Accounting for Correlational Structures in Stochastic Comparative Life Cycle Assessments Through Copula Modeling
The international journal of life cycle assessment(2021)
摘要
Purpose The presence of correlations between input parameters in a life cycle assessment (LCA) is a well-known issue. On top of that, the univariate distribution of environmental indicators, in most cases, does not follow the Gaussian nor the lognormal distribution. In this article, we introduce the copula modeling to build joint multivariate sampling spaces, irrespective of the marginal distributions of the environmental indicators, for LCA uncertainty analyses. To exemplify the proposed method, we integrate the copula modeling to the stochastic multiattribute analysis (SMAA) method to perform the normalization and weighting steps in a comparative agricultural LCA. Methods An attributional LCA was performed to compare the environmental impact of two tomato production systems (GH, greenhouse; OF, open field) with different intensification levels. To choose the best environmental performance system, we implemented the outranking procedure of the SMAA method but based on the true multivariate distribution of the environmental indicators. As required by the SMAA method, initially, we fitted skewed multivariate distributions among the environmental indicators but accounting for their correlation structure through the copula method. Afterwards, the standard SMAA procedure was followed, leading to the calculation of overall scores indicating the environmental performance of the systems under comparison. Results and discussion After individual LCAs were performed for each grower, the variability observed in the primary data was propagated to the environmental indicators. The marginal distributions of the environmental indicators showed a right skewed trend which were fitted to gamma, log-normal, or Weibull distributions as applicable. The application of the copula method for the environmental indicators of the GH and OF systems resulted in D-vine models consisting of 46 and 45 bivariate copulas requiring 47 parameters each, respectively. Sampling the multivariate space configured by the D-vine models and integrating it with the SMAA method indicated that the OF system is more likely to have a better environmental performance with a rank acceptability index (RAI) of 57.6% while the GH system yielded a lower RAI (42.4%). Conclusions We applied a stochastic unbiased approach to compare the environmental performance of agricultural systems but recognizing the correlation structure of the indicators. The copula method introduced here can be applied to uncertainty or multicriteria decision analysis where correlation needs to be accounted for. Joining the copula and the SMAA methods to produce an unbiased preference indicator allows to evaluate scenarios in a realistic way, producing results that can easily be communicated.
更多查看译文
关键词
Agricultural LCA,Copula,Error propagation,Monte Carlo simulation,Multivariate sampling distribution,Stochastic multiattribute analysis
AI 理解论文
溯源树
样例
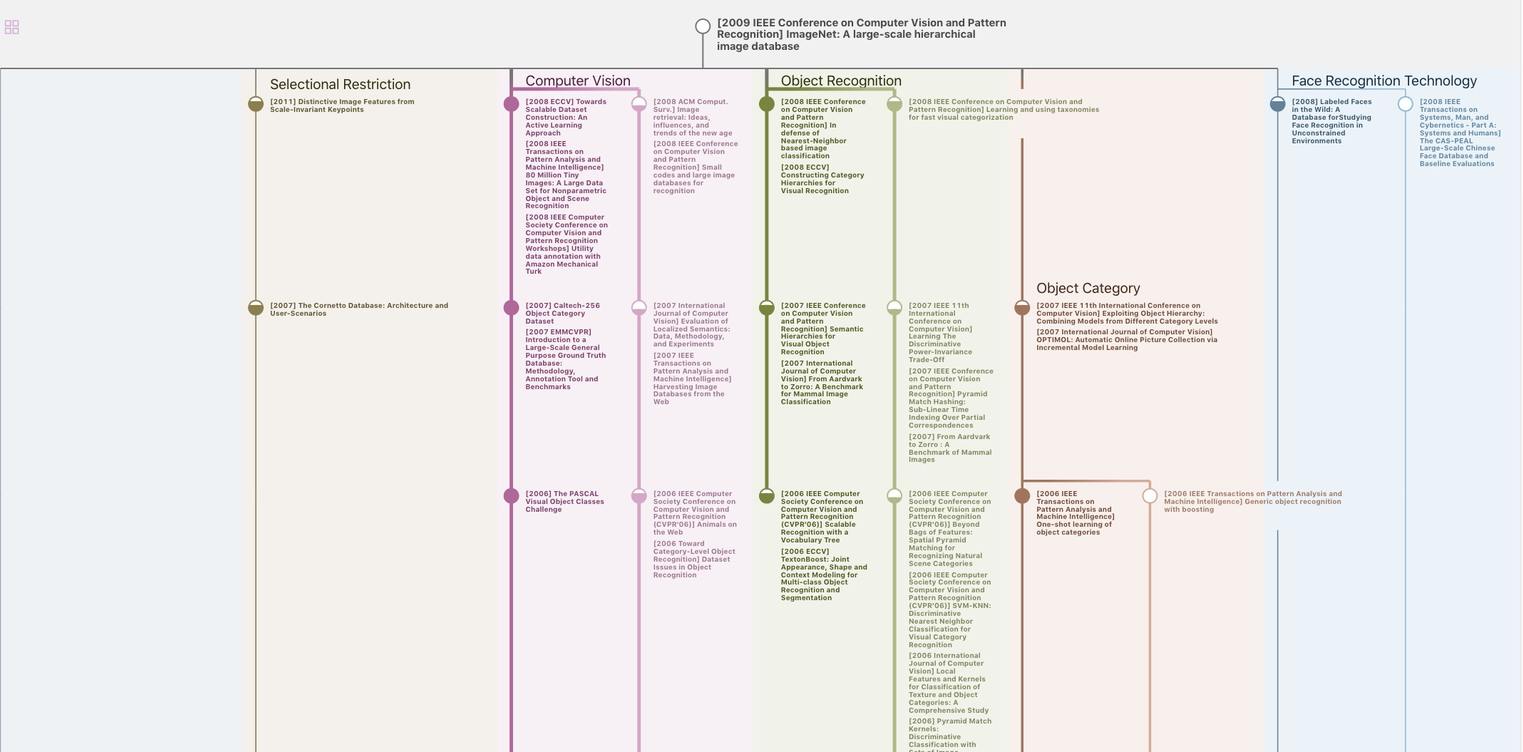
生成溯源树,研究论文发展脉络
Chat Paper
正在生成论文摘要