Model-based reinforcement learning with missing data
2020 Eighth International Symposium on Computing and Networking Workshops (CANDARW)(2020)
摘要
Model-based reinforcement learning is a powerful paradigm for learning tasks in robotics. However, real world learning tasks often involve complex patterns of missing data, and model-based reinforcement learning cannot handle missing data directly. To overcome this problem, in this paper, we focus on M-PGPE(GP) proposed by Mori et al. as a model-based reinforcement learning, and propose an extension of M-PGPE(GP) to handle missing data, which we call MM-PGPE(GP). The performance of the proposed MM-PGPE(GP) is assessed in two experiments with mountain car task. These experiments highlight the MM-PGPE(GP) produces higher average return and outperforms the conventional M-PGPE(GP) with simple linear interpolation.
更多查看译文
关键词
reinforcement learning,model-based reinforcement learning,PGPE,missing data
AI 理解论文
溯源树
样例
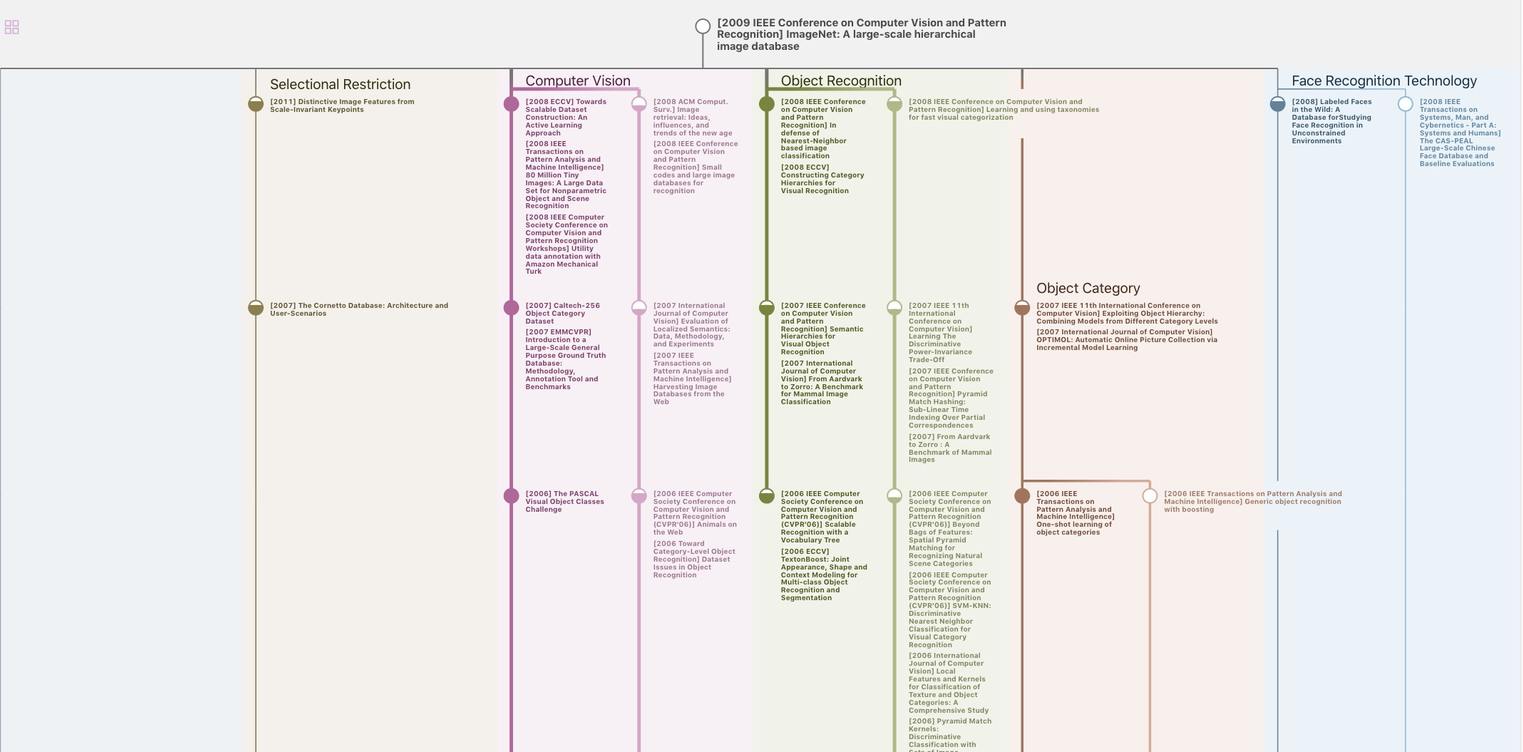
生成溯源树,研究论文发展脉络
Chat Paper
正在生成论文摘要