Logarithmic Regret in Feature-based Dynamic Pricing
Annual Conference on Neural Information Processing Systems(2021)
摘要
Feature-based dynamic pricing is an increasingly popular model of setting prices for highly differentiated products with applications in digital marketing, online sales, real estate and so on. The problem was formally studied as an online learning problem [Javanmard and Nazerzadeh, 2019] where a seller needs to propose prices on the fly for a sequence of T products based on their features x while having a small regret relative to the best -"omniscient"- pricing strategy she could have come up with in hindsight. We revisit this problem and provide two algorithms (EMLP and ONSP) for stochastic and adversarial feature settings, respectively, and prove the optimal O(d log T) regret bounds for both. In comparison, the best existing results are O (min {1/lambda(2)(min) log T, root T}) and O(T-2/3) respectively, with.min being the smallest eigenvalue of E[xx(T)] that could be arbitrarily close to 0. We also prove an Omega(root T) information-theoretic lower bound for a slightly more general setting, which demonstrates that "knowing-the-demand-curve" leads to an exponential improvement in feature-based dynamic pricing.
更多查看译文
关键词
feature-based
AI 理解论文
溯源树
样例
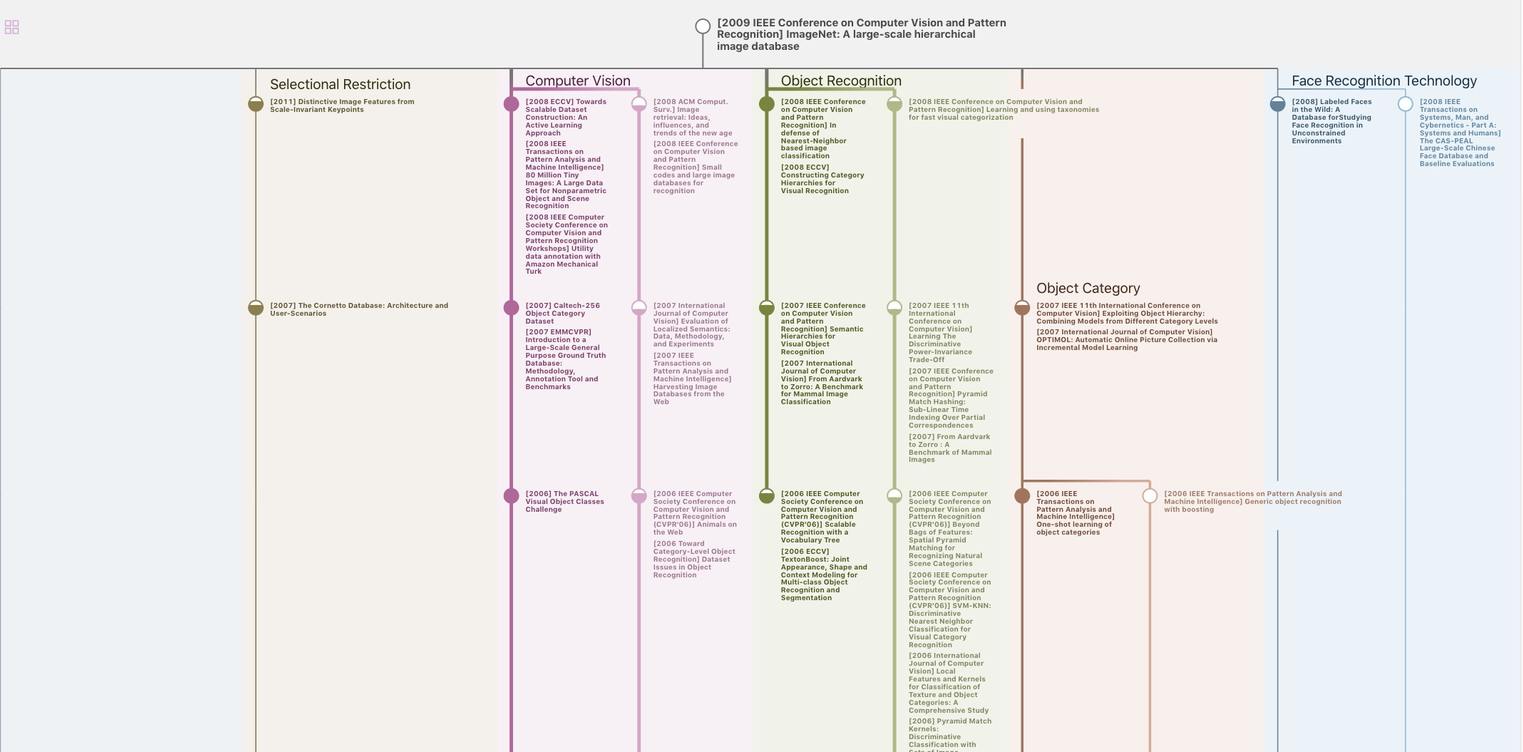
生成溯源树,研究论文发展脉络
Chat Paper
正在生成论文摘要