Differential Tweetment: Mitigating Racial Dialect Bias in Harmful Tweet Detection
PROCEEDINGS OF THE 2021 ACM CONFERENCE ON FAIRNESS, ACCOUNTABILITY, AND TRANSPARENCY, FACCT 2021(2021)
摘要
Automated systems for detecting harmful social media content are afflicted by a variety of biases, some of which originate in their training datasets. In particular, some systems have been shown to propagate racial dialect bias: they systematically classify content aligned with the African American English (AAE) dialect as harmful at a higher rate than content aligned with White English (WE). This perpetuates prejudice by silencing the Black community. Towards this problem we adapt and apply two existing bias mitigation approaches: preferential sampling pre-processing and adversarial debiasing in-processing. We analyse the impact of our interventions on model performance and propagated bias. We find that when bias mitigation is employed, a high degree of predictive accuracy is maintained relative to baseline, and in many cases bias against AAE in harmful tweet predictions is reduced. However, the specific effects of these interventions on bias and performance vary widely between dataset contexts. This variation suggests the unpredictability of autonomous harmful content detection outside of its development context. We argue that this, and the low performance of these systems at baseline, raise questions about the reliability and role of such systems in high-impact, real-world settings.
更多查看译文
关键词
bias,fairness,racial disparities,dialect,content moderation
AI 理解论文
溯源树
样例
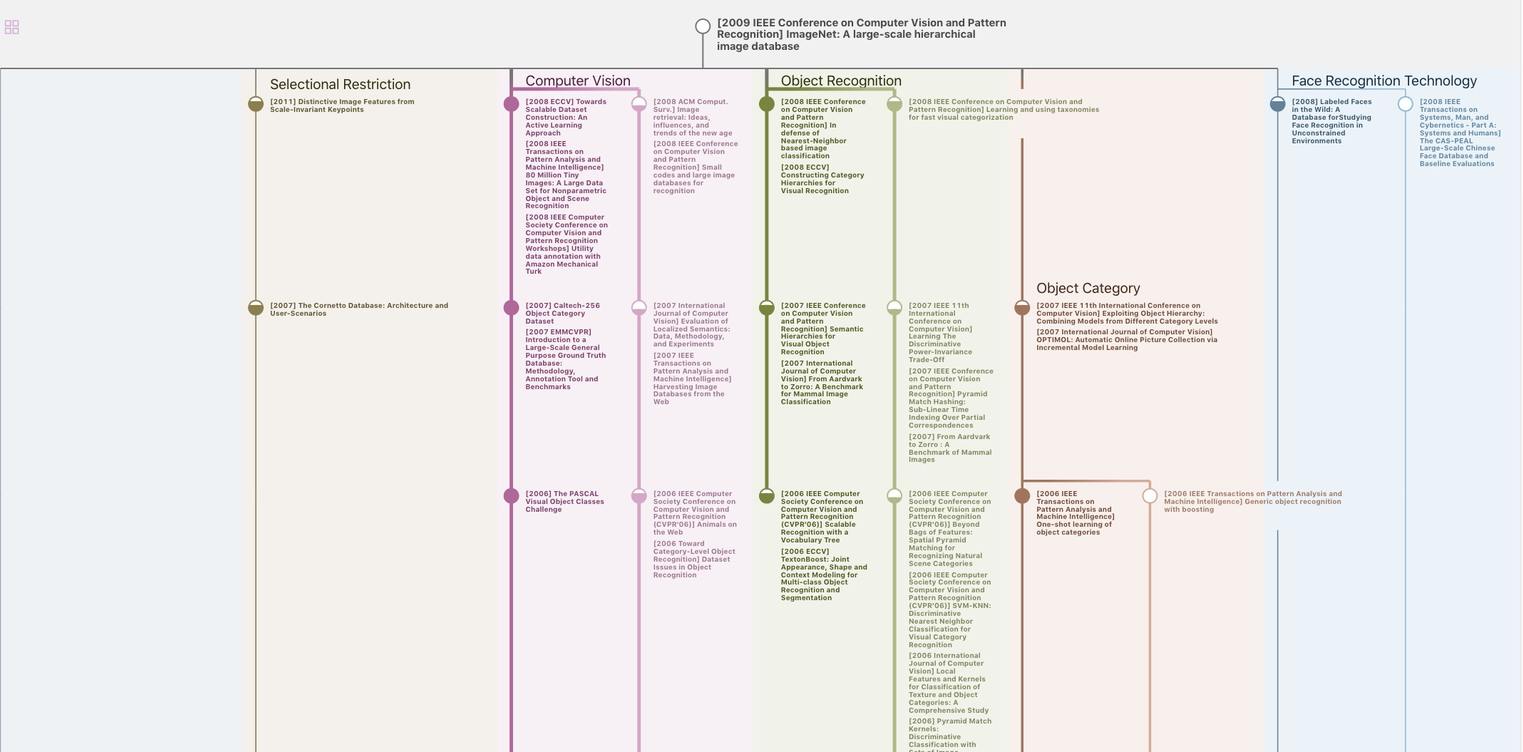
生成溯源树,研究论文发展脉络
Chat Paper
正在生成论文摘要