Workload time series prediction in storage systems: a deep learning based approach
Cluster Computing(2021)
摘要
Storage workload prediction is a critical step for fine-grained load balancing and job scheduling in realtime and adaptive cluster systems. However, how to perform workload time series prediction based on a deep learning method has not yet been thoroughly studied. In this paper, we propose a storage workload prediction method called CrystalLP based on deep learning. CrystalLP includes workload collecting, data preprocessing, time series prediction, and data post-processing phase. The time series prediction phase is based on a long short-term memory network (LSTM). Furthermore, to improve the efficiency of LSTM, we study the sensitivity of the hyperparameters in LSTM. Extensive experimental results show that CrystalLP can obtain performance improvement compared with three classic time series prediction algorithms.
更多查看译文
关键词
Workload prediction, LSTM, Time series
AI 理解论文
溯源树
样例
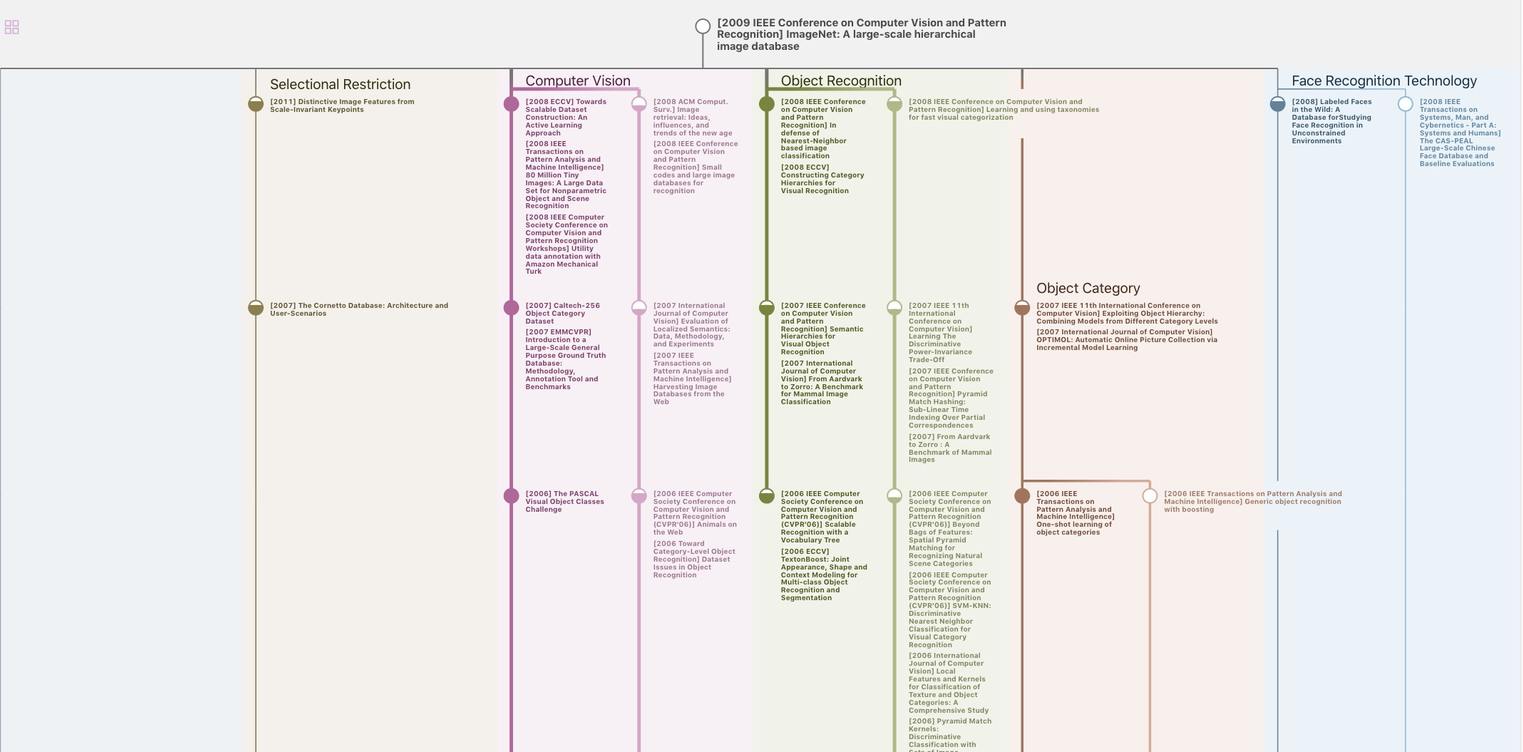
生成溯源树,研究论文发展脉络
Chat Paper
正在生成论文摘要