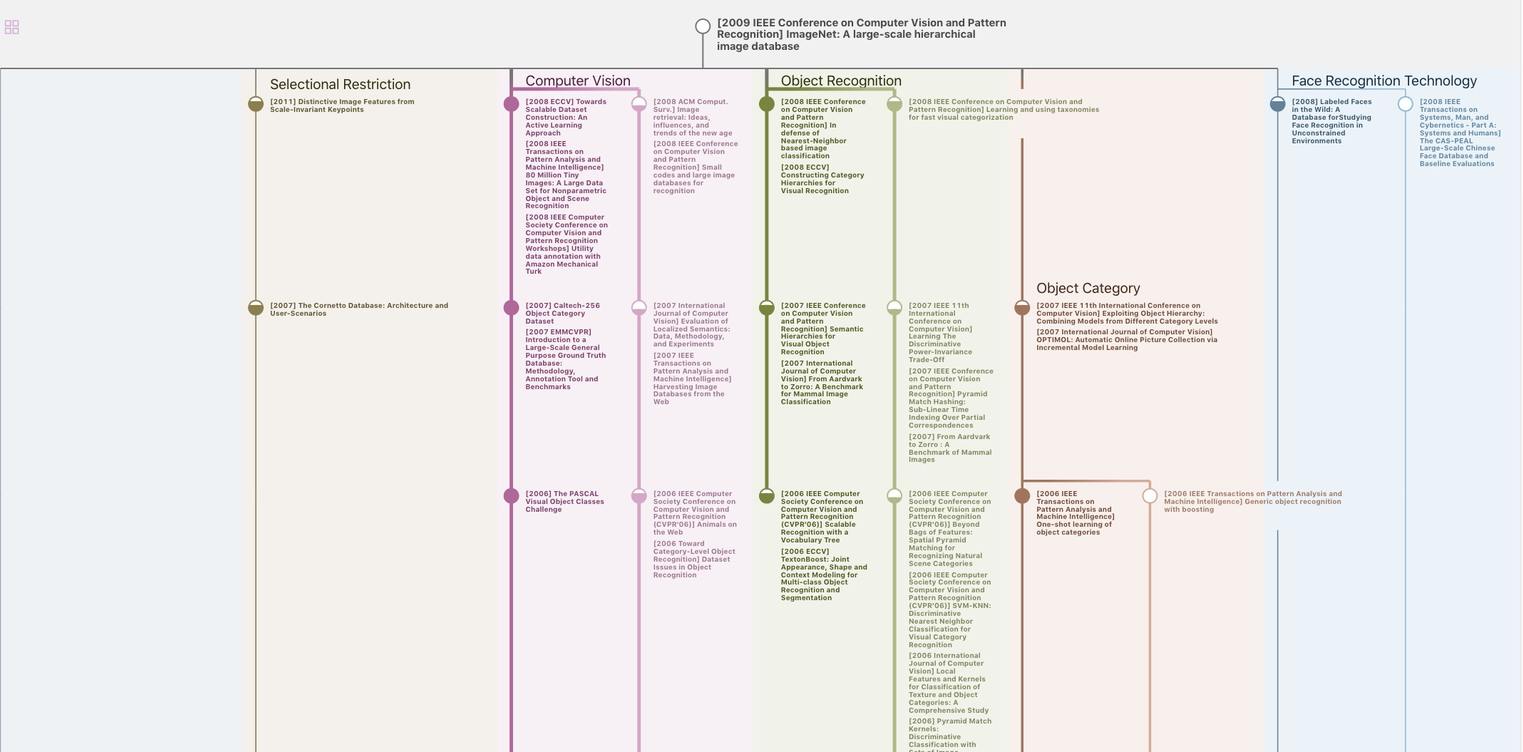
生成溯源树,研究论文发展脉络
Chat Paper
正在生成论文摘要