Kernel-based Identification of Asymptotically Stable Continuous-Time Linear Dynamical Systems
International journal of control(2021)
摘要
In many engineering applications, continuous-time models are preferred to discrete-time ones, in that they provide good physical insight and can be derived also from non-uniformly sampled data. However, for such models, model selection is a hard task if no prior physical knowledge is given. In this paper, we propose a non-parametric approach to infer a continuous-time linear model from data, by automatically selecting a proper structure of the transfer function and guaranteeing to preserve the system stability properties. By means of benchmark simulation examples, the proposed approach is shown to outperform state-of-the-art continuous-time methods, also in the critical case when short sequences of canonical input signals, like impulses or steps, are used for model learning.
更多查看译文
关键词
Kernel-based learning,linear identification,continuous-time identification
AI 理解论文
溯源树
样例
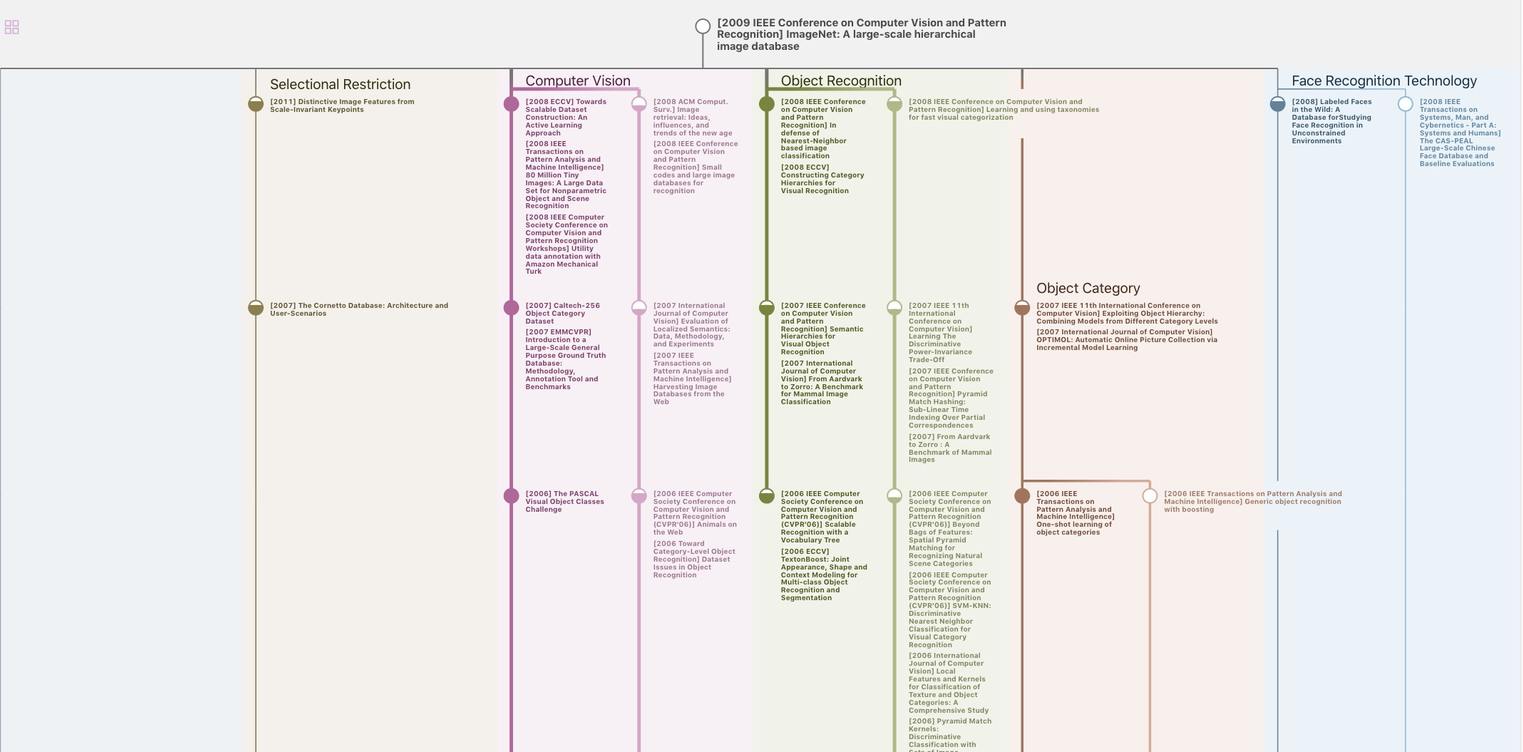
生成溯源树,研究论文发展脉络
Chat Paper
正在生成论文摘要