Misguided Use of Observed Covariates to Impute Missing Covariates in Conditional Prediction: A Shrinkage Problem
arxiv(2021)
摘要
Researchers regularly perform conditional prediction using imputed values of missing data. However, applications of imputation often lack a firm foundation in statistical theory. This paper originated when we were unable to find analysis substantiating claims that imputation of missing data has good frequentist properties when data are missing at random (MAR). We focused on the use of observed covariates to impute missing covariates when estimating conditional means of the form E(y|x, w). Here y is an outcome whose realizations are always observed, x is a covariate whose realizations are always observed, and w is a covariate whose realizations are sometimes unobserved. We examine the probability limit of simple imputation estimates of E(y|x, w) as sample size goes to infinity. We find that these estimates are not consistent when covariate data are MAR. To the contrary, the estimates suffer from a shrinkage problem. They converge to points intermediate between the conditional mean of interest, E(y|x, w), and the mean E(y|x) that conditions only on x. We use a type of genotype imputation to illustrate.
更多查看译文
关键词
missing covariates,conditional prediction,observed covariates
AI 理解论文
溯源树
样例
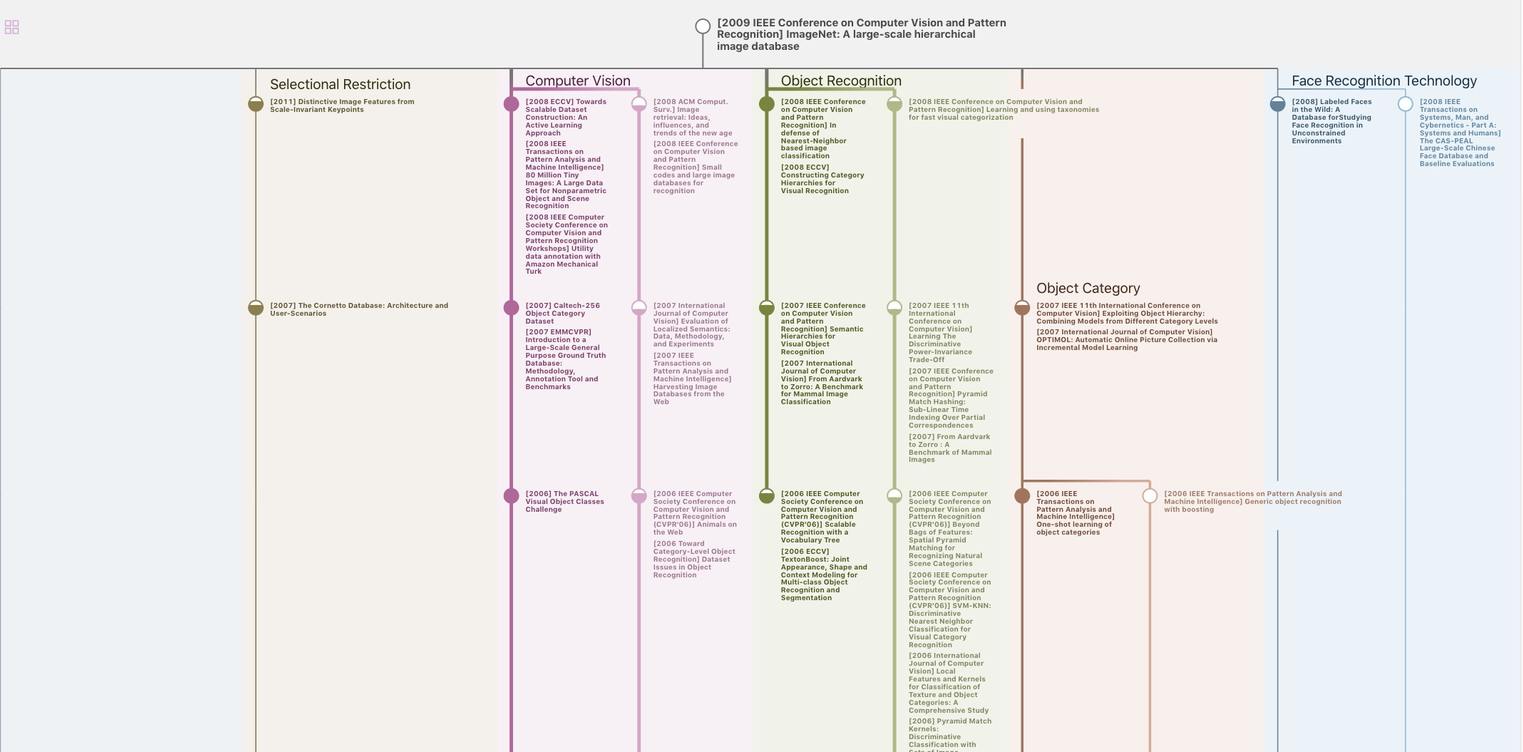
生成溯源树,研究论文发展脉络
Chat Paper
正在生成论文摘要