Medical Transformer: Gated Axial-Attention for Medical Image Segmentation
MEDICAL IMAGE COMPUTING AND COMPUTER ASSISTED INTERVENTION - MICCAI 2021, PT I(2021)
摘要
Over the past decade, deep convolutional neural networks have been widely adopted for medical image segmentation and shown to achieve adequate performance. However, due to inherent inductive biases present in convolutional architectures, they lack understanding of long-range dependencies in the image. Recently proposed transformer-based architectures that leverage self-attention mechanism encode long-range dependencies and learn representations that are highly expressive. This motivates us to explore transformer-based solutions and study the feasibility of using transformer-based network architectures for medical image segmentation tasks. Majority of existing transformer-based network architectures proposed for vision applications require large-scale datasets to train properly. However, compared to the datasets for vision applications, in medical imaging the number of data samples is relatively low, making it difficult to efficiently train transformers for medical imaging applications. To this end, we propose a gated axial-attention model which extends the existing architectures by introducing an additional control mechanism in the self-attention module. Furthermore, to train the model effectively on medical images, we propose a Local-Global training strategy (LoGo) which further improves the performance. Specifically, we operate on the whole image and patches to learn global and local features, respectively. The proposed Medical Transformer (MedT) is evaluated on three different medical image segmentation datasets and it is shown that it achieves better performance than the convolutional and other related transformer-based architectures. Code: https://github.com/jeya-maria-jose/Medical-Transformer
更多查看译文
关键词
Transformers, Medical image segmentation, Self-attention
AI 理解论文
溯源树
样例
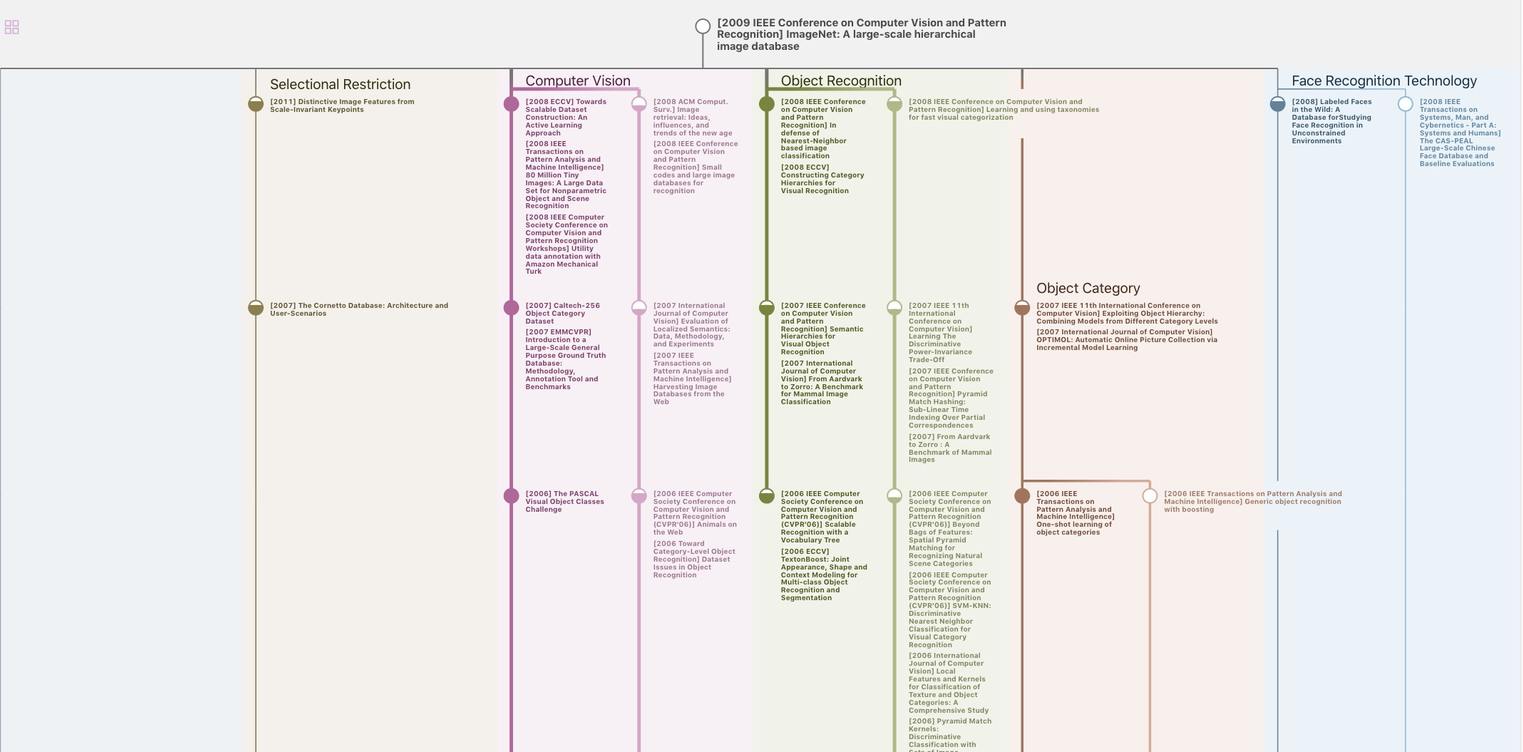
生成溯源树,研究论文发展脉络
Chat Paper
正在生成论文摘要