Cascaded SE-ResUnet for segmentation of thoracic organs at risk
Neurocomputing(2021)
Abstract
Computed Tomography (CT) has been widely used in the planning of radiation therapy, which is one of the most effective clinical lung cancer treatment options. Accurate segmentation of organs at risk (OARs) in thoracic CT images is a key step for radiotherapy planning to prevent healthy organs from getting over irradiation. However, known automatic image segmentation methods can hardly yield desired OAR delineation results, while manual delineation tends to take long time and tedious effort. In this paper, we propose a novel deep learning network, called cascaded SE-ResUnet, for automatic segmentation of thoracic organs including left lung, right lung, heart, esophagus, trachea, and spinal cord. Specifically, we first use a coarse segmentation network to identify the regions of interest (ROIs), and then a fine segmentation network is applied to achieve refined segmentation results, organ by organ. Finally, different configured models are ensembled to obtain the final segmentation results. In the StructSeg 2019 Challenge, we showed the capability of our new framework and won the 1st place at the test phase. Our code is available open-source at https://github.com/zjuybh/StructSeg2019. (c) 2021 Elsevier B.V. All rights reserved.
MoreTranslated text
Key words
Organs at risk,SE-ResUnet,Segmentation
AI Read Science
Must-Reading Tree
Example
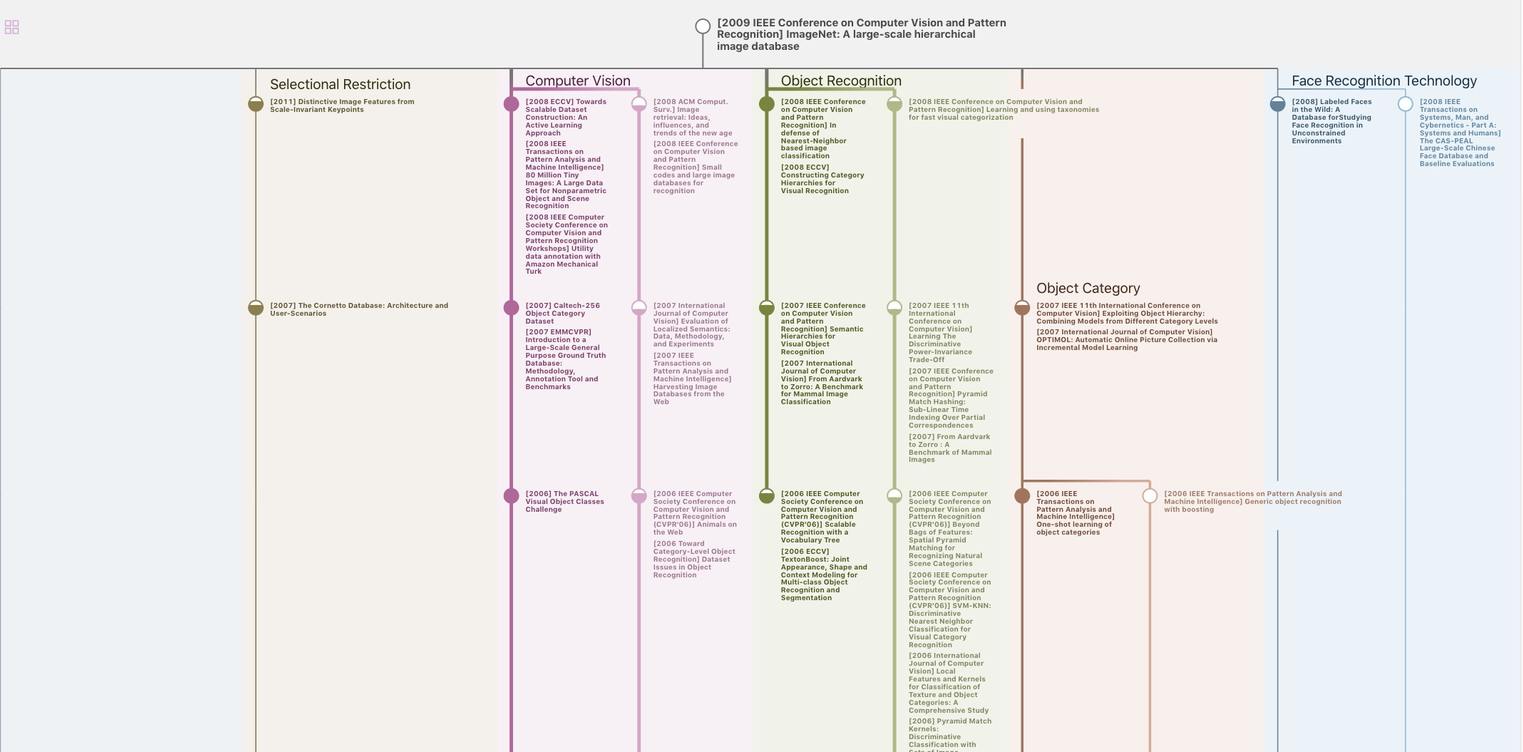
Generate MRT to find the research sequence of this paper
Chat Paper
Summary is being generated by the instructions you defined