A Deep Machine Learning Approach for Lidar Based Boundary Layer Height Detection
IGARSS 2020 - 2020 IEEE INTERNATIONAL GEOSCIENCE AND REMOTE SENSING SYMPOSIUM(2020)
摘要
Inspired by the importance of Planetary Boundary Layer Heights (PBLH) for inferring Air Quality assessments and the disappointing performance of current weather forecasts of PBLH, this paper presents the proposed impact of using a Machine Learning derived PBLH (ML-PBLH) using ground-based Ceilometer observing systems. The PBLH is vital in air pollution studies to determine the extent of vertical mixing of pollutants (e.g., particles, trace gases, etc.). On the other hand, model forecasts of the nighttime collapse of the PBLH close to the surface, grossly underestimate the actual observations. We propose using machine learning methods, employing deep neural networks for denoising and image segmentation to detect PBLH from the Ceilometer backscatter signal from the LIDAR observations. The ML-PBLH detection algorithm shows promising early results when compared with conventional ground-based Ceilometer retrieval methods such as Wavelet methods used for LIDAR identification of PBLH under normal conditions. The use of conventional methods as well as the wavelet method for LIDAR backscatter retrievals is limited in the presence of dense clouds. However, the proposed machine learning approach is able to infer PBLH even under such conditions.
更多查看译文
关键词
machine learning methods,LIDAR observations,ML-PBLH detection algorithm,conventional ground-based,deep machine learning approach,lidar based boundary layer height detection,Planetary Boundary Layer Heights,Air Quality assessments,PBLH using ground-based,air pollution studies,ground-based Ceilometer observing systems,deep neural networks,image segmentation
AI 理解论文
溯源树
样例
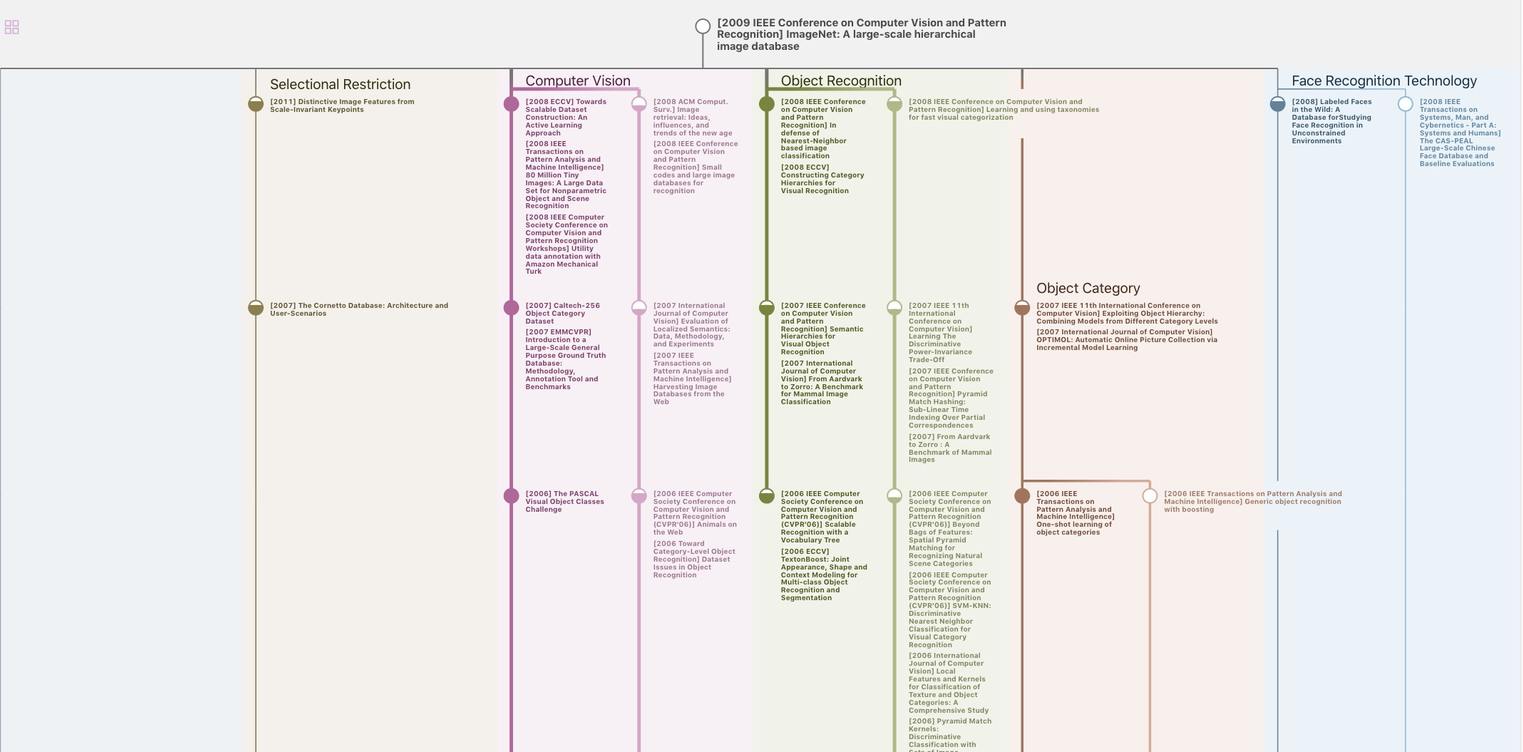
生成溯源树,研究论文发展脉络
Chat Paper
正在生成论文摘要