Feature-Align Network with Knowledge Distillation for Efficient Denoising
2022 IEEE/CVF Winter Conference on Applications of Computer Vision Workshops (WACVW)(2022)
摘要
We propose an efficient neural network for RAW image denoising. Although neural network-based denoising has been extensively studied for image restoration, little attention has been given to efficient denoising for compute limited and power sensitive devices, such as smartphones and wearables. In this paper, we present a novel architecture and a suite of training techniques for high quality denoising in mobile devices. Our work is distinguished by three main contributions. (1) The Feature-Align layer that modulates the activations of an encoder-decoder architecture with the input noisy images. The auto modulation layer enforces attention to spatially varying noise that tends to be “washed away” by successive application of convolutions and non-linearity. (2) A novel Feature Matching Loss that allows knowledge distillation from large denoising networks in the form of a perceptual content loss. (3) Empirical analysis of our efficient model trained to specialize on different noise subranges. This opens an additional avenue for model size reduction by sacrificing memory for compute. Extensive experimental validation shows that our efficient model produces high quality denoising results that compete with state-of-the-art large networks, while using significantly fewer parameters and MACs. On the Darmstadt Noise Dataset benchmark, we achieve a PSNR of 48. 28dB, while using $263\times$ fewer MACs and $17.6\times$ fewer parameters than the state-of-the-art network, which achieves 49. 12dB.
更多查看译文
关键词
efficient denoising,knowledge distillation,feature-align
AI 理解论文
溯源树
样例
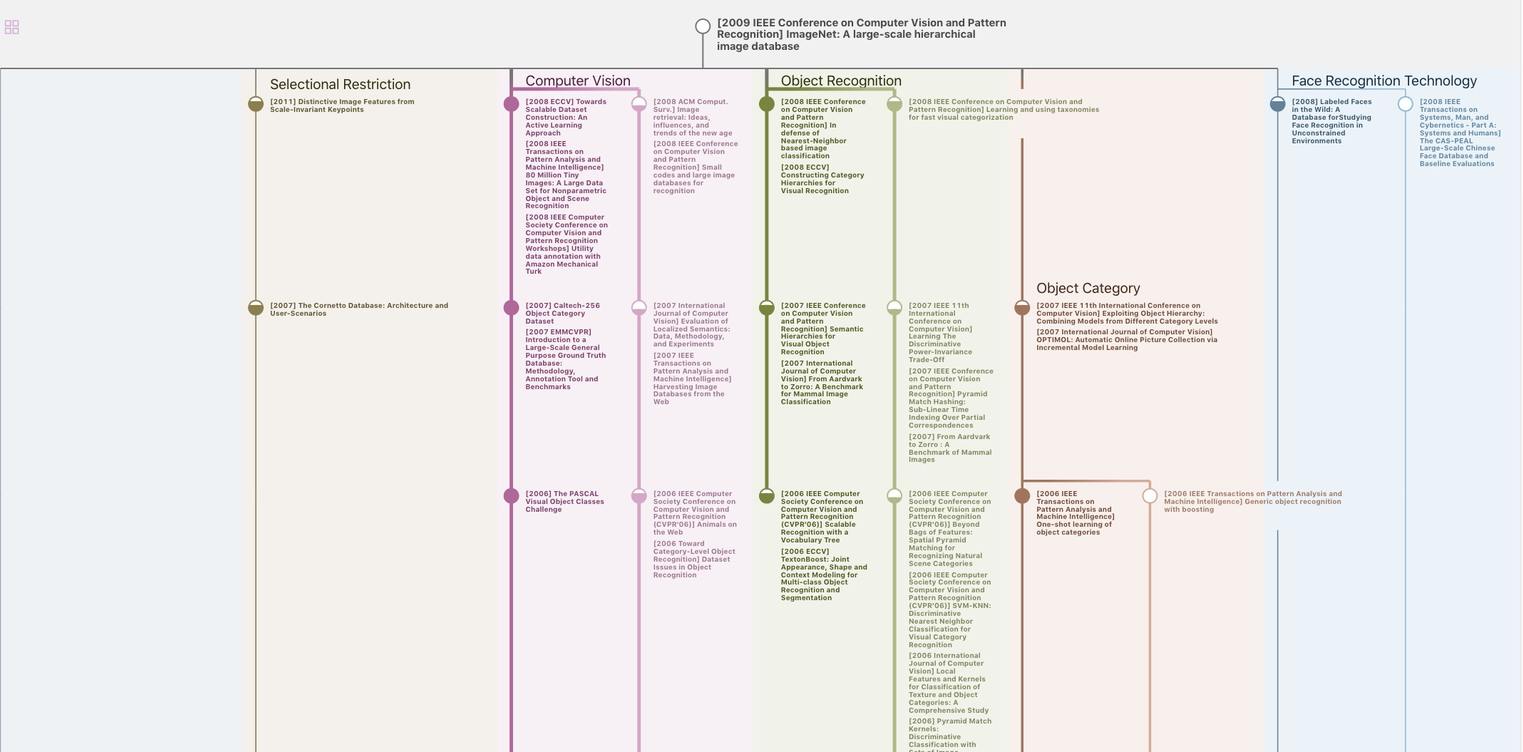
生成溯源树,研究论文发展脉络
Chat Paper
正在生成论文摘要