Adaptive Granularity Based Last-Level Cache Prefetching Method with Edram Prefetch Buffer for Graph Processing Applications
Applied sciences(2021)
摘要
The emergence of big data processing and machine learning has triggered the exponential growth of the working set sizes of applications. In addition, several modern applications are memory intensive with irregular memory access patterns. Therefore, we propose the concept of adaptive granularities to develop a prefetching methodology for analyzing memory access patterns based on a wider granularity concept that entails both cache lines and page granularity. The proposed prefetching module resides in the last-level cache (LLC) to handle the large working set of memory-intensive workloads. Additionally, to support memory access streams with variable intervals, we introduced an embedded-DRAM based LLC prefetch buffer that consists of three granularity-based prefetch engines and an access history table. By adaptively changing the granularity window for analyzing memory streams, the proposed model can swiftly and appropriately determine the stride of memory addresses to generate hidden delta chains from irregular memory access patterns. The proposed model achieves 18% and 15% improvements in terms of energy consumption and execution time compared to global history buffer and best offset prefetchers, respectively. In addition, our model reduced the total execution time and energy consumption by approximately 6% and 2.3%, compared to those of the Markov prefetcher and variable-length delta prefetcher.
更多查看译文
关键词
computer architecture,memory architecture,memory management,cache memory,prefetching,high-performance computing
AI 理解论文
溯源树
样例
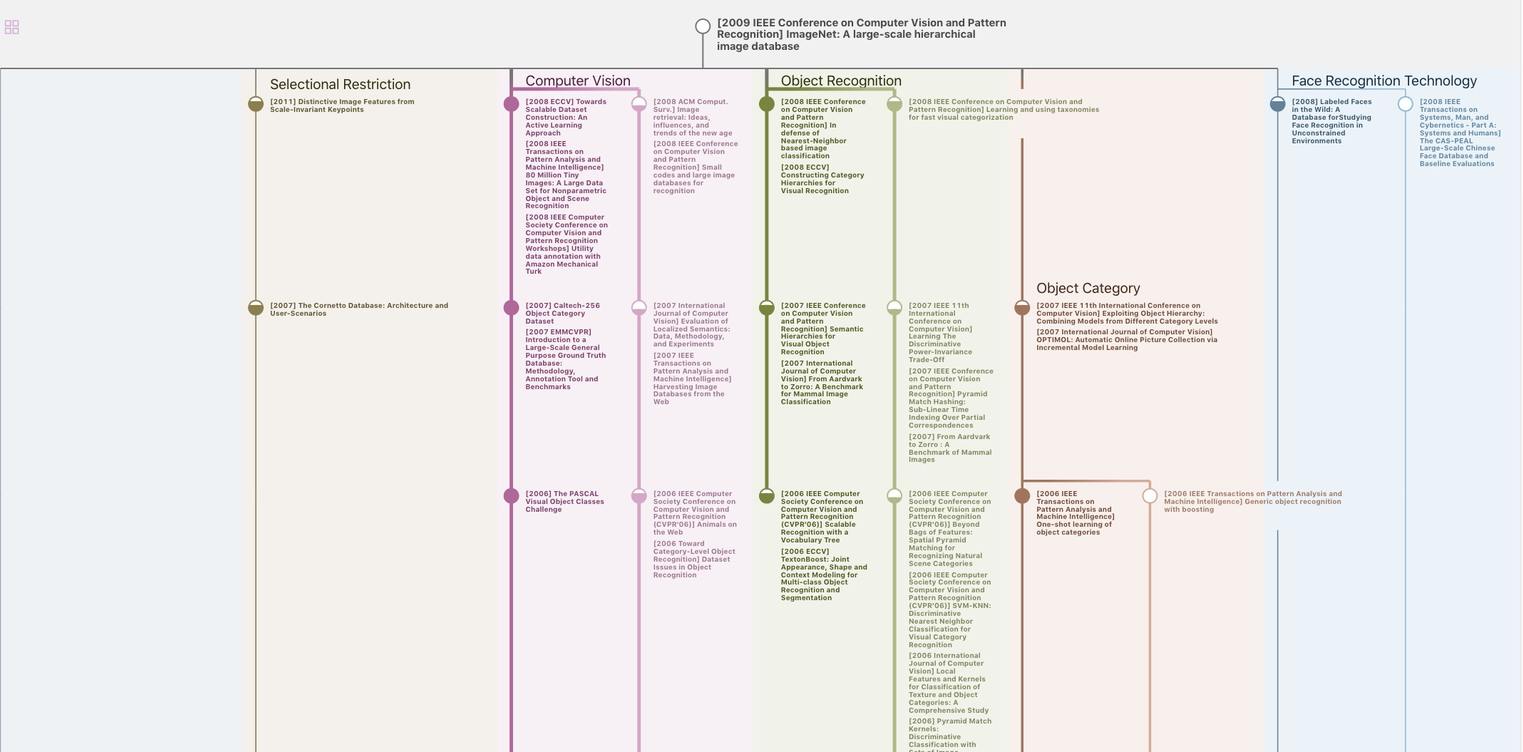
生成溯源树,研究论文发展脉络
Chat Paper
正在生成论文摘要