Bid-Aware Privacy-Preserving Participant Recruitment in Mobile Crowd-Sensing
2020 IEEE 92nd Vehicular Technology Conference (VTC2020-Fall)(2020)
摘要
With the prevalence of smartphones, mobile crowd-sensing (MCS) becomes an appealing paradigm for sensing and collecting data. However, this rises new privacy concerns making participants reluctant to conduct sensing tasks. To cope with this problem, we develop a new privacy-preserving incentive framework for worker recruitment in MCS campaigns. This task is challenging as, on the one hand, the workers have heterogeneous requirements to protect their sensitive information, and on the other hand, the MCS platform looks for the participants who ensure its utility in terms of data quality. Therefore, our study concentrates specifically on the design of a privacy-preserving mapping mechanism to achieve an adequate privacy-utility trade-off. Particularly, we formulate an optimization problem that allows the platform to select among the participants those who maximize its utility after bidding and receiving their privacy needs. Once the workers are recruited to fulfill the sensing task, the platform provides them with incentives to cover their personal data exposure. Considering all these measures and the formulated optimization problem, we develop a meta-heuristic tabu-search based algorithm as the basis of our computationally efficient auction mechanism. The proposed meta-heuristic algorithm aims at revealing the false bids submitted by selfish workers who want to maximize their rewards while providing low data quality samples. As a result, we obtain a truthful and individually rational incentive process. The proposed solution is validated through extensive simulations using real-datasets. This demonstrates that our framework responds to the workers' heterogeneous privacy constrains while maximizing the platform utility.
更多查看译文
关键词
mobile crowd-sensing,appealing paradigm,privacy concerns,sensing task,privacy-preserving incentive framework,worker recruitment,MCS campaigns,MCS platform,privacy-preserving mapping mechanism,adequate privacy-utility trade-off,privacy needs,personal data exposure,meta-heuristic tabu-search based algorithm,computationally efficient auction mechanism,meta-heuristic algorithm,false bids,selfish workers,low data quality samples,individually rational incentive process,platform utility,bid-aware privacy-preserving participant recruitment
AI 理解论文
溯源树
样例
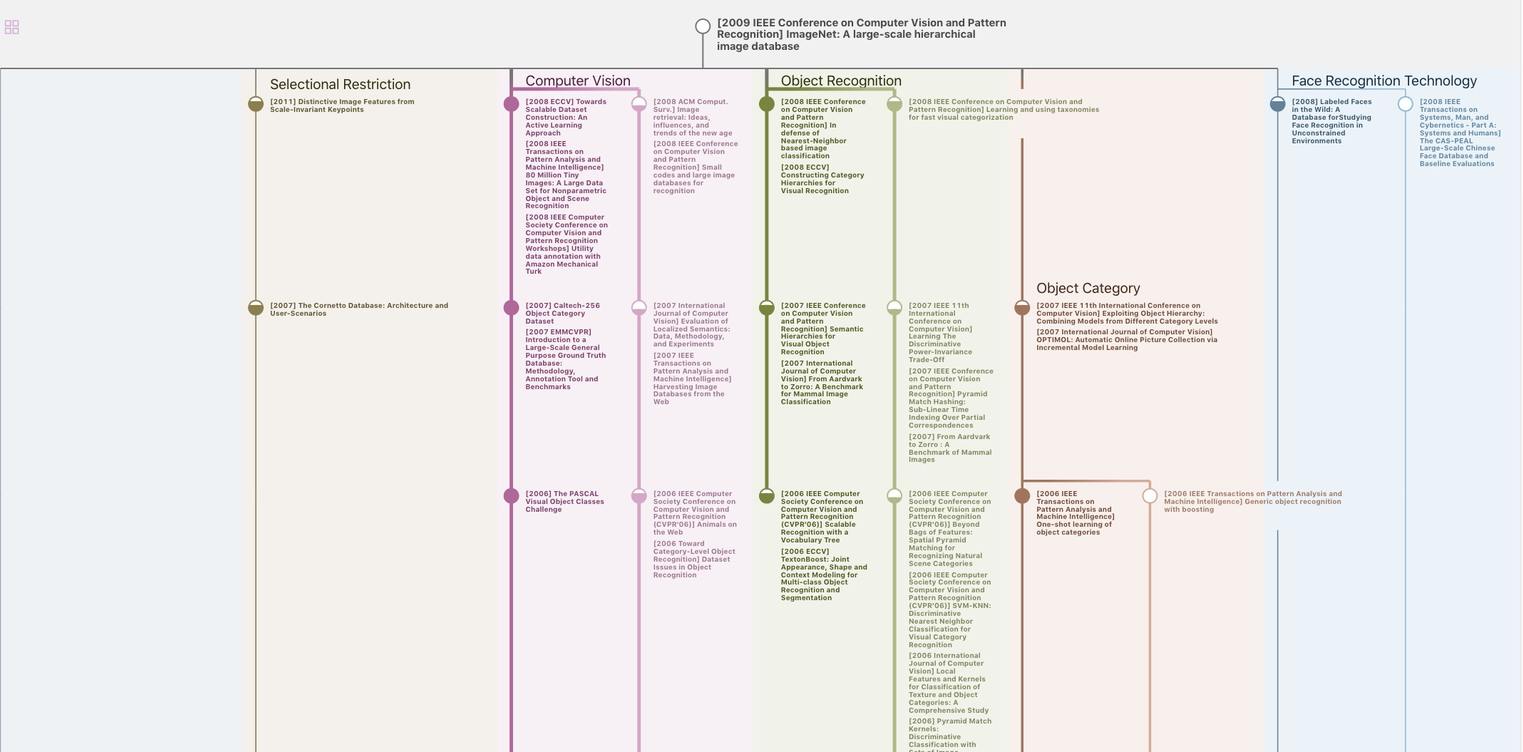
生成溯源树,研究论文发展脉络
Chat Paper
正在生成论文摘要